A Tradeoff Study Of Real-Time Traffic Prediction Approaches For Intelligent Transportation System
2019 IEEE INTELLIGENT TRANSPORTATION SYSTEMS CONFERENCE (ITSC)(2019)
摘要
Recent development on various sensing techniques has made real-time traffic data readily available. The growing traffic data sources facilitate a shift to the data-driven prediction modelling paradigm to support real-time Intelligent Transportation System (ITS) applications, such as traffic forecast, prediction of congestion, bus arrival and so on. Both prediction accuracy and computational time are key factors in supporting real-time ITS applications, especially in such dynamic environment. In this paper, we propose two methods of data-driven traffic prediction for urban intersections. A time-based implementation can provide greater details and accuracy while an event-based implementation can complete prediction faster. Extensive experiments are conducted to study the tradeoff between prediction accuracy and processing time; different metrics, such as travelling delay and stops, are used to evaluate the prediction accuracy achieved between the two methods of implementation. Results show that both models can achieve good accuracy performance in predicting vehicles' travelling delay. Specifically, the time-based prediction model can achieve 92% accuracy in predicting the in-queue state of a vehicle, while event-based prediction model can reduce 80% computation time compared to time-based prediction model.
更多查看译文
关键词
event-based prediction model,time-based prediction model,real-time traffic prediction approaches,real-time traffic data,data-driven prediction modelling paradigm,intelligent transportation system,real-time ITS applications
AI 理解论文
溯源树
样例
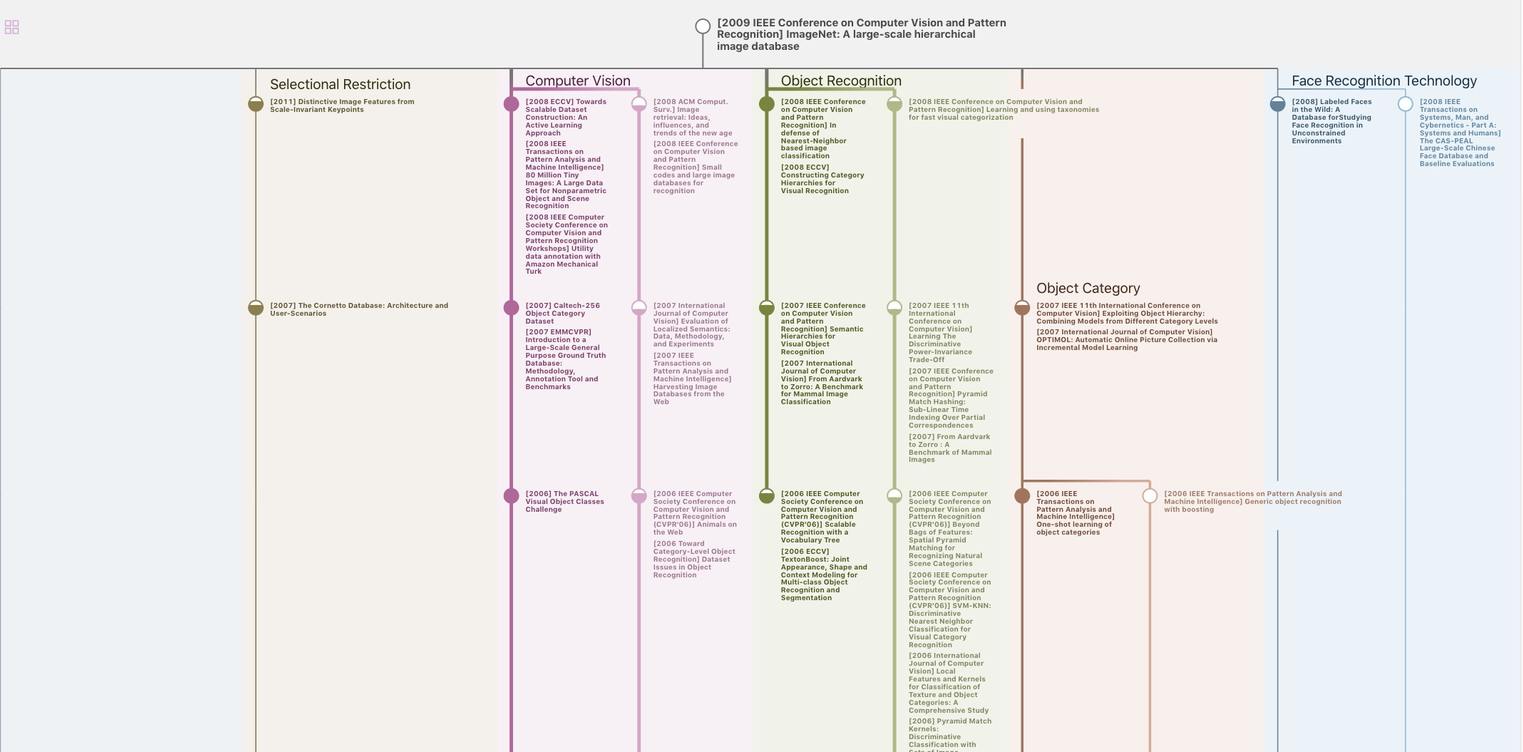
生成溯源树,研究论文发展脉络
Chat Paper
正在生成论文摘要