Differentiable Mapping Networks: Learning Structured Map Representations for Sparse Visual Localization
ICRA(2020)
摘要
Mapping and localization, preferably from a small number of observations, are fundamental tasks in robotics. We address these tasks by combining spatial structure (differentiable mapping) and end-to-end learning in a novel neural network architecture: the Differentiable Mapping Network (DMN). The DMN constructs a spatially structured view-embedding map and uses it for subsequent visual localization with a particle filter. Since the DMN architecture is end-to-end differentiable, we can jointly learn the map representation and localization using gradient descent. We apply the DMN to sparse visual localization, where a robot needs to localize in a new environment with respect to a small number of images from known viewpoints. We evaluate the DMN using simulated environments and a challenging real-world Street View dataset. We find that the DMN learns effective map representations for visual localization. The benefit of spatial structure increases with larger environments, more viewpoints for mapping, and when training data is scarce. Project website: https://sites.google.com/view/differentiable-mapping.
更多查看译文
关键词
sparse visual localization,structured mapping representations,networks
AI 理解论文
溯源树
样例
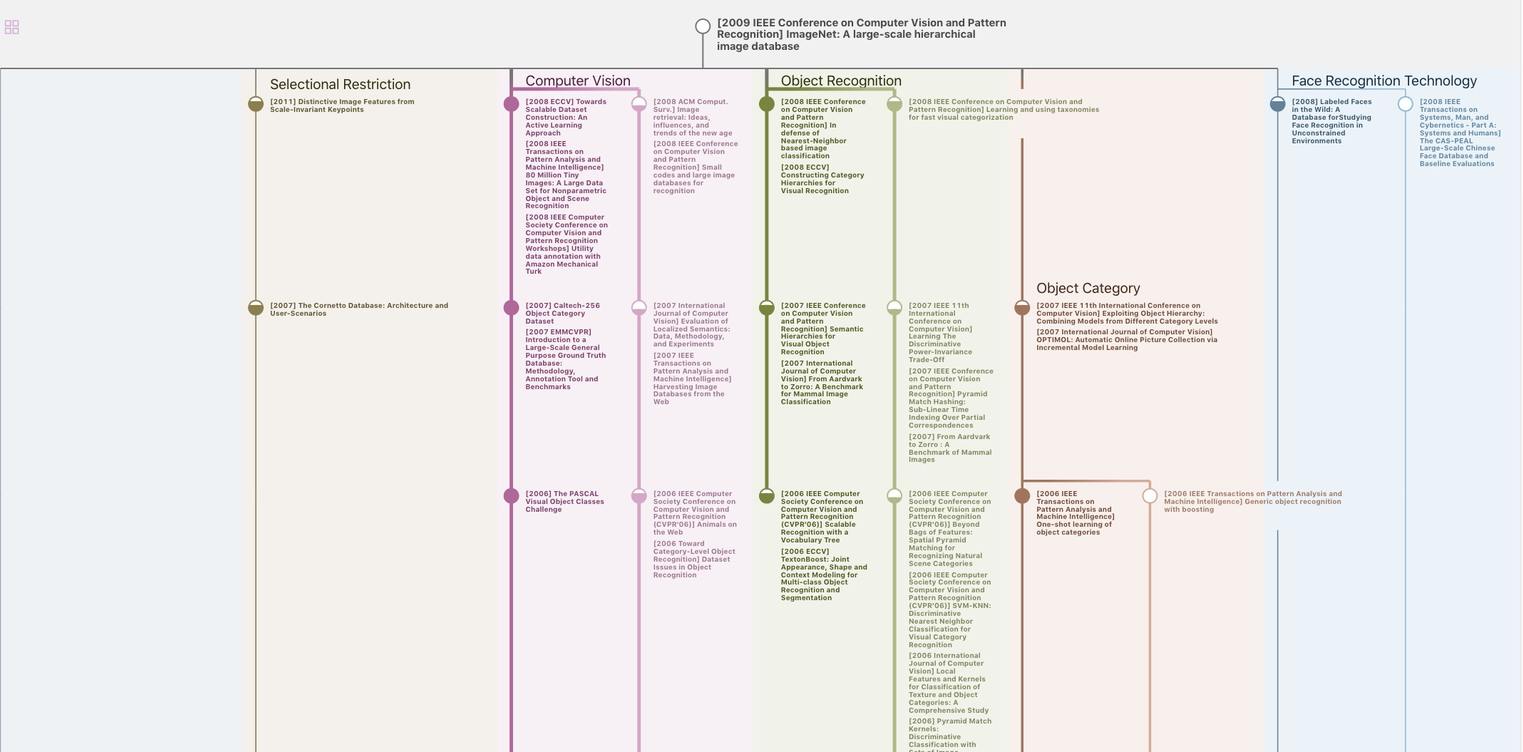
生成溯源树,研究论文发展脉络
Chat Paper
正在生成论文摘要