Goal-Oriented Obstacle Avoidance with Deep Reinforcement Learning in Continuous Action Space
ELECTRONICS(2020)
摘要
In this paper, we propose a goal-oriented obstacle avoidance navigation system based on deep reinforcement learning that uses depth information in scenes, as well as goal position in polar coordinates as state inputs. The control signals for robot motion are output in a continuous action space. We devise a deep deterministic policy gradient network with the inclusion of depth-wise separable convolution layers to process the large amounts of sequential depth image information. The goal-oriented obstacle avoidance navigation is performed without prior knowledge of the environment or a map. We show that through the proposed deep reinforcement learning network, a goal-oriented collision avoidance model can be trained end-to-end without manual tuning or supervision by a human operator. We train our model in a simulation, and the resulting network is directly transferred to other environments. Experiments show the capability of the trained network to navigate safely around obstacles and arrive at the designated goal positions in the simulation, as well as in the real world. The proposed method exhibits higher reliability than the compared approaches when navigating around obstacles with complex shapes. The experiments show that the approach is capable of avoiding not only static, but also dynamic obstacles.
更多查看译文
关键词
deep reinforcement learning,obstacle avoidance,map-less vector navigation,mixed-input network
AI 理解论文
溯源树
样例
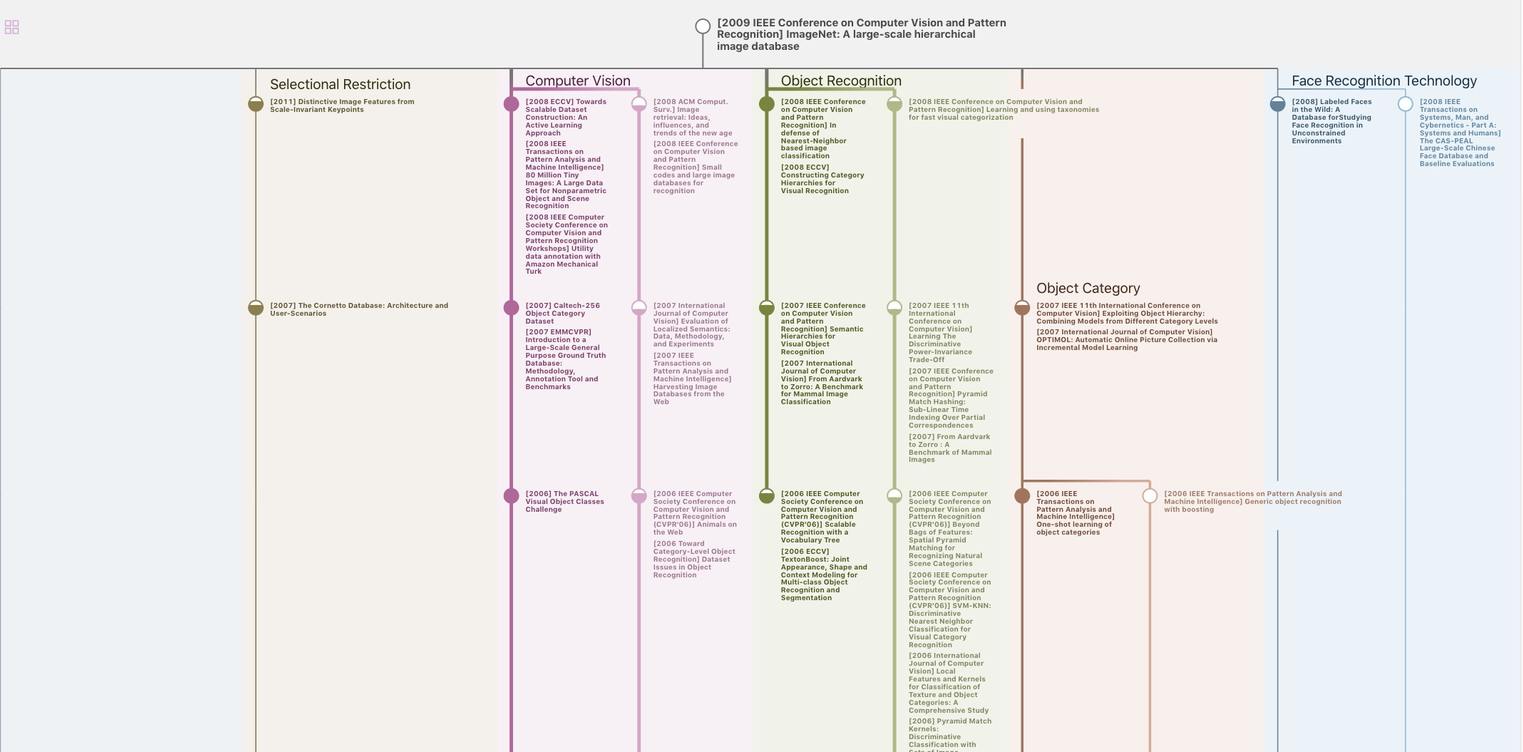
生成溯源树,研究论文发展脉络
Chat Paper
正在生成论文摘要