Adversarial Neural Network Inversion via Auxiliary Knowledge Alignment.
arXiv: Cryptography and Security(2019)
摘要
The rise of deep learning technique has raised new privacy concerns about the training data and test data. In this work, we investigate the model inversion problem in the adversarial settings, where the adversary aims at inferring information about the target modelu0027s training data and test data from the modelu0027s prediction values. We develop a solution to train a second neural network that acts as the inverse of the target model to perform the inversion. The inversion model can be trained with black-box accesses to the target model. We propose two main techniques towards training the inversion model in the adversarial settings. First, we leverage the adversaryu0027s background knowledge to compose an auxiliary set to train the inversion model, which does not require access to the original training data. Second, we design a truncation-based technique to align the inversion model to enable effective inversion of the target model from partial predictions that the adversary obtains on victim useru0027s data. We systematically evaluate our inversion approach in various machine learning tasks and model architectures on multiple image datasets. Our experimental results show that even with no full knowledge about the target modelu0027s training data, and with only partial prediction values, our inversion approach is still able to perform accurate inversion of the target model, and outperform previous approaches.
更多查看译文
关键词
auxiliary knowledge alignment,neural network
AI 理解论文
溯源树
样例
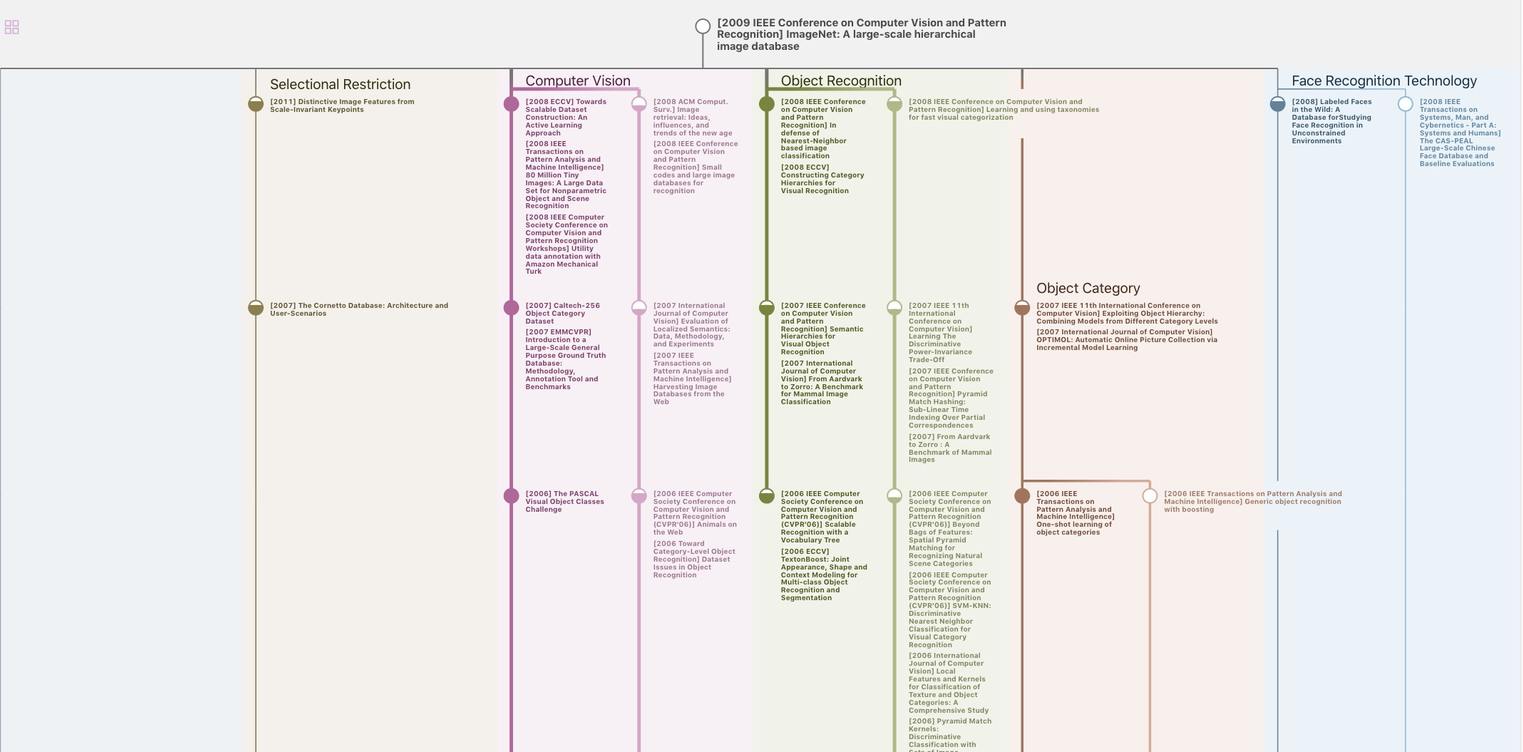
生成溯源树,研究论文发展脉络
Chat Paper
正在生成论文摘要