Deep inverse rendering for high-resolution SVBRDF estimation from an arbitrary number of images
ACM Transactions on Graphics (TOG)(2019)
摘要
In this paper we present a unified deep inverse rendering framework for estimating the spatially-varying appearance properties of a planar exemplar from an arbitrary number of input photographs, ranging from just a single photograph to many photographs. The precision of the estimated appearance scales from plausible when the input photographs fails to capture all the reflectance information, to accurate for large input sets. A key distinguishing feature of our framework is that it directly optimizes for the appearance parameters in a latent embedded space of spatially-varying appearance, such that no handcrafted heuristics are needed to regularize the optimization. This latent embedding is learned through a fully convolutional auto-encoder that has been designed to regularize the optimization. Our framework not only supports an arbitrary number of input photographs, but also at high resolution. We demonstrate and evaluate our deep inverse rendering solution on a wide variety of publicly available datasets.
更多查看译文
关键词
SVBRDF, auto-encoder, deep learning, material capture
AI 理解论文
溯源树
样例
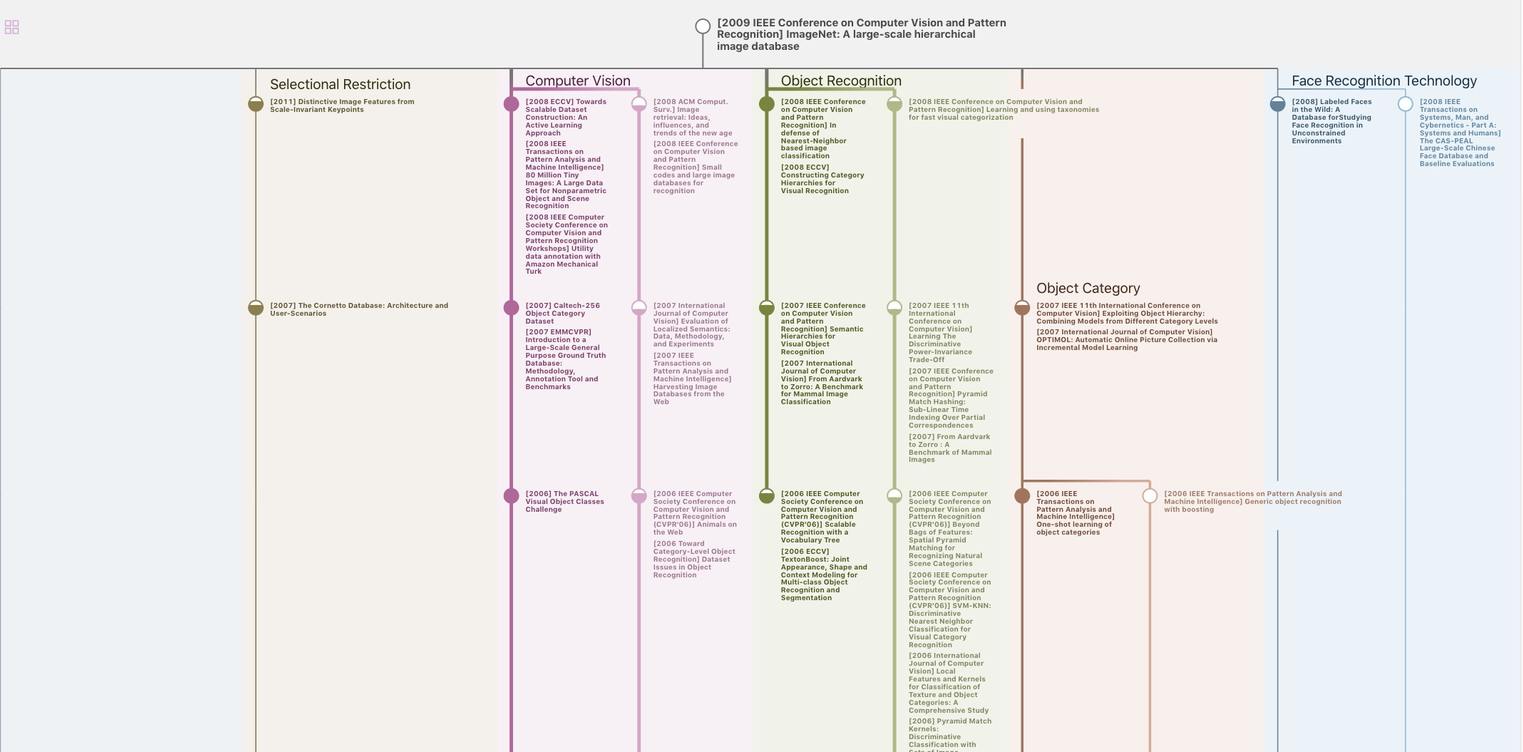
生成溯源树,研究论文发展脉络
Chat Paper
正在生成论文摘要