Multi-task Learning in Deep Gaussian Processes with Multi-kernel Layers
arXiv: Machine Learning(2019)
摘要
We present a multi-task learning formulation for Deep Gaussian processes (DGPs), describing a multi-kernel architecture for DGP layers. The proposed model is a non-linear mixture of latent Gaussian processes (GPs) with components shared between the tasks, in addition to separate task-specific components. Our formulation allows for learning complex relationships between tasks. We benchmark our model on three real-world datasets showing empirically that our formulation is able to improve the learning performance and transfer information between the tasks, outperforming state-of-the-art GP-based single-task learning and multi-task learning models.
更多查看译文
AI 理解论文
溯源树
样例
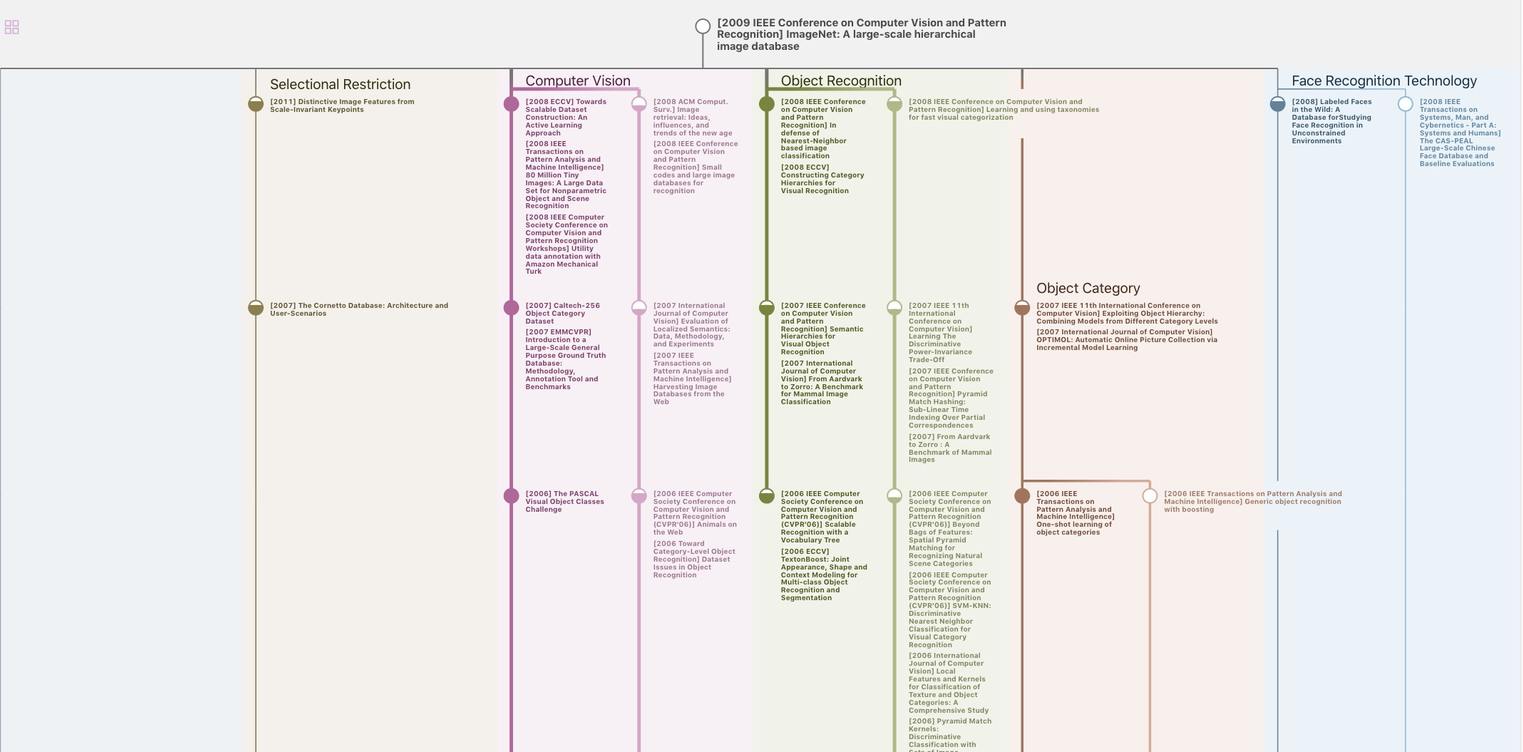
生成溯源树,研究论文发展脉络
Chat Paper
正在生成论文摘要