Transfer Learning Using Raw Waveform Sincnet For Robust Speaker Diarization
2019 IEEE INTERNATIONAL CONFERENCE ON ACOUSTICS, SPEECH AND SIGNAL PROCESSING (ICASSP)(2019)
摘要
Speaker diarization tells who spoke and when? in an audio stream. SincNet is a recently developed novel convolutional neural network (CNN) architecture where the first layer consists of parameterized sinc filters. Unlike conventional CNNs, SincNet take raw speech waveform as input. This paper leverages SincNet in vanilla transfer learning (VTL) setup. Out-domain data is used for training SincNet-VTL to perform frame-level speaker classification. Trained SincNet-VTL is later utilized as feature extractor for in-domain data. We investigated pooling (max, avg) strategies for deriving utterance-level embedding using frame-level features extracted from trained network. These utterance/segment level embedding are adopted as speaker models during clustering stage in diarization pipeline. We compared the proposed SincNet-VTL embedding with baseline i-vector features. We evaluated our approaches on two corpora, CRSS-PLTL and AMI. Results show the efficacy of trained SincNet-VTL for speaker-discriminative embedding even when trained on small amount of data. Proposed features achieved relative DER improvements of 19.12% and 52.07% for CRSS-PLTL and AMI data, respectively over baseline i-vectors.
更多查看译文
关键词
Speaker Clustering, SincNet, Audio Diarization, Peer-led team learning, Transfer Learning
AI 理解论文
溯源树
样例
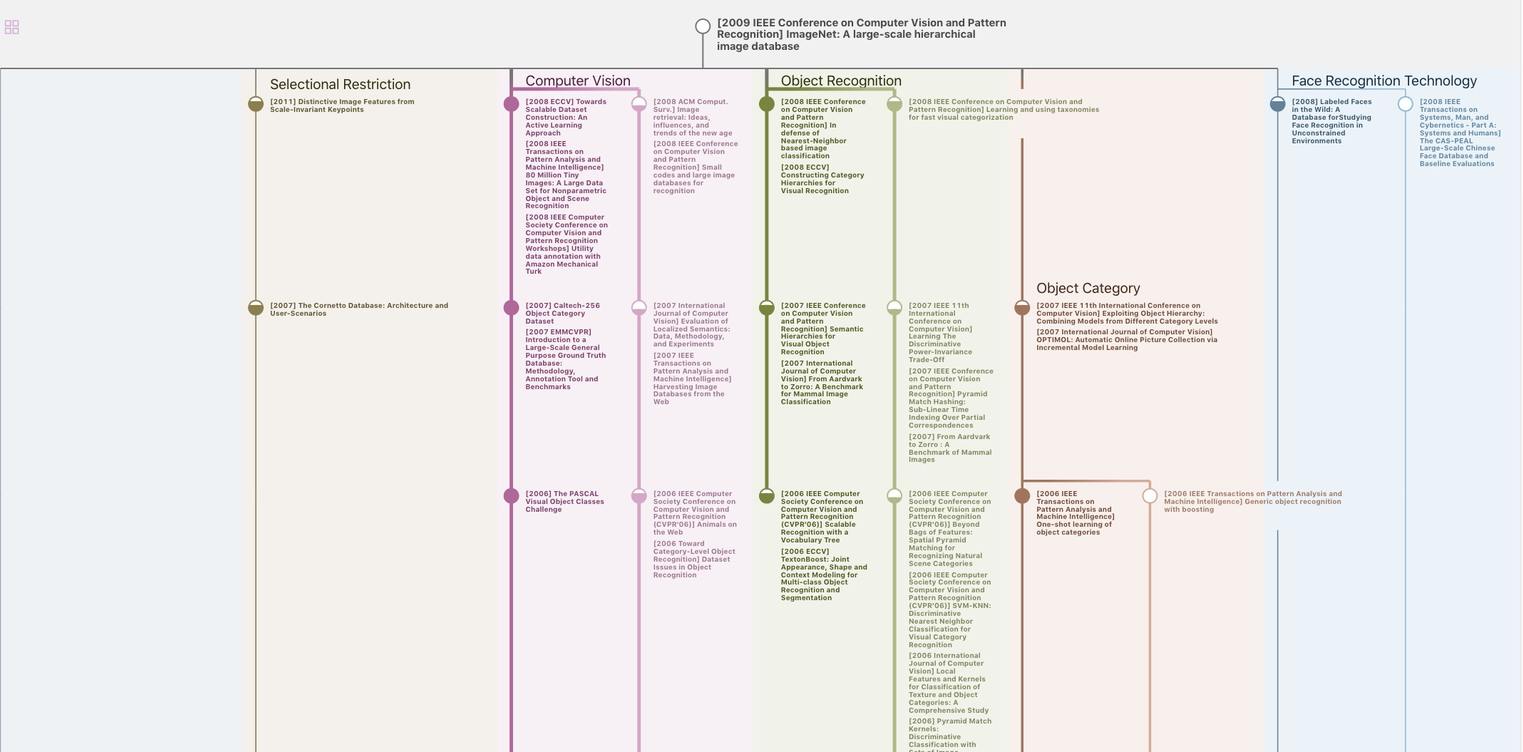
生成溯源树,研究论文发展脉络
Chat Paper
正在生成论文摘要