Semantic Mapping For View-Invariant Relocalization
2019 INTERNATIONAL CONFERENCE ON ROBOTICS AND AUTOMATION (ICRA)(2019)
摘要
We propose a system for visual simultaneous localization and mapping (SLAM) that combines traditional local appearance-based features with semantically meaningful object landmarks to achieve both accurate local tracking and highly view-invariant object-driven relocalization. Our mapping process uses a sampling-based approach to efficiently infer the 3D pose of object landmarks from 2D bounding box object detections. These 3D landmarks then serve as a view-invariant representation which we leverage to achieve camera relocalization even when the viewing angle changes by more than 125 degrees. This level of view-invariance cannot be attained by local appearance-based features (e.g. SIFT) since the same set of surfaces are not even visible when the viewpoint changes significantly. Our experiments show that even when existing methods fail completely for viewpoint changes of more than 70 degrees, our method continues to achieve a relocalization rate of around 90%, with a mean rotational error of around 8 degrees.
更多查看译文
关键词
semantic mapping,view-invariant relocalization,accurate local tracking,view-invariant object-driven relocalization,sampling-based approach,2D bounding box object detections,view-invariant representation,camera relocalization,view-invariance,relocalization rate,visual simultaneous localization and mapping,object landmarks,local appearance-based features,SLAM,3D pose,SIFT,mean rotational error
AI 理解论文
溯源树
样例
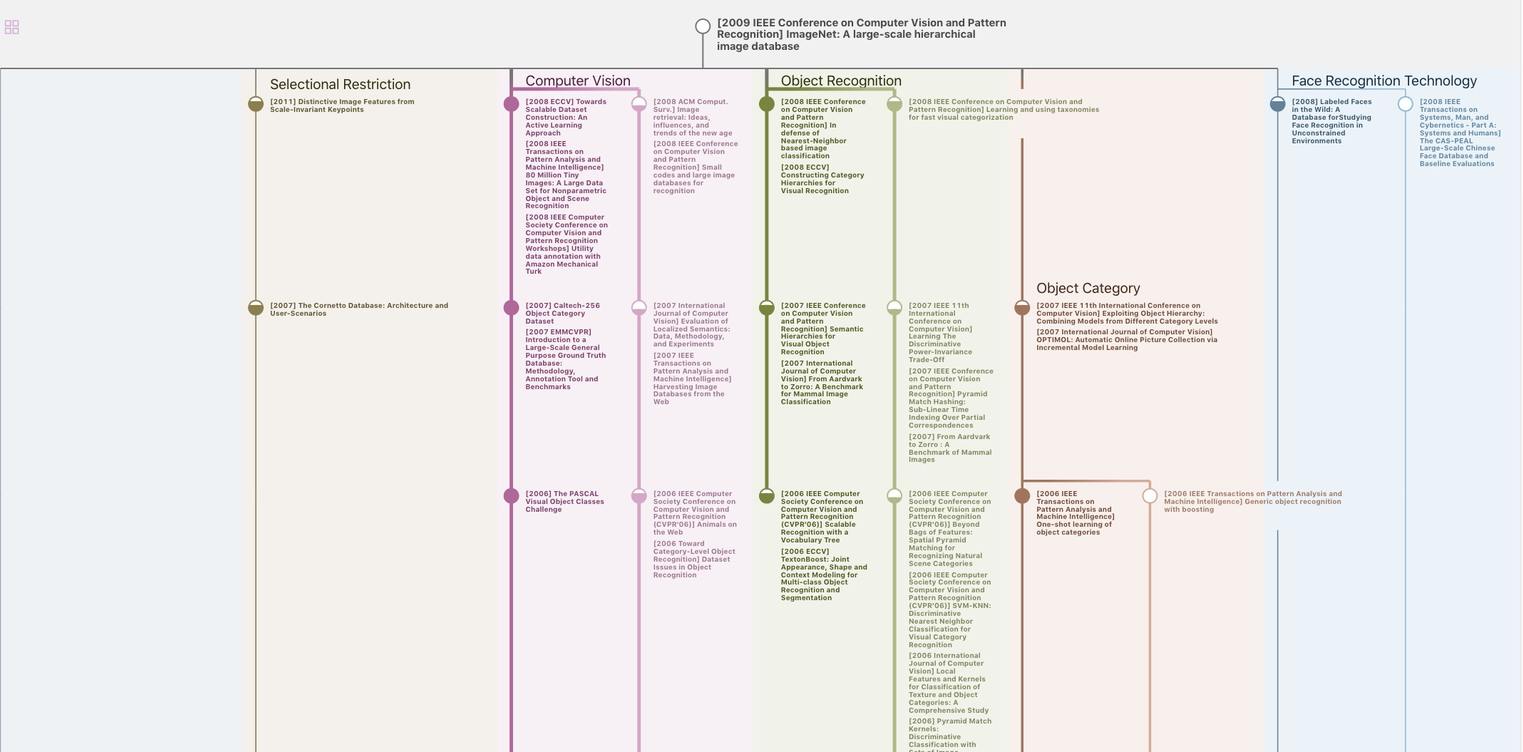
生成溯源树,研究论文发展脉络
Chat Paper
正在生成论文摘要