Utterance-level Aggregation for Speaker Recognition in the Wild
2019 IEEE INTERNATIONAL CONFERENCE ON ACOUSTICS, SPEECH AND SIGNAL PROCESSING (ICASSP)(2019)
摘要
The objective of this paper is speaker recognition `in the wild' - where utterances may be of variable length and also contain irrelevant signals. Crucial elements in the design of deep networks for this task are the type of trunk (frame level) network, and the method of temporal aggregation. We propose a powerful speaker recognition deep network, using a `thin-ResNet' trunk architecture, and a dictionary-based NetVLAD or GhostVLAD layer to aggregate features across time, that can be trained end-to-end. We show that our network achieves state of the art performance by a significant margin on the VoxCeleb1 test set for speaker recognition, whilst requiring fewer parameters than previous methods. We also investigate the effect of utterance length on performance, and conclude that for `in the wild' data, a longer length is beneficial.
更多查看译文
关键词
speaker recognition, speaker verification, speech, deep learning, CNNs
AI 理解论文
溯源树
样例
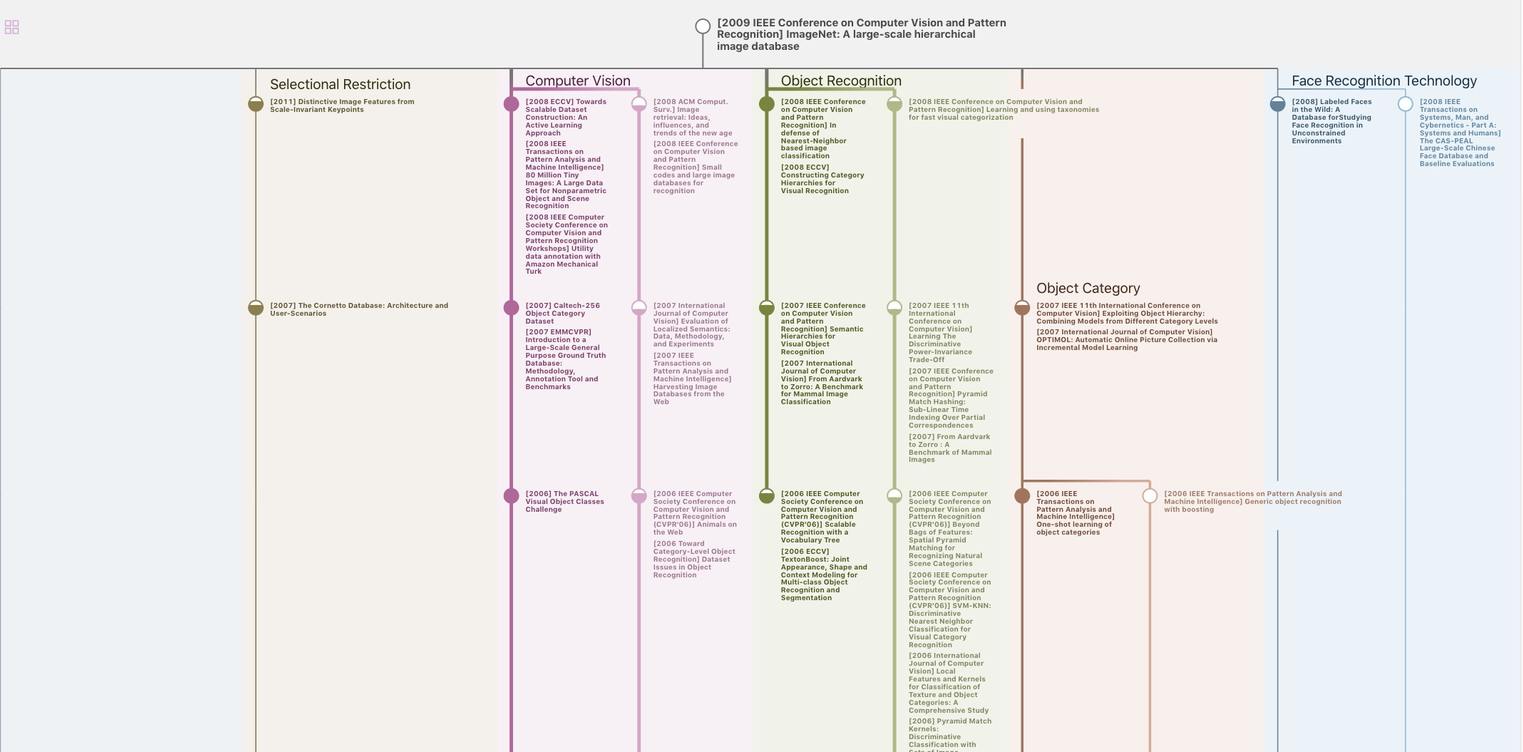
生成溯源树,研究论文发展脉络
Chat Paper
正在生成论文摘要