Disentangled Graph Convolutional Networks
international conference on machine learning(2019)
摘要
The formation of a real-world graph typically arises from the highly complex interaction of many latent factors. The existing deep learning methods for graph-structured data neglect the entanglement of the latent factors, rendering the learned representations non-robust and hardly explainable. However, learning representations that disentangle the latent factors poses great challenges and remains largely unexplored in the literature of graph neural networks. In this paper, we introduce the disentangled graph convolutional network (DisenGCN) to learn disentangled node representations. In particular, we propose a novel neighborhood routing mechanism, which is capable of dynamically identifying the latent factor that may have caused the edge between a node and one of its neighbors, and accordingly assigning the neighbor to a channel that extracts and convolutes features specific to that factor. We theoretically prove the convergence properties of the routing mechanism. Empirical results show that our proposed model can achieve significant performance gains, especially when the data demonstrate the existence of many entangled factors.
更多查看译文
AI 理解论文
溯源树
样例
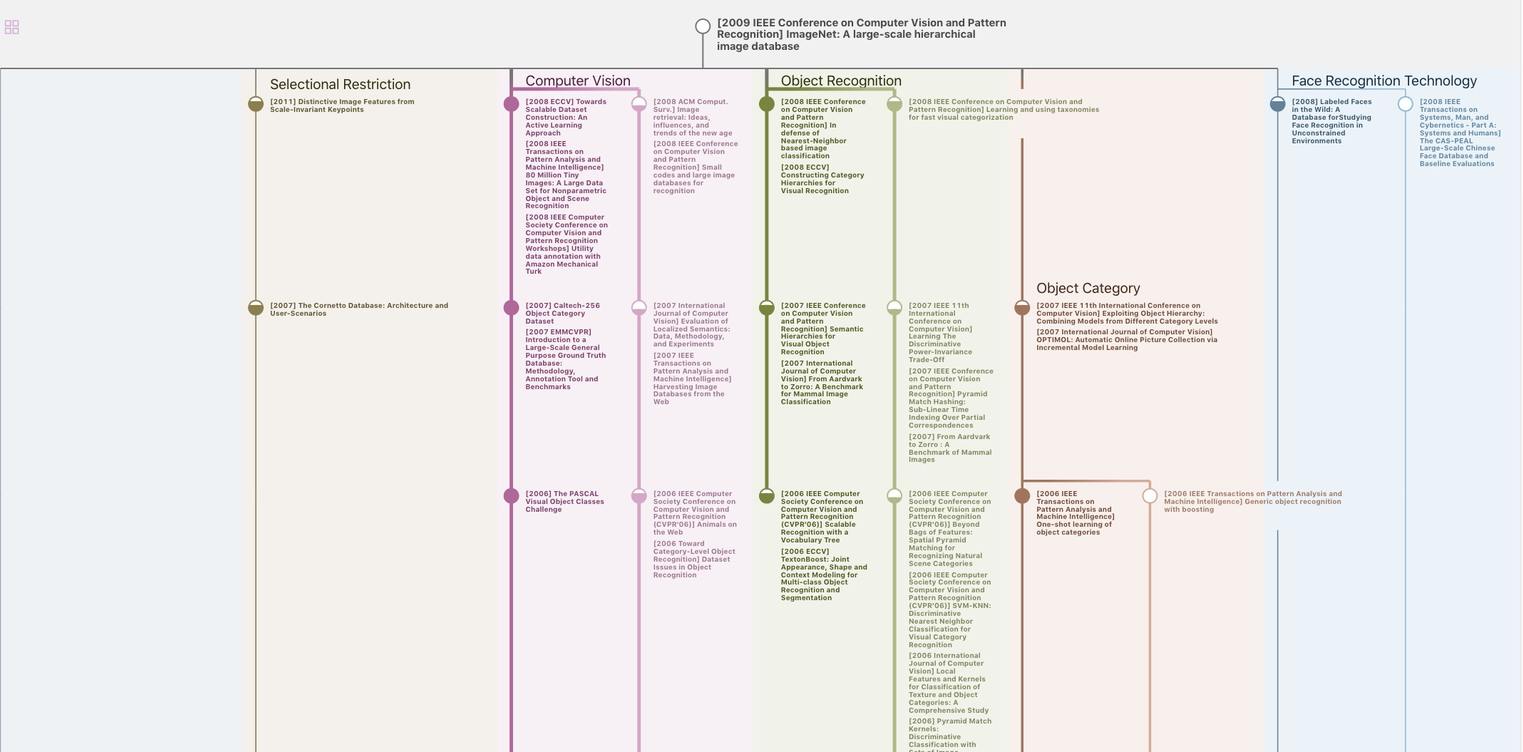
生成溯源树,研究论文发展脉络
Chat Paper
正在生成论文摘要