Robust Variational Autoencoders For Outlier Detection And Repair Of Mixed-Type Data
arxiv(2020)
摘要
We focus on the problem of unsupervised cell outlier detection and repair in mixed-type tabular data. Traditional methods are concerned only with detecting which rows in the dataset are outliers. However, identifying which cells are corrupted in a specific row is an important problem in practice, and the very first step towards repairing them. We introduce the Robust Variational Autoencoder (RVAE), a deep generative model that learns the joint distribution of the clean data while identifying the outlier cells, allowing their imputation (repair). RVAE explicitly learns the probability of each cell being an outlier, balancing different likelihood models in the row outlier score, making the method suitable for outlier detection in mixed-type datasets. We show experimentally that not only RVAE performs better than several state-of-the-art methods in cell outlier detection and repair for tabular data, but also that is robust against the initial hyper-parameter selection.
更多查看译文
关键词
outlier detection,mixed-type
AI 理解论文
溯源树
样例
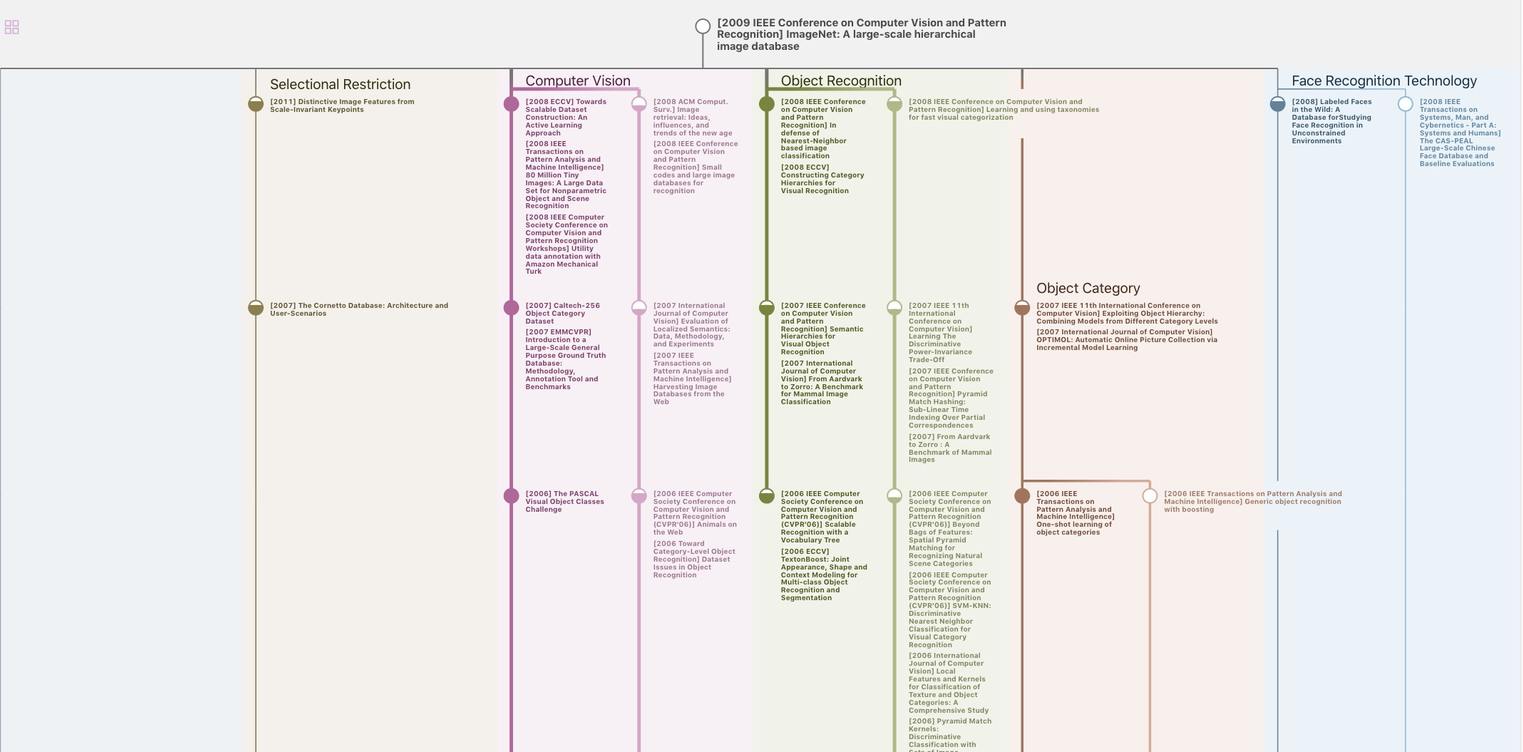
生成溯源树,研究论文发展脉络
Chat Paper
正在生成论文摘要