Sampling From Non-Log-Concave Distributions Via Stochastic Variance-Reduced Gradient Langevin Dynamics
international conference on artificial intelligence and statistics(2019)
摘要
We study stochastic variance reduction-based Langevin dynamic algorithms, SVRG-LD and SAGA-LD (Dubey et al., 2016), for sampling from non-log-concave distributions. Under certain assumptions on the log density function, we establish the convergence guarantees of SVRG-LD and SAGA-LD in 2-Wasserstein distance. More specifically, we show that both SVRG-LD and SAGA-LD require (O) over tilde (n+n(3/4)/epsilon(2)+n(1/2)/epsilon(4)).exp ((O) over tilde (d+ gamma)) stochastic gradient evaluations to achieve epsilon-accuracy in 2-Wasserstein distance, which outperforms the (O) over tilde (n/epsilon(4)).exp ((O) over tilde (d + gamma)) gradient complexity achieved by Langevin Monte Carlo Method (Raginsky et al., 2017). Experiments on synthetic data and real data back up our theory.
更多查看译文
AI 理解论文
溯源树
样例
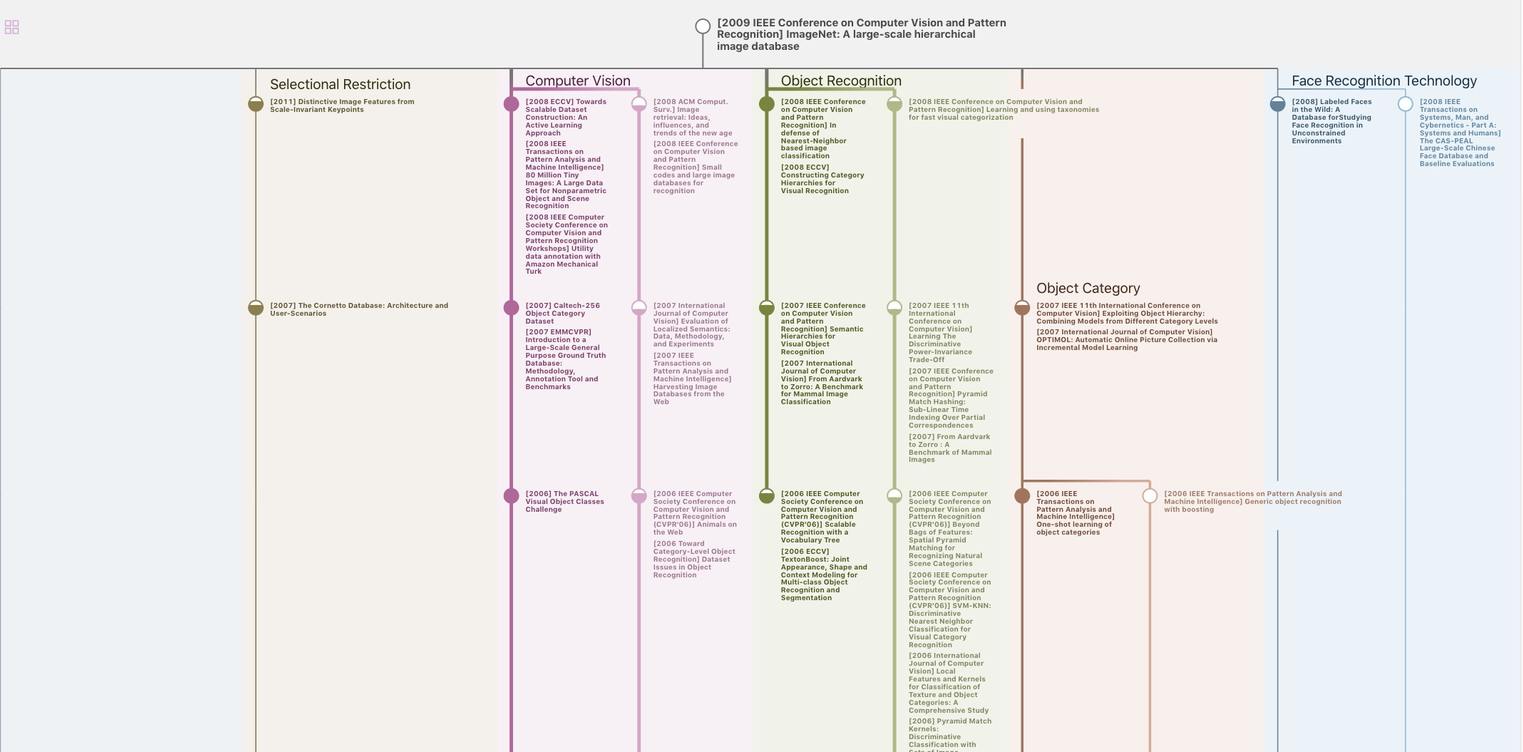
生成溯源树,研究论文发展脉络
Chat Paper
正在生成论文摘要