Supervised Saliency Mapping for First-Person Videos With an Inverse Sparse Coding Framework.
IEEE ACCESS(2019)
摘要
Saliency mapping is an efficient means of processing large amounts of incoming visual information from images and videos. Existing methods based on feature integration and construction error provide a rough saliency mapping and are sensitive to real-world noise. Furthermore, for first-person-vision video related to human activities, existing methods identify objects in the image without considering the human actions being performed. To address this issue, we propose a novel supervised saliency mapping based on a sparse coding framework. Different from the normal sparse representation, which uses dictionary atoms to represent signals, we use the inverse expression, whereby the use original image or video frame signals provide the normal dictionary, and these signals are used to represent the salience superpixel matrix, which is learned from a class of training images. We then construct the salient map by inverse sparse coding. In this paper, we first describe how the salience superpixel matrix is extracted by supervised selection, and then explain how to obtain the salient map through an inverse sparse coding framework. The experimental results show the enhanced accuracy of the proposed method compared with previous methods, as well as the time efficiency of the proposed method.
更多查看译文
关键词
inverse sparse coding,orthogonal matching pursuit (OMP),salience superpixel matrix,saliency mapping,supervised
AI 理解论文
溯源树
样例
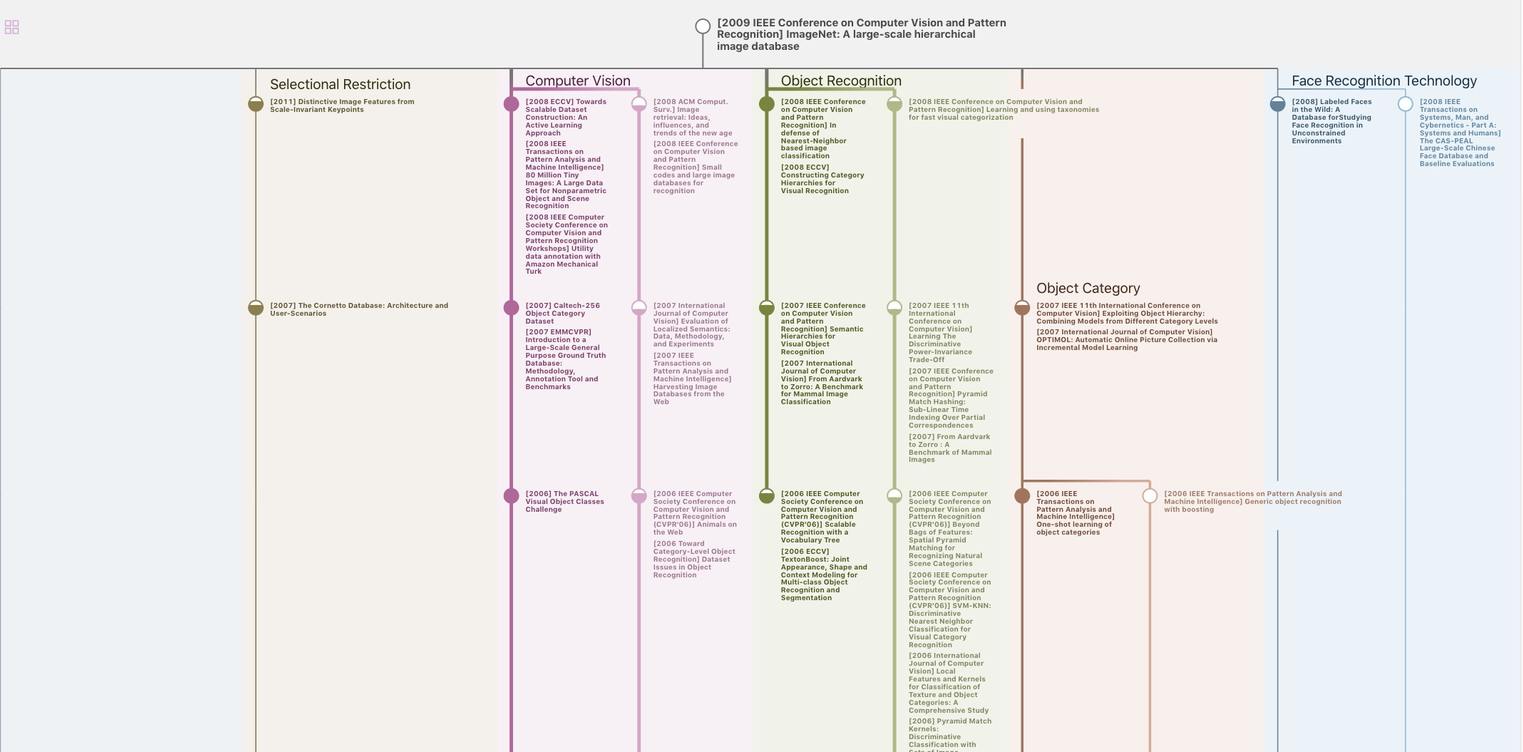
生成溯源树,研究论文发展脉络
Chat Paper
正在生成论文摘要