Transferable Force-Torque Dynamics Model for Peg-in-hole Task
arxiv(2019)
摘要
We present a learning-based force-torque dynamics to achieve model-based control for contact-rich peg-in-hole task using force-only inputs. Learning the force-torque dynamics is challenging because of the ambiguity of the low-dimensional 6-d force signal and the requirement of excessive training data. To tackle these problems, we propose a multi-pose force-torque state representation, based on which a dynamics model is learned with the data generated in a sample-efficient offline fashion. In addition, by training the dynamics model with peg-and-holes of various shapes, scales, and elasticities, the model could quickly transfer to new peg-and-holes after a small number of trials. Extensive experiments show that our dynamics model could adapt to unseen peg-and-holes with 70% fewer samples required compared to learning from scratch. Along with the learned dynamics, model predictive control and model-based reinforcement learning policies achieve over 80% insertion success rate. Our video is available at https://youtu.be/ZAqldpVZgm4.
更多查看译文
AI 理解论文
溯源树
样例
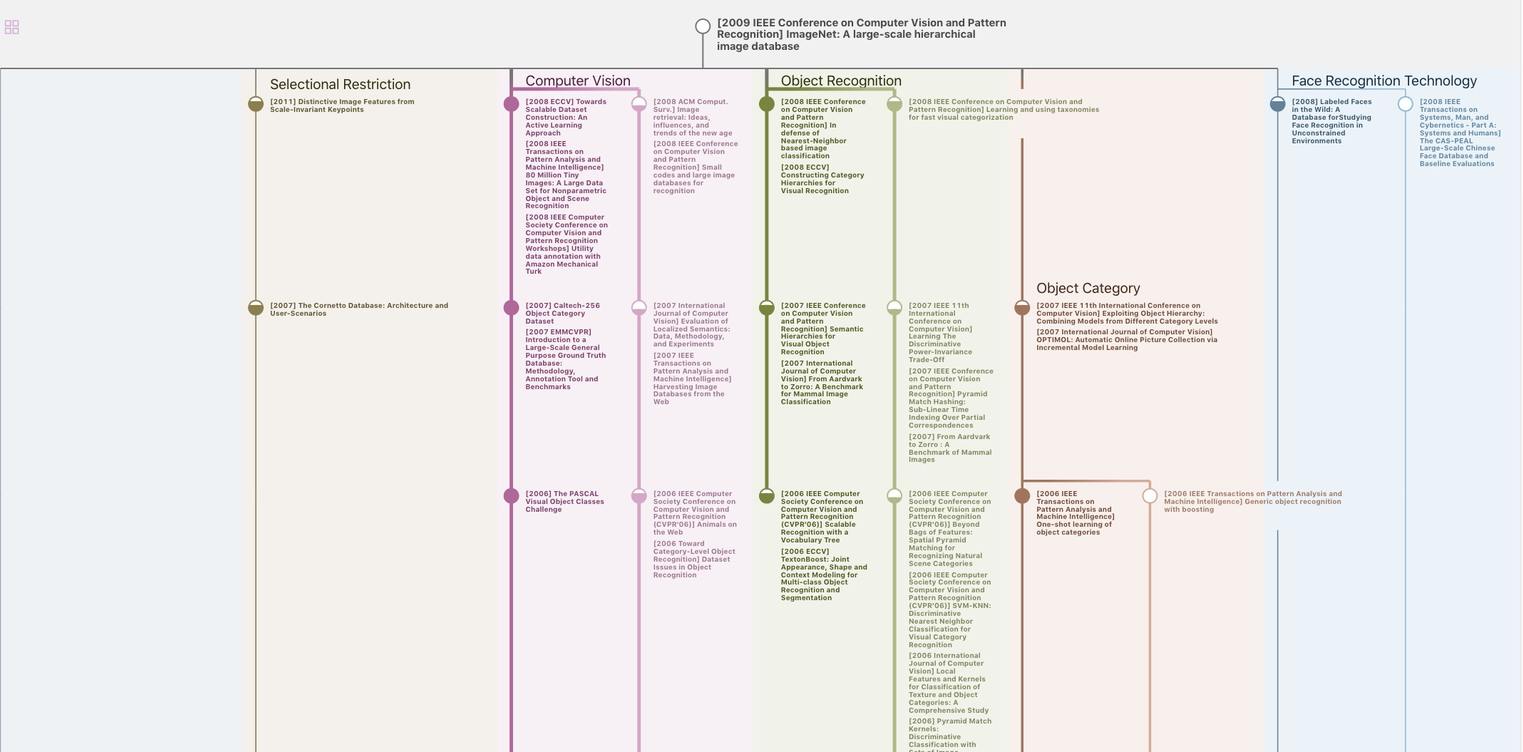
生成溯源树,研究论文发展脉络
Chat Paper
正在生成论文摘要