Generating Contrastive Explanations with Monotonic Attribute Functions.
arXiv: Learning(2019)
摘要
Explaining decisions of deep neural networks is a hot research topic with applications in medical imaging, video surveillance, and self driving cars. Many methods have been proposed in literature to explain these decisions by identifying relevance of different pixels. In this paper, we propose a method that can generate contrastive explanations for such data where we not only highlight aspects that are in themselves sufficient to justify the classification by the deep model, but also new aspects which if added will change the classification. One of our key contributions is how we define "addition" for such rich data in a formal yet humanly interpretable way that leads to meaningful results. This was one of the open questions laid out in Dhurandhar et.al. (2018) [5], which proposed a general framework for creating (local) contrastive explanations for deep models. We showcase the efficacy of our approach on CelebA and Fashion-MNIST in creating intuitive explanations that are also quantitatively superior compared with other state-of-the-art interpretability methods.
更多查看译文
AI 理解论文
溯源树
样例
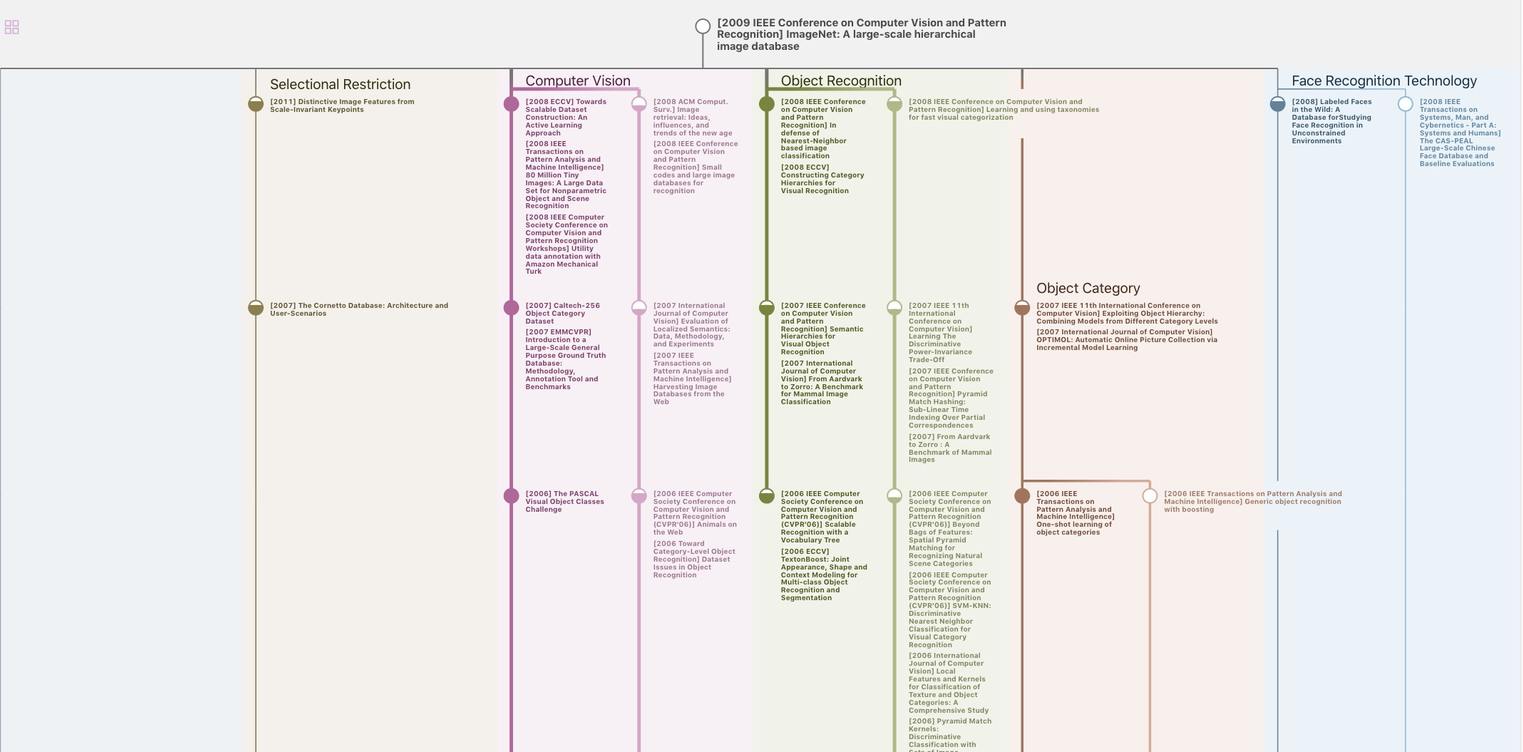
生成溯源树,研究论文发展脉络
Chat Paper
正在生成论文摘要