Optimizing Earth Moving Operations Via Reinforcement Learning
2019 WINTER SIMULATION CONFERENCE (WSC)(2019)
摘要
Earth moving operations are a critical component of construction and mining industries with a lot of potential for optimization and improved productivity. In this paper we combine discrete event simulation with reinforcement learning (RL) and neural networks to optimize these operations that tend to be cyclical and equipment-intensive. One advantage of RL is that it can learn near-optimal policies from the simulators with little human guidance. We compare three different RL methods including Q-learning, actor-critic, and trust region policy optimization and show that they all converge to significantly better policies than human-designed heuristics. We conclude that RL is a promising approach to automate and optimize earth moving and other similar expensive operations in construction, mining, and manufacturing industries.
更多查看译文
关键词
earth moving operations,reinforcement learning,critical component,mining industries,productivity,discrete event simulation,Q-learning method,actor-critic method,trust region policy optimization,construction industries,neural networks,human-designed heuristics,manufacturing industries
AI 理解论文
溯源树
样例
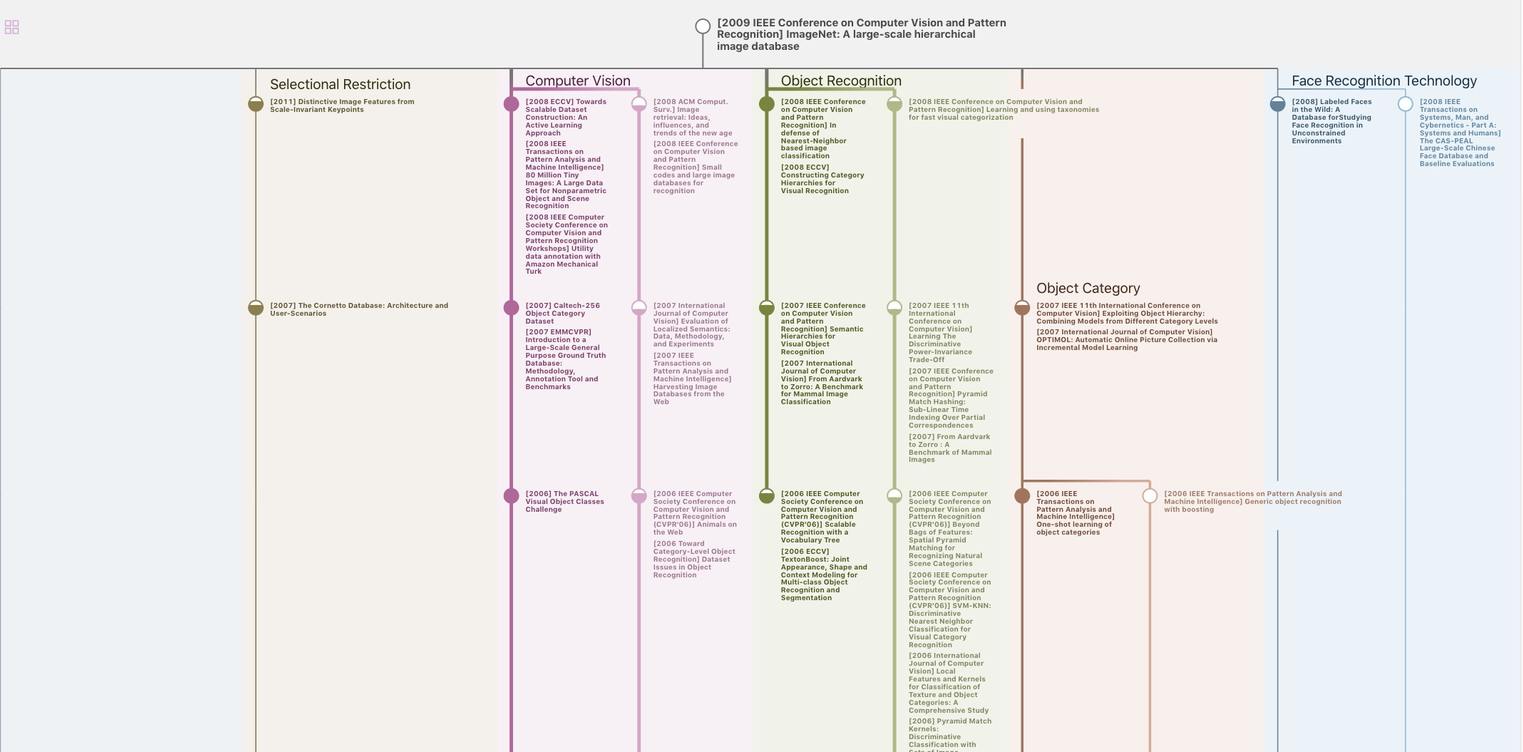
生成溯源树,研究论文发展脉络
Chat Paper
正在生成论文摘要