Rapidly Adaptable Legged Robots Via Evolutionary Meta-Learning
IEEE/RJS International Conference on Intelligent RObots and Systems(2020)
关键词
evolutionary strategies,high noise settings,robotics applications,quadruped robot,gradient-based approaches,adaptable legged robots,evolutionary meta-learning,adaptable policies,meta-learning method,meta-learning algorithms,second-order gradient estimation,noise-tolerant Batch Hill-Climbing adaptation operator,time 3.0 min
AI 理解论文
溯源树
样例
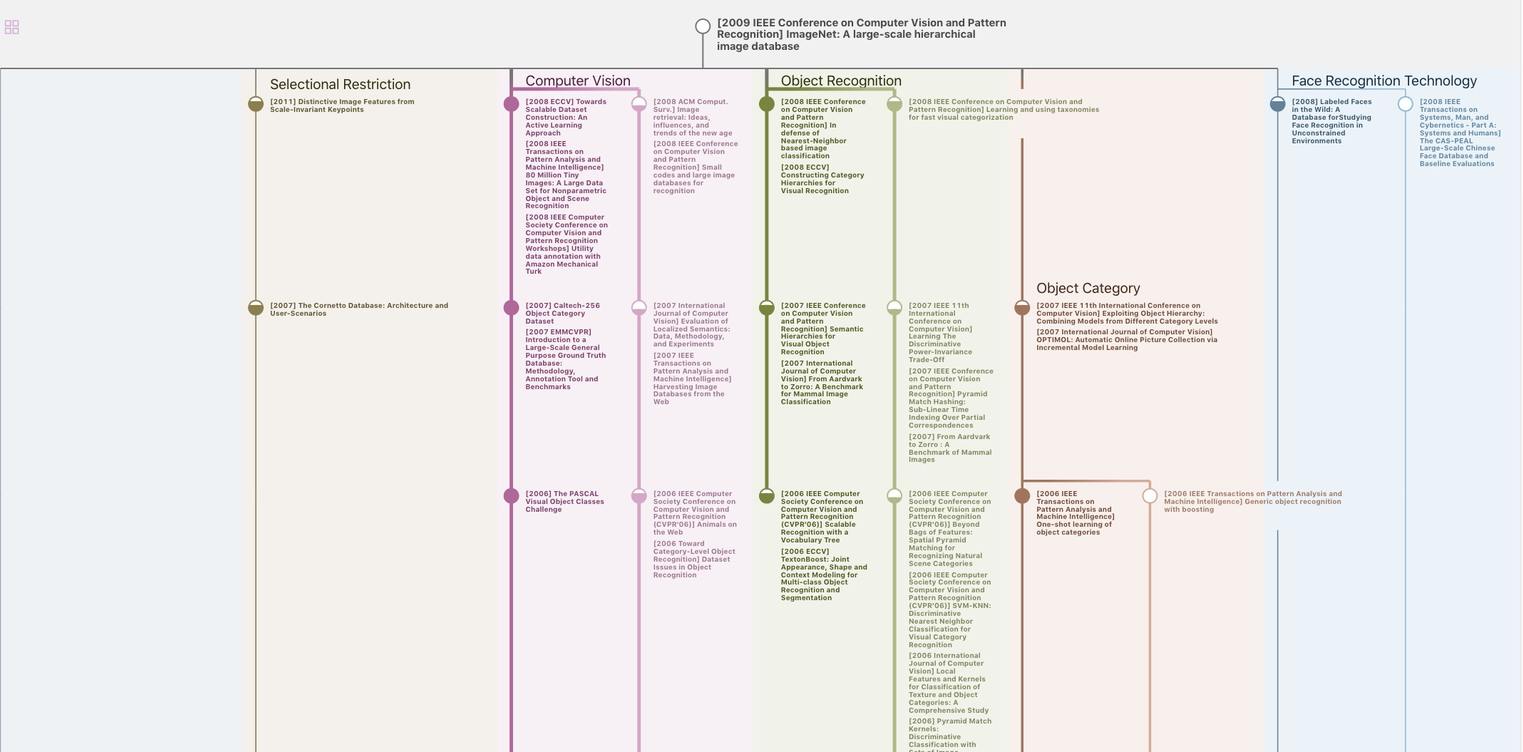
生成溯源树,研究论文发展脉络
Chat Paper
正在生成论文摘要