Recurrent Boosting Method for Time-Dependent Classification of Epileptiform Signals
Advances in Artificial Intelligence(2007)
摘要
Boosted ensemble classifiers have a demonstrated ability to discover regularities in large, poorly modeled datasets. In this paper we present an application of multihypothesis AdaBoost to detect epileptiform activity from electrophysiological recordings. While existing boosting methods do not account automatically for the sequence information that is available when analyzing timeseries data, we present a recurrent extension to AdaBoost, and show that it improves classification accuracy in our application domain.Medical treatment design has long been the exclusive domain of clinical experts. In recent years however, there has been a growing awareness of the difficulties involved in optimizing adaptive treatment strategies for the management of chronic diseases. The challenge is in developing sequences of treatments which automatically adapt to a patient’s characteristics and the disease’s progression. There are tremendous opportunities in applying automated learning and discovery techniques to this class of problems.
更多查看译文
AI 理解论文
溯源树
样例
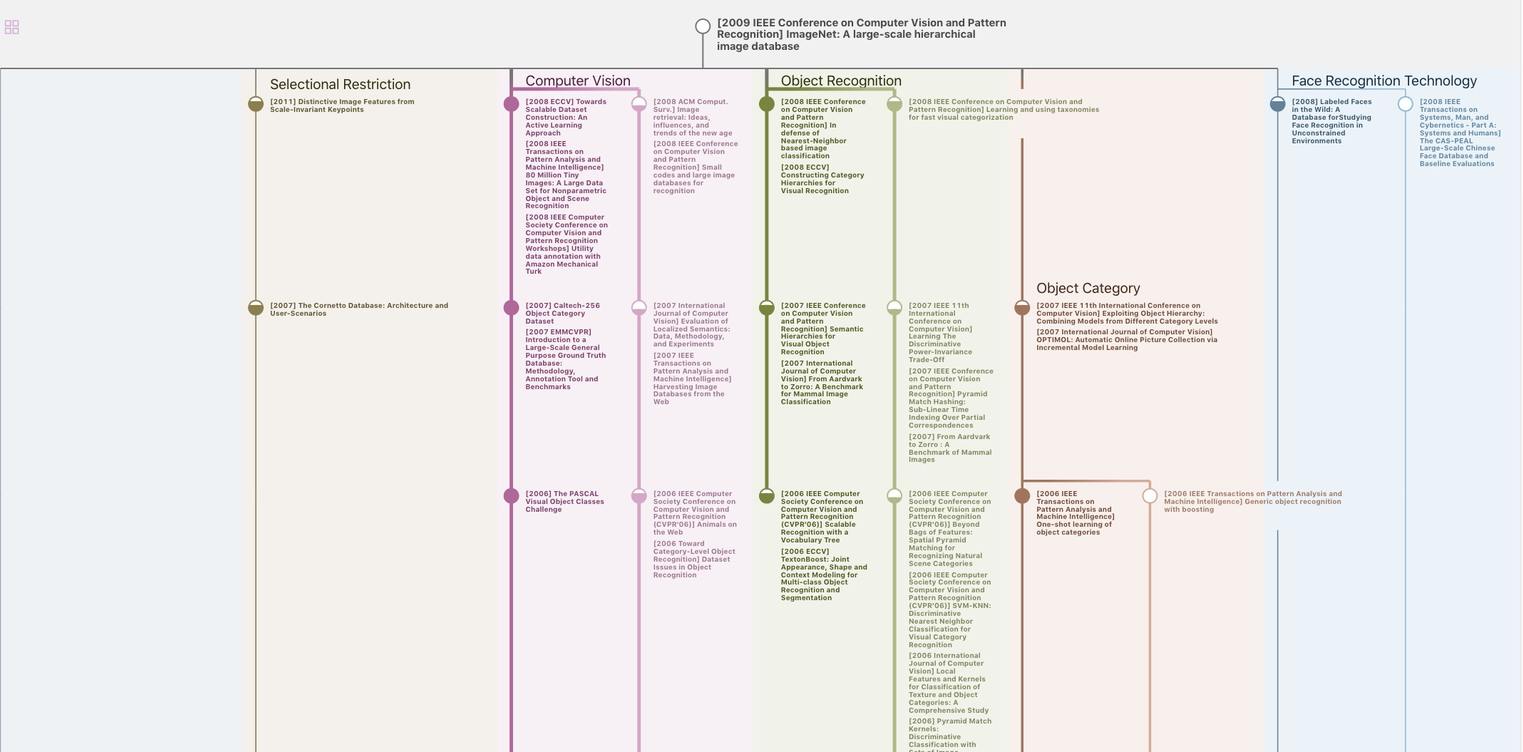
生成溯源树,研究论文发展脉络
Chat Paper
正在生成论文摘要