A Variable Batch Size Strategy for Large Scale Distributed DNN Training
2019 IEEE Intl Conf on Parallel & Distributed Processing with Applications, Big Data & Cloud Computing, Sustainable Computing & Communications, Social Computing & Networking (ISPA/BDCloud/SocialCom/SustainCom)(2019)
摘要
Large batch distributed synchronous stochastic gradient descent (SGD) has been widely used to train deep neural networks on a distributed memory system with multi-nodes, which can leverage parallel resources to reduce the number of iterative steps and speed up the convergence of training process. However, the large-batch SGD leads to a poor test accuracy, which would counteract the benefits of large scale parallel SGD. Existing solutions for large-batch training either significantly degrade accuracy or require massive additional hyper-parameter tuning. To overcome the difficulty above, we propose a novel variable batchsize strategy. With an in-depth analysis of the different stages in the recent multi-step schedule, we find that the training process at the first stage is sensitive to the batchsize, while different batchsize do not significantly impact the latter stages. Based on this discovery, we first claim that different stages of training should use different batchsize. Hence, the variable batchsize strategy is proposed for the large scale distributed training. Furthermore, in order to turn existing hyper-parameters automatically, an auto-tuning engine is designed for the variable batchsize strategy to achieve higher testing accuracy in the extremely large batchsize cases. By using our strategy, we successfully scale the batchsize to 120K in latter stages on ImageNet-1K with ResNet50 without accuracy loss and 128K with slight accuracy loss. The experimental evaluation on 2048 GPUs shows that the variable batchsize strategy with our auto-tuning engine could complete the training of ResNet-50 in 25 minutes. Furthermore, the new strategy successfully decreases the number of parameter updates by about 1.7 times compared with Facebook's multi-step schedule.
更多查看译文
关键词
Distributed Computing,Synchronous SGD,Large Batchsize,ImageNet-1K,Deep learning
AI 理解论文
溯源树
样例
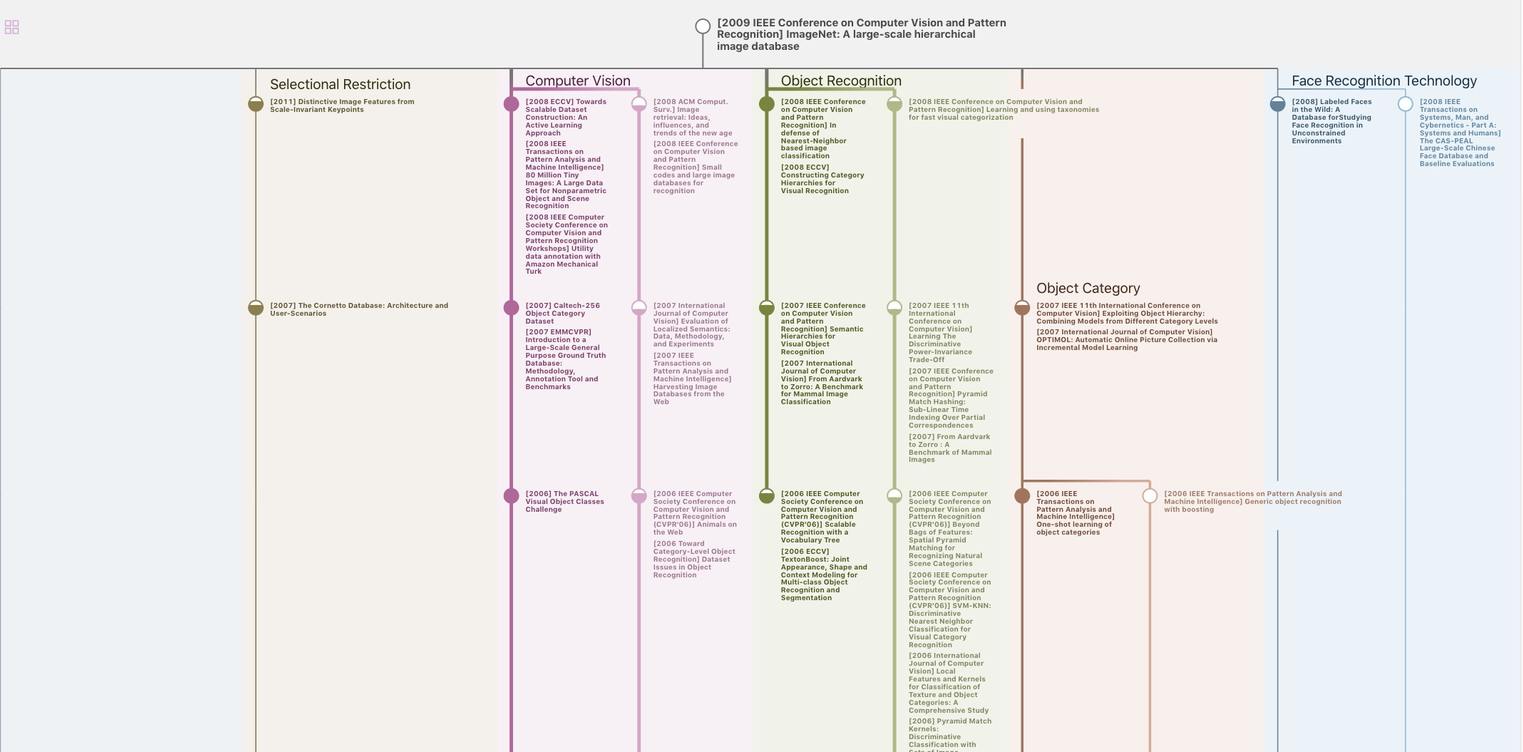
生成溯源树,研究论文发展脉络
Chat Paper
正在生成论文摘要