Why do Larger Models Generalize Better? A Theoretical Perspective via the XOR Problem
arXiv: Learning(2019)
摘要
Empirical evidence suggests that neural networks with ReLU activations generalize better with over-parameterization. However, there is currently no theoretical analysis that explains this observation. In this work, we provide theoretical and empirical evidence that, in certain cases, overparameterized convolutional networks generalize better than small networks because of an interplay between weight clustering and feature exploration at initialization. We demonstrate this theoretically for a 3-layer convolutional neural network with max-pooling, in a novel setting which extends the XOR problem. We show that this interplay implies that with overparamterization, gradient descent converges to global minima with better generalization performance compared to global minima of small networks. Empirically, we demonstrate these phenomena for a 3-layer convolutional neural network in the MNIST task.
更多查看译文
关键词
larger models generalize,xor problem,theoretical perspective
AI 理解论文
溯源树
样例
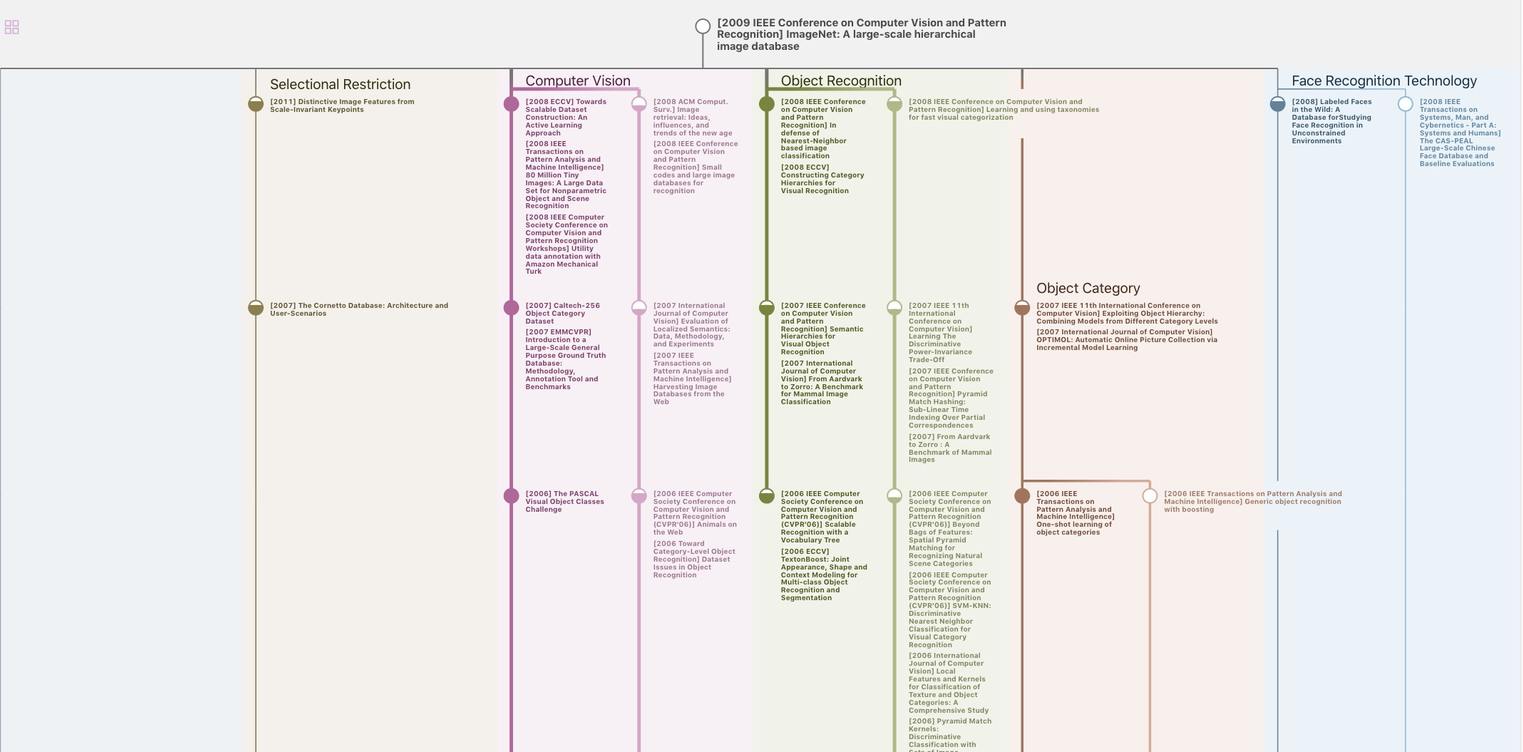
生成溯源树,研究论文发展脉络
Chat Paper
正在生成论文摘要