Enhancing Endoscopic Image Classification with Symptom Localization and Data Augmentation
Proceedings of the 27th ACM International Conference on Multimedia(2019)
摘要
Inspired by recent advances in computer vision and deep learning, we propose new enhancements to tackle problems appearing in endoscopic image analysis, especially abnormality finding and anatomical landmark detection. In details, a combination of Residual Neural Network and Faster R-CNN are jointly applied in order to take all of their advantages and improve the overall performance. Nevertheless, novel data augmentation is designed and adapted to corresponding domains. Our approaches prove their competitive results in term of not only the accuracy but also the inference time in Medico: The 2018 Multimedia for Medicine Task and The Biomedia ACM MM Grand Challenge 2019. These results show the great potential of the collaborating between deep learning models and data augmentation in medical image analysis applications. Especially, more than 4900 bounding boxes localizing the symptom of some classes from KVASIR dataset that we annotated and used in this project are shared online for future research.
更多查看译文
关键词
anatomical landmarks detection, endoscopic image, image classification, object detection, symptoms localization
AI 理解论文
溯源树
样例
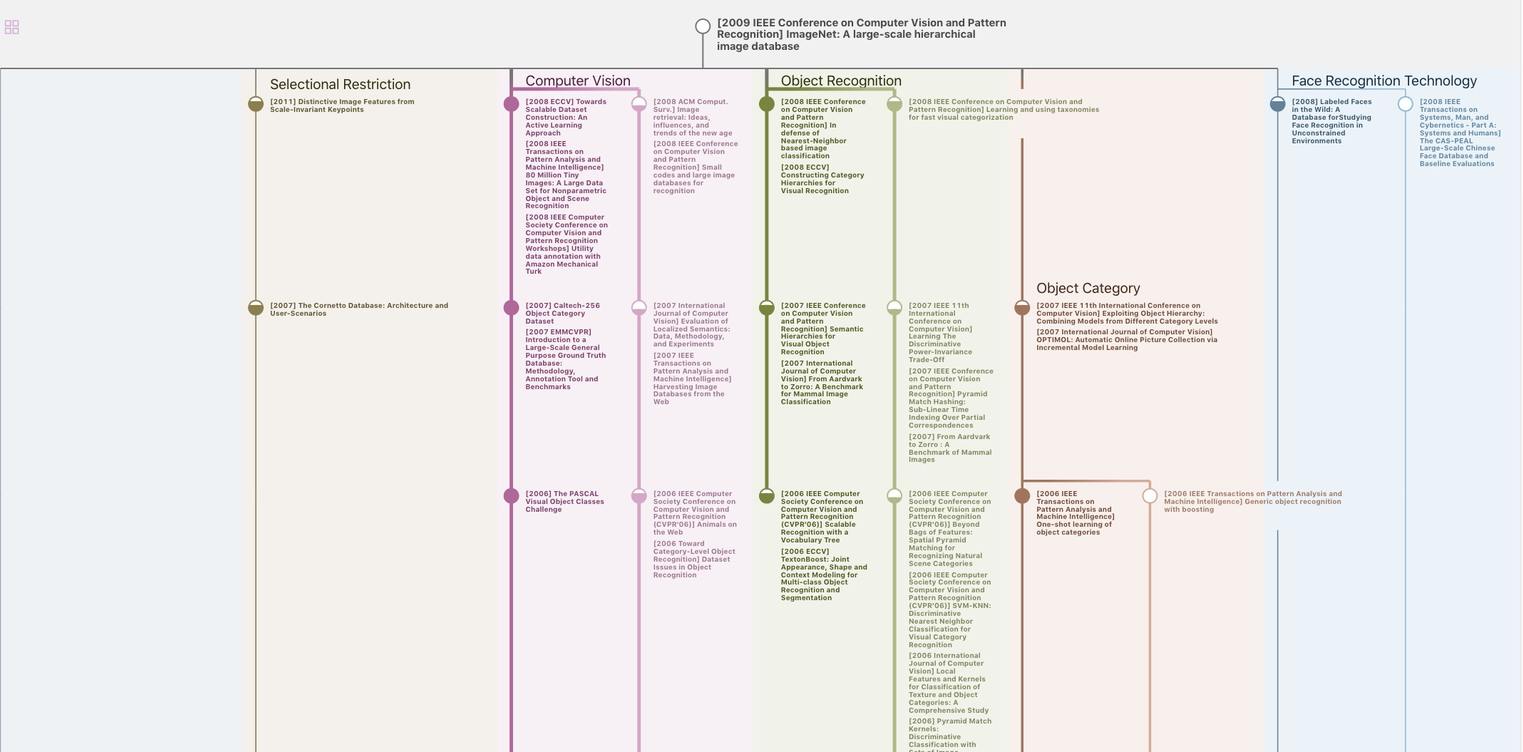
生成溯源树,研究论文发展脉络
Chat Paper
正在生成论文摘要