Intention-based Behavioral Anomaly Detection
user-5ebe27fd4c775eda72abcdc7(2019)
摘要
In this work we consider the problem of detecting anomalous deviations from expected behavioral trajectories generated by a multi-agent system. Common methods for time series anomaly detection do not explicitly consider the goal-directed nature of rational agents. This work proposes a method for detection of anomalous behaviors based on agent intent formulated using agent-based Lagrangian Mechanics. We propose an anomaly detection method that simultaneously learns to 1) predict the intended goals of agents from their trajectories, and 2) detect anomalies based on the predictions. The method uses a structured one-class support vector machine (structured OC-SVM), where latent variables represent the intentions (goals) of agents, and SVM weights represent the attraction or repulsion strength of potential goals and obstructions. Model parameters are learned in an unsupervised way. We conduct experiments in a marine surveillance setting, where we monitor high-traffic ports and detect anomalous vessels. Experimental results show that our algorithm shows promise for detecting anomalous behaviors in systems of goal-directed agents.
更多查看译文
AI 理解论文
溯源树
样例
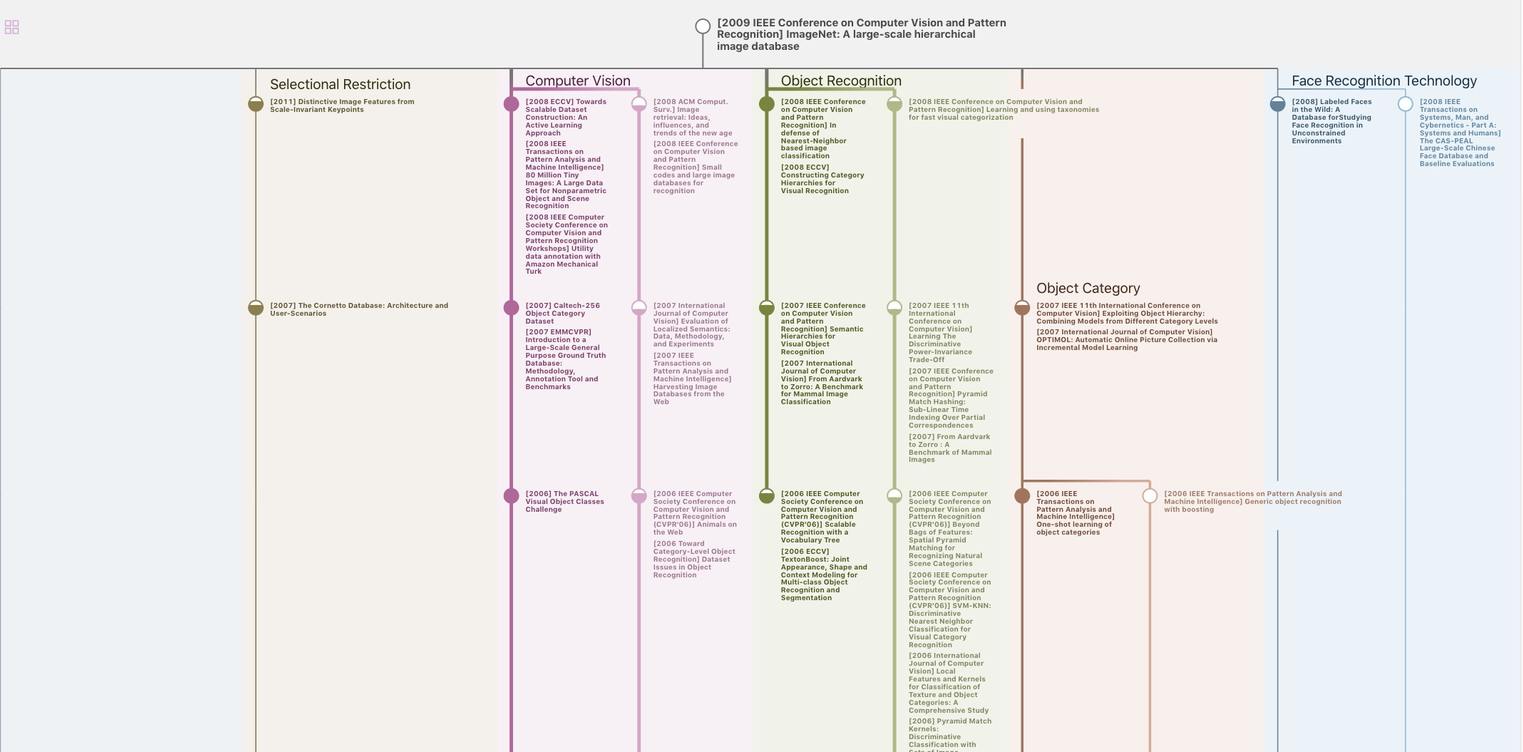
生成溯源树,研究论文发展脉络
Chat Paper
正在生成论文摘要