Supervised Mixture Models For Population Health
2019 IEEE INTERNATIONAL CONFERENCE ON BIOINFORMATICS AND BIOMEDICINE (BIBM)(2019)
摘要
We examine a machine learning approach for deriving insights from observational healthcare data in order to improve public health. Our goal is to simultaneously identify patient subpopulations with differing health risks and find the distinct risk factors or determinants associated with each subpopulation. Here, we develop a supervised Gaussian Mixture Model (GMM) approach for subpopulation modeling that combines GMMs with L1-logistic regression. We demonstrate the approach on an analysis of high cost drivers of Medicaid expenditures for inpatient stays associated with Newborn, Pregnancy, and Circulatory Systems diagnostic categories. These conditions were chosen because they had the highest total inpatient expenditures in New York State (NYS) in 2016. When compared with state-of-the-art learning methods (random forests, boosting, deep learning), our approach provides comparable prediction performance but also extracts insightful explanations of the subpopulation structure and risk factors within each subpopulation. Sequentially applying unsupervised learning methods and then applying logistic regression fails to yield equally meaningful results: the unsupervised subpopulations are homogeneous and moderately predictable, while some of our subpopulations are highly predictable with easy-to-identify drivers of cost. Focusing on newborns, we unveil subpopulations indicative of the landscape of healthcare in NYS: about 90% of the discharges are healthy New York City babies and about 1% are costly complex cases. Subpopulations indicate regional disparities: for example newborns from Central, Southern and Western NY are of higher risk for high-cost stays associated with substance abuse. The results indicate the promise of the approach for future population health studies based on electronic health care records.
更多查看译文
关键词
Machine Learning, Supervised Clustering Learning, Health Informatics, Subpopulation Detection
AI 理解论文
溯源树
样例
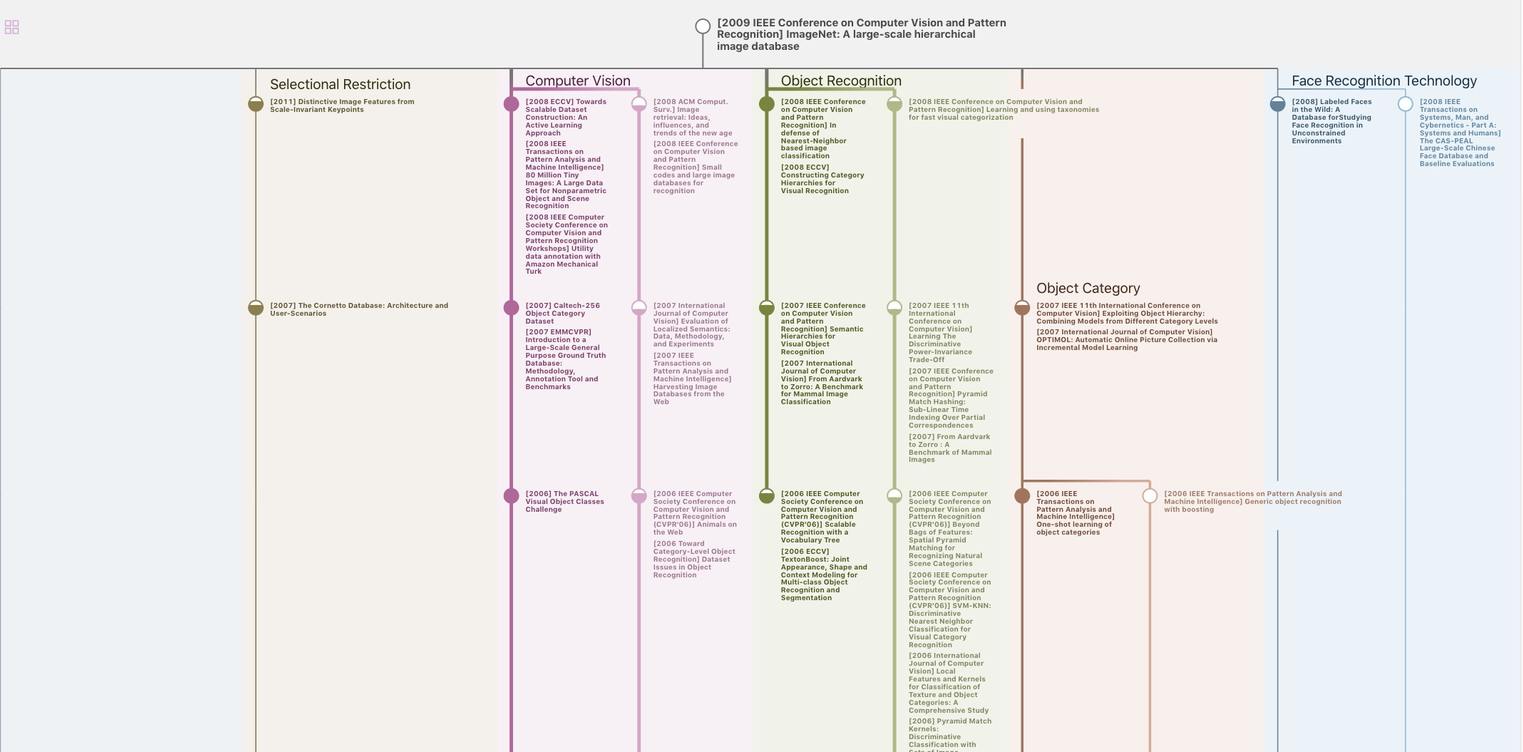
生成溯源树,研究论文发展脉络
Chat Paper
正在生成论文摘要