Causation-Driven Visualizations for Insurance Recommendation
2019 IEEE International Conference on Multimedia & Expo Workshops (ICMEW)(2019)
摘要
Recommender systems provide insurance enterprises with insights on customer behaviors and demands, thereby serving as an important source of revenue generation. Key success factors for a recommender include incorporation of multi-dimensional data from diverse media, clear and interpretable presentation of analytical results, and accurate recommendation of insurance products. However, most existing methods in the industry produce merely a list of recommendations without effectively visualizing them, and meanwhile are based on correlation-driven algorithms that can omit significant causal patterns in both customer and product data. In this paper, we propose a causation-driven visualization system that fundamentally transforms cross-media insurance data into network diagrams and performs recommendation reasoning. The system leverages data retrieved from both local insurance service providers and resources across the Web, and utilizes a multi-valued confounder balancing algorithm to compute the causal effect between customer and products, aiming to explore the reasons behind customers' purchasing behaviors and make appropriate recommendations accordingly. Furthermore, we conduct domain expert studies to evaluate the effectiveness of knowledge discovery by our causation-driven visualization system and correlation-driven tools currently adopted in the insurance industry. Results show that our system considerably outperforms others in terms of all evaluation metrics, and can potentially help insurers devise practical decision-making strategies.
更多查看译文
关键词
causation-driven,recommendation reasoning,confounder balancing,insurance recommendation
AI 理解论文
溯源树
样例
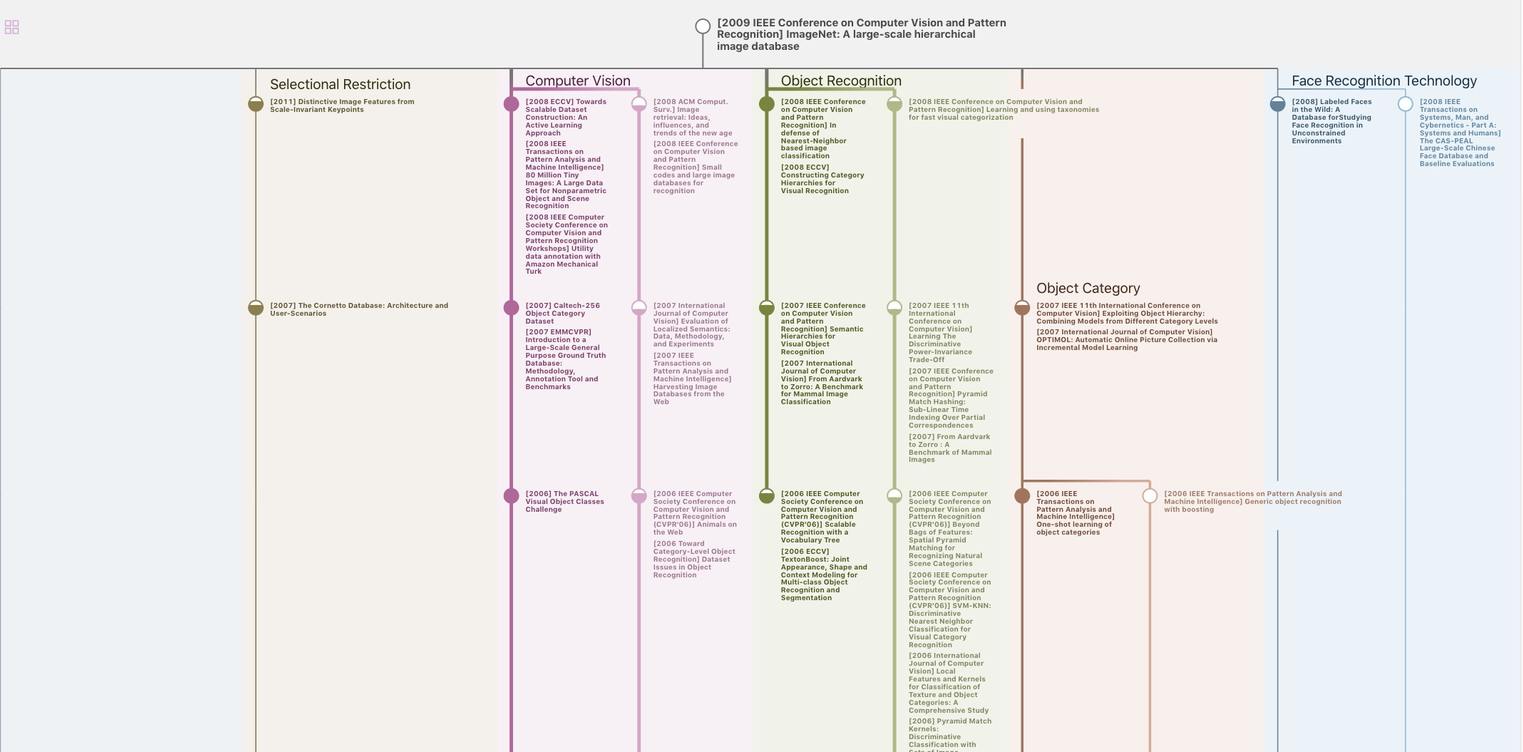
生成溯源树,研究论文发展脉络
Chat Paper
正在生成论文摘要