Metric Learning Based Framework For Streaming Classification With Concept Evolution
2019 INTERNATIONAL JOINT CONFERENCE ON NEURAL NETWORKS (IJCNN)(2019)
摘要
A primary challenge in label prediction over a stream of continuously occurring data instances is the emergence of instances belonging to unknown or novel classes. It is imperative to detect such novel-class instances quickly along the stream for a superior prediction performance. Existing techniques that perform novel class detection typically employ a clustering-based mechanism by observing that instances belonging to the same class (intra-class) are closer to each other (cohesion) than interclass samples (separation). While this is generally true in low dimensional feature spaces, we observe that such a property is not intrinsic among instances in complex real-world high-dimensional feature space such as images and text. In this paper, we focus on addressing this key challenge that negatively affects prediction performance of a data stream classifier. Concretely, we develop a metric learning mechanism that transforms high-dimensional features into a latent feature space to make above property holds true. Unlike existing metric learning method which only focus on classification task, our approach address the novel class detection and stream classification simultaneously. We showcase a framework along the stream to achieve larger prediction performance compared to existing state-of-the-art detection techniques while using the least amount of labeled data during detection. Extensive experimental results on simulated and real-world stream demonstrate the effectiveness of our approach.
更多查看译文
关键词
concept evolution,label prediction,data instances,novel-class instances,superior prediction performance,class detection,clustering-based mechanism,intra-class samples,streaming classification,stream classification,classification task,metric learning method,latent feature space,high-dimensional features,data stream classifier,real-world high-dimensional feature space,low dimensional feature spaces,inter-class samples
AI 理解论文
溯源树
样例
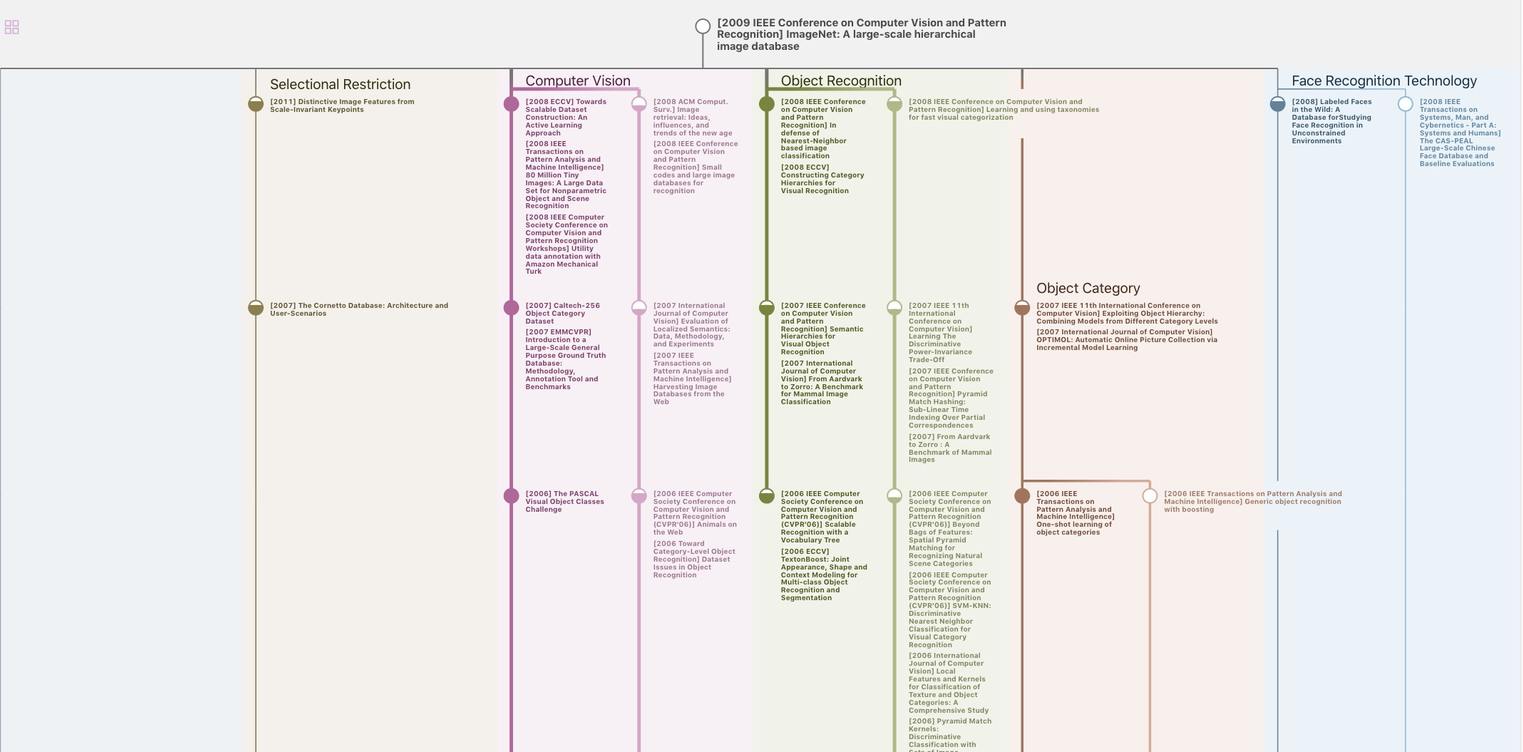
生成溯源树,研究论文发展脉络
Chat Paper
正在生成论文摘要