A 16-nm Always-On DNN Processor With Adaptive Clocking and Multi-Cycle Banked SRAMs
IEEE Journal of Solid-state Circuits(2019)
摘要
Always-on subsystems in mobile/Internet of Things (IoT) SoCs process a variety of real-time sensor data deep neural network (DNN) classification workloads in a heavily constrained energy budget. This can be achieved with robust, low-voltage circuits, and specialized hardware accelerators. We present a 16-nm always-on DNN processor, which consists primarily of a microcontroller and a DNN accelerator with on-chip SRAM for the model weights. The design operates robustly from 0.4 to 1-V, with calibration-free automatic voltage/frequency tuning provided by tracking small non-zero razor timing error rates. A novel timing error-driven synchronization-free adaptive clocking scheme significantly reduces the adaptation latency to provide resilience to fast on-chip supply noise and reduce margins. To accommodate the tight energy constraints of always-on IoT workloads, we implement a multi-cycle SRAM read scheme that allows the memory voltage to scale at iso-throughput, improving energy efficiency across the entire operating range. The wide operating range allows for high performance at 1.36 GHz, low-power consumption downs to 750
$\mu \text{W}$
, and state-of-the-art raw efficiency at 16-bit precision of 750 GOPS/W dense or 1.81 TOPS/W sparse.
更多查看译文
关键词
Random access memory,Timing,Hardware,Clocks,Pipelines,Latches,System-on-chip
AI 理解论文
溯源树
样例
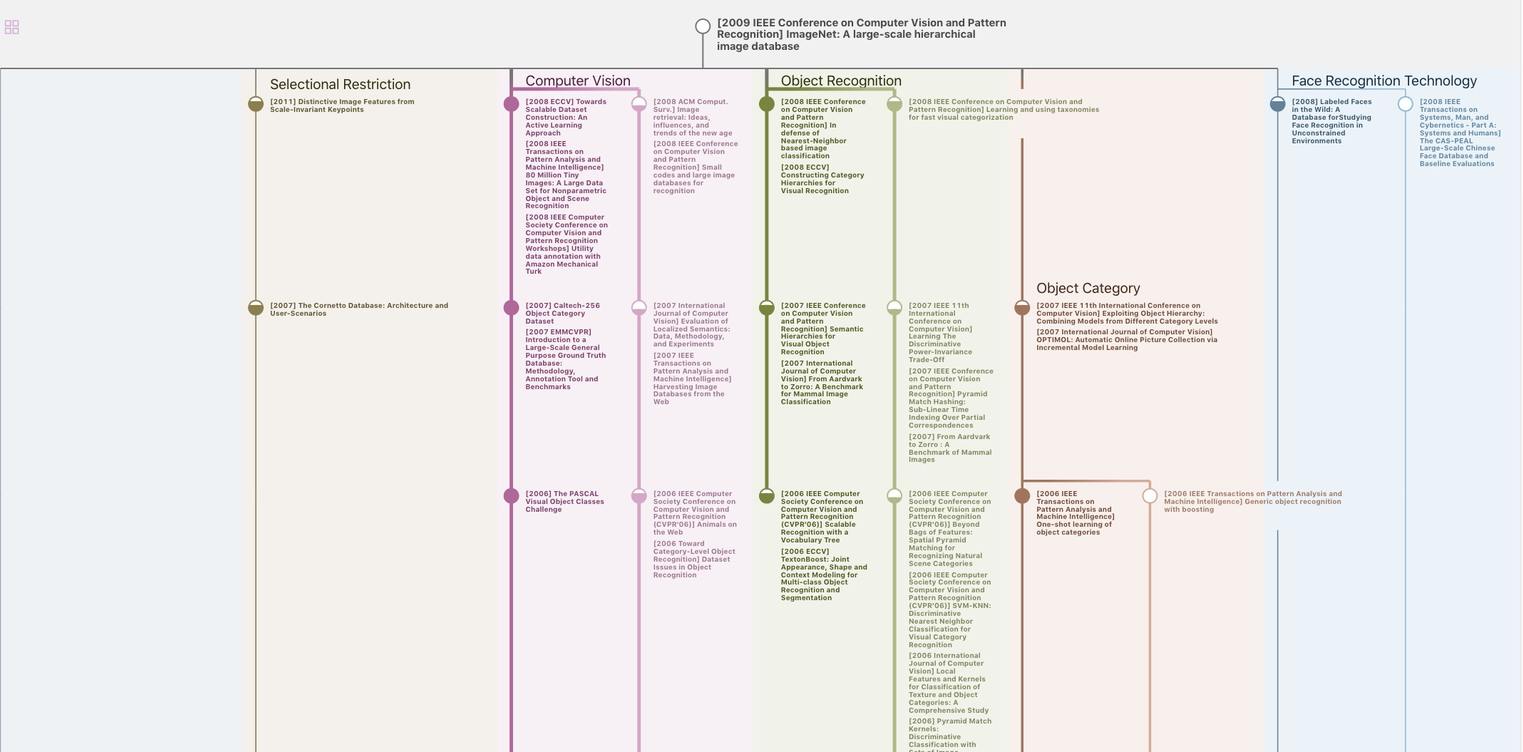
生成溯源树,研究论文发展脉络
Chat Paper
正在生成论文摘要