FRI0119 PREDICTING REMISSION AMONG PATIENTS WITH RHEUMATOID ARTHRITIS STARTING TOCILIZUMAB MONOTHERAPY: MODEL DERIVATION AND VALIDATION USING CONVENTIONAL REGRESSION AND MACHINE LEARNING
Poster presentations(2019)
摘要
Background: Most rheumatoid arthritis (RA) patients strive to consolidate their treatment from methotrexate combinations to monotherapy with the goal of the most effective and simplified therapy. Tocilizumab (TCZ) appears to have similar efficacy when used with or without concomitant csDMARDs in RA. Thus, it is clinically useful to predict treatment benefit among patients with RA starting TCZ monotherapy. Objectives: To identify RA patients most likely to achieve remission with TCZ monotherapy by developing and validating a prediction model through conventional regression and machine learning. Methods: We followed the TRIPOD recommendations for derivation and validation of clinical risk prediction models. Four TCZ monotherapy randomized controlled trials (RCT) in RA were identified (ACT-RAY, ADACTA, AMBITION, and FUNCTION) – two were chosen for derivation of the prediction models and two for validation. Relevant data for individual subjects from the trials were obtained, including demographics, RA characteristics, prior treatments, comorbid conditions, and baseline disease activity. In the two derivation trials, we examined each potential predictor of remission one at a time in logistic regression models, adjusted for age, sex, and baseline CDAI. The dependent variable in all models was CDAI ≤ 2.8 (remission) at 24 weeks. Three methods were used for variable selection: odds ratio (OR) based (forwarding variables to multivariable models if OR > 1.5 or < 0.66); AIC based (lowest AIC); and a machine learning approach that fit a logistic regression model to covariates selected by a LASSO-regularized logistic regression. Based on these different variable selection approaches, variables were advanced to the final multivariable model in the derivation cohort (DC). Age, sex, and baseline CDAI were forced into all models. We assessed discrimination with the area under the curve (AUC), model fit with the AIC and BIC, and calibration graphically using loess smoothing and with calibration slopes. We assessed model performance in the DC and in the validation cohort (VC), using variables selected in the DC. Results: Baseline characteristics in the derivation (n=473) and validation (n=380) cohorts were generally similar: mean age 51.6 years in DC and 52.1 in VC; 77% female in DC and 83% in VC; mean baseline CDAI 39.8 in DC and 42.1 in VC; disease duration was longer in VC 3.6 years compared to 0.9 in DC. Seventy-eight patients (17%) reached remission in the DC; 49 (13%) in the VC. After testing possible variables in the derivation models, the variables selected as predictors of remission included younger age, male sex, lower baseline CDAI, shorter RA disease duration, region of world (Europe and South America increased odds of remission vs. Asia and North America), no previous exposure to DMARD/MTX, lower baseline HAQ-DI, and baseline hematocrit (see Table). In the DC, model fit and calibration were adequate in all three models, though discrimination was notably reduced under 10 fold cross-validation. The VC modeling suggested that all three models were similar in discrimination and were well calibrated (see Table). Conclusion: Different modeling strategies selected similar variables to predict remission using TCZ monotherapy: shorter disease duration, lower HAQ-DI, and no prior DMARD/methotrexate use. While these results should be interpreted with caution, as the analysis combined different studies that may represent different populations, they may provide guidance to clinicians tailoring treatment options based on baseline characteristics. Disclosure of Interests: Jamie Collins Grant/research support from: genentech, Fredrik Johansson Grant/research support from: Genentech, Sara Gale Shareholder of: Roche, Employee of: Genentech/Roche, Seoyoung Kim Grant/research support from: Pfizer, Bristol-Myers Squibb, Roche/Genentech and AbbVie., Swastina Shrestha Grant/research support from: Genentech, David Sontag Grant/research support from: Genentech, Jacklyn Stratton Grant/research support from: Genentech, Trinh Huong Shareholder of: Roche, Employee of: Genentech/Roche, Chang Xu Grant/research support from: Pfizer, Elena Losina Grant/research support from: Genentech, Pfizer, Samumed and Flexion, Consultant for: Regeneron, Daniel Solomon Grant/research support from: Abbvie, Amgen, Genentech, Janssen, and Pfizer
更多查看译文
AI 理解论文
溯源树
样例
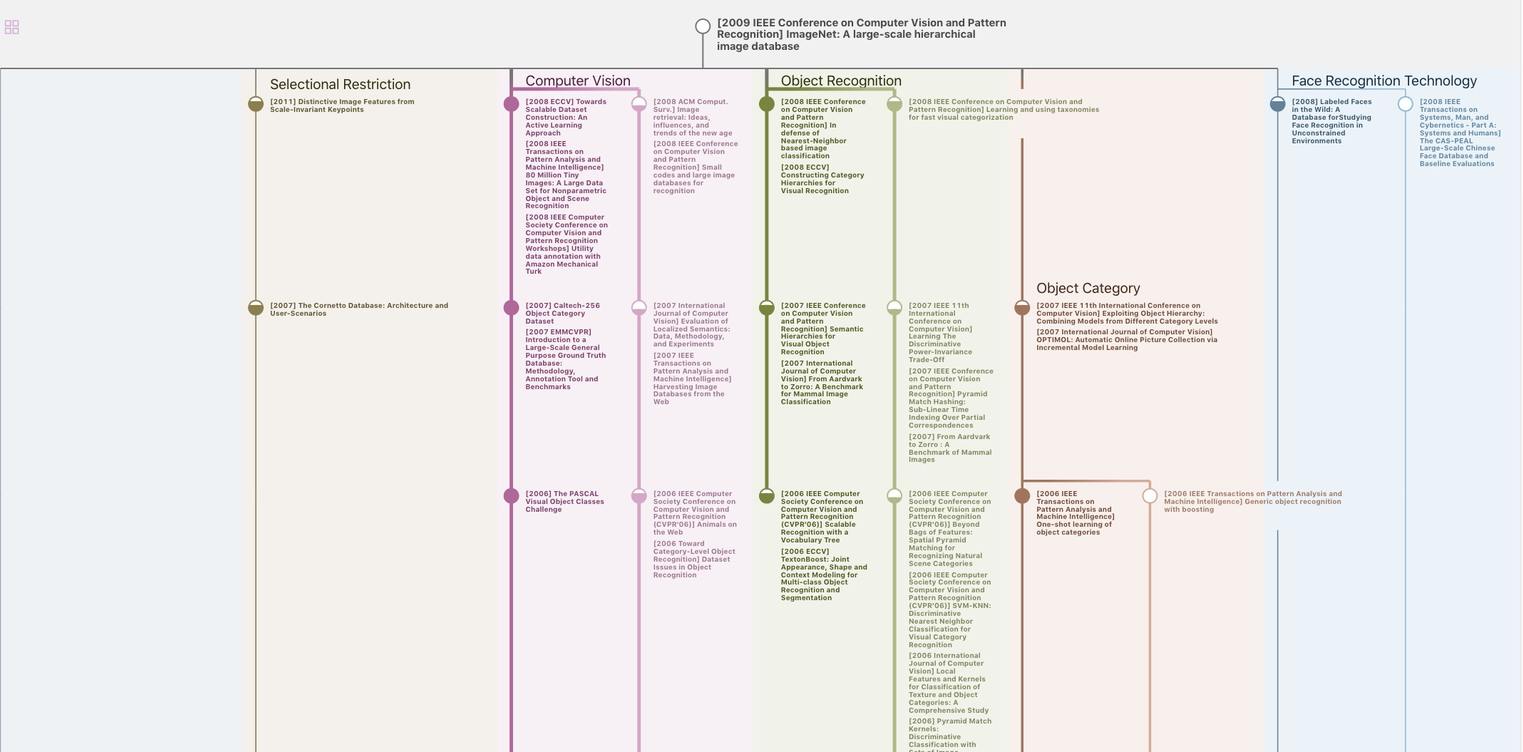
生成溯源树,研究论文发展脉络
Chat Paper
正在生成论文摘要