Brain Tumor Detection And Classification From Multi-Sequence Mri: Study Using Convnets
BRAINLESION: GLIOMA, MULTIPLE SCLEROSIS, STROKE AND TRAUMATIC BRAIN INJURIES, BRAINLES 2018, PT I(2018)
摘要
In this paper, we thoroughly investigate the power of Deep Convolutional Neural Networks (ConvNets) for classification of brain tumors using multi-sequence MR images. First we propose three ConvNets, which are trained from scratch, on MRI patches, slices, and multiplanar volumetric slices. The suitability of transfer learning for the task is next studied by applying two existing ConvNets models (VGGNet and ResNet) pre-trained on ImageNet dataset, through fine-tuning of the last few layers. Leave-one-patient-out (LOPO) testing scheme is used to evaluate the performance of the ConvNets. Results demonstrate that ConvNet achieves better accuracy in all cases where the model is trained on the multi-planar volumetric dataset. Unlike conventional models, it obtains a testing accuracy of 97% without any additional effort towards extraction and selection of features. We also study the properties of self-learned kernels/filters in different layers, through visualization of the intermediate layer outputs.
更多查看译文
关键词
Convolutional neural network, Deep learning, Brain tumor, Glioblastoma multiforme, Multi-sequence MRI, Transfer learning
AI 理解论文
溯源树
样例
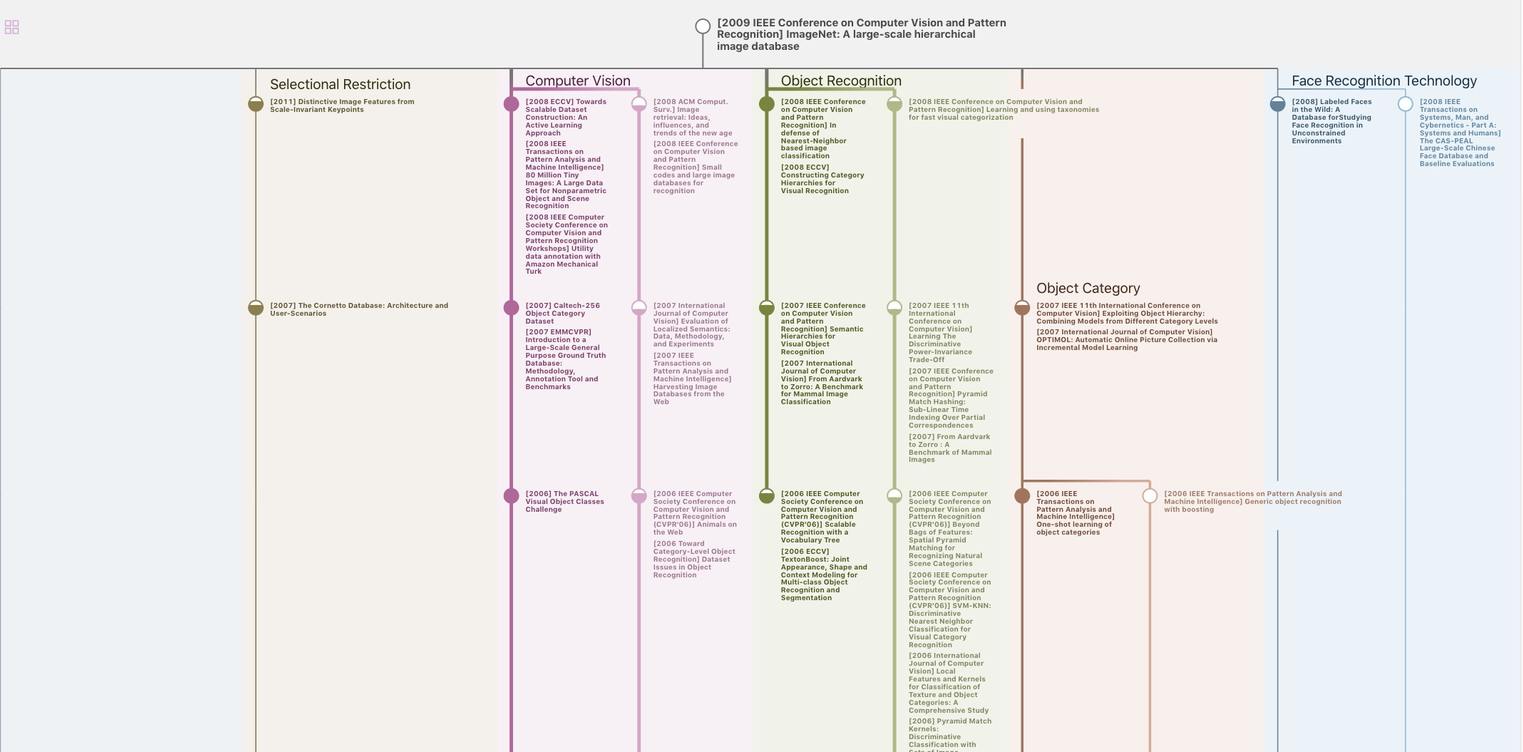
生成溯源树,研究论文发展脉络
Chat Paper
正在生成论文摘要