Boosting Few-Shot Learning With Adaptive Margin Loss
CVPR(2020)
摘要
Few-shot learning (FSL) has attracted increasing attention in recent years but remains challenging, due to the intrinsic difficulty in learning to generalize from a few examples. This paper proposes an adaptive margin principle to improve the generalization ability of metric-based meta-learning approaches for few-shot learning problems. Specifically, we first develop a class-relevant additive margin loss, where semantic similarity between each pair of classes is considered to separate samples in the feature embedding space from similar classes. Further, we incorporate the semantic context among all classes in a sampled training task and develop a task-relevant additive margin loss to better distinguish samples from different classes. Our adaptive margin method can be easily extended to a more realistic generalized FSL setting. Extensive experiments demonstrate that the proposed method can boost the performance of current metric-based meta-learning approaches, under both the standard FSL and generalized FSL settings.
更多查看译文
关键词
class-relevant additive margin loss,semantic similarity,feature embedding space,similar classes,semantic context,sampled training task,task-relevant additive margin loss,adaptive margin method,adaptive margin loss,intrinsic difficulty,adaptive margin principle,generalization ability,metric-based meta-learning approaches,few-shot learning problems,realistic generalized FSL
AI 理解论文
溯源树
样例
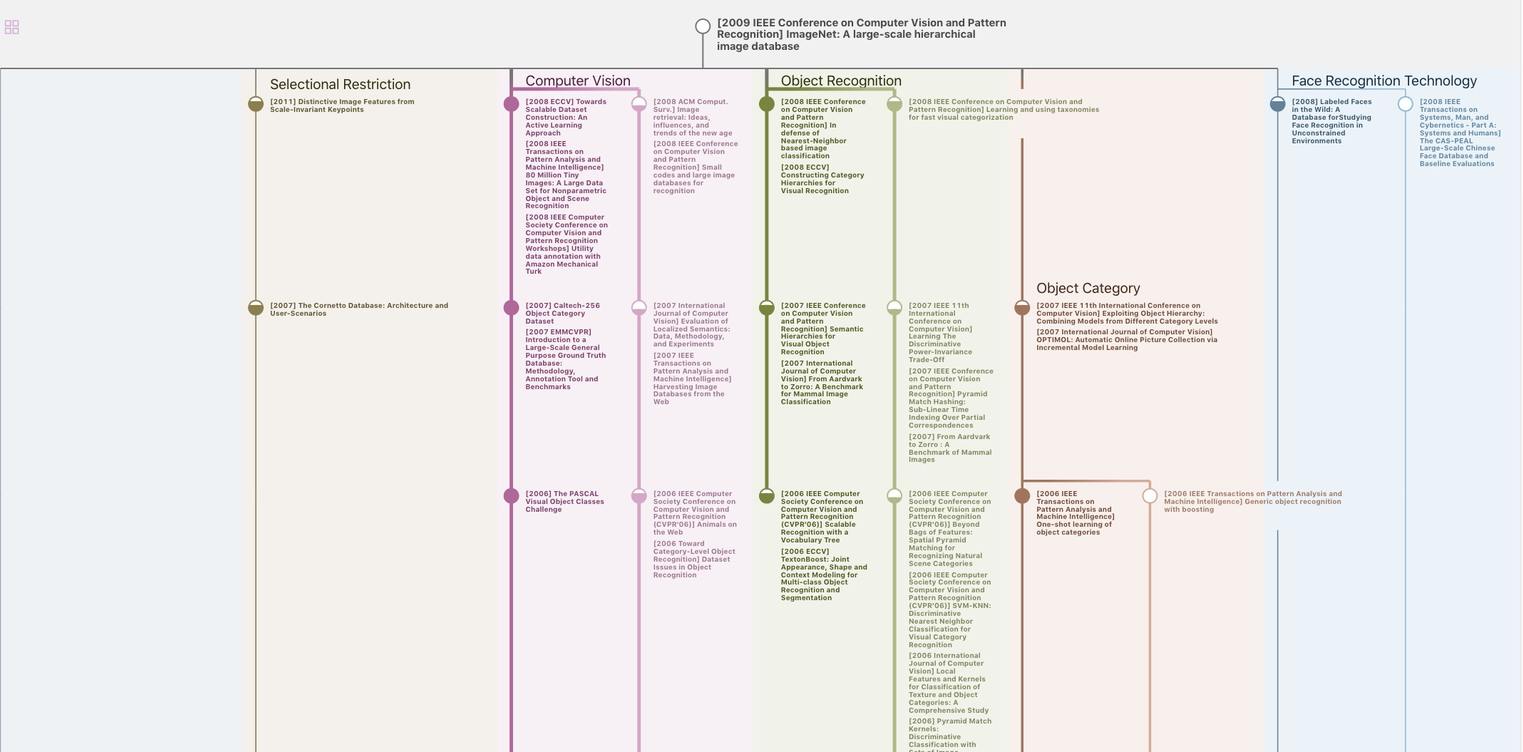
生成溯源树,研究论文发展脉络
Chat Paper
正在生成论文摘要