Probabilistic Modeling of Reaction Force/Torque through Fourier Transform and Entropy Analysis
2019 International Conference on Electronics, Information, and Communication (ICEIC)(2019)
摘要
In this paper, we propose a method to improve the recognition performance of a probabilistic model through entropy analysis after transforming time-varying reaction force/torque signals into frequency components. To perform tasks that require physical interaction, it is important for robots to recognize reaction force/torque during the interactions between robots and environments. However, the reaction force/torque measured by F/T sensor contains a lot of noise signals due to the sensitivity of the sensor. Therefore, the recognition performance depends on noise signals included in training and/or test dataset. To solve this problem, the reaction force/torque signals are transformed from time domain into frequency domain by fast Fourier transform. Then, some task-relevant frequency components are selected based on their entropy analysis, after which they are used to learn a hidden Markov model. To evaluate our proposed method, several robot manipulation tasks are performed using an open dataset including reaction force/torque signals: approaching, transferring, and positioning.
更多查看译文
关键词
Hidden Markov models,Task analysis,Entropy,Robots,Bars,Time-frequency analysis
AI 理解论文
溯源树
样例
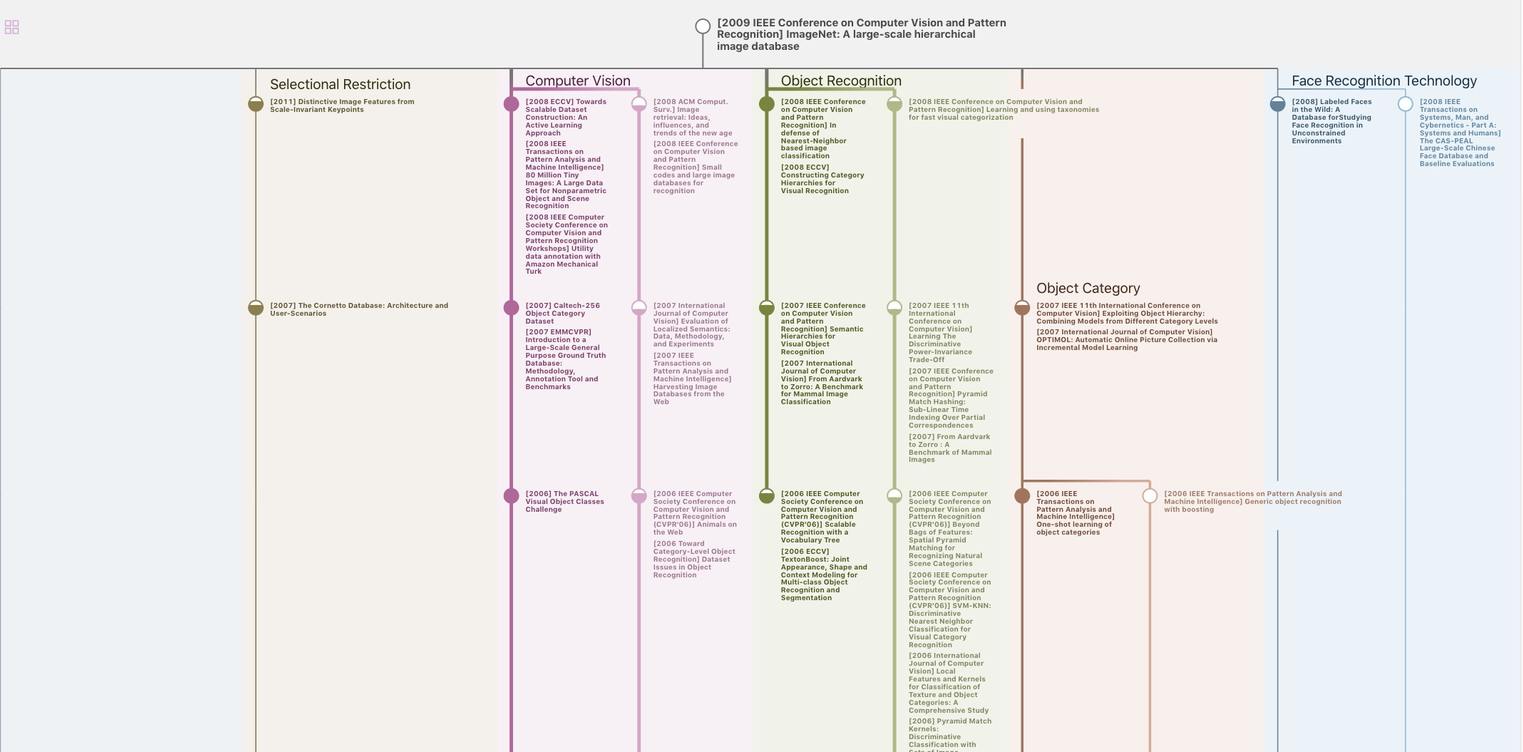
生成溯源树,研究论文发展脉络
Chat Paper
正在生成论文摘要