Learning to see before learning to act: Visual pre-training for manipulation
ICRA(2020)
摘要
Does having visual priors (eg the ability to detect objects) facilitate learning to perform vision-based manipulation (eg picking up objects)? We study this problem under the framework of transfer learning, where the model is first trained on a passive vision task, and adapted to perform an active manipulation task. We find that pre-training on vision tasks significantly improves generalization and sample efficiency for learning to manipulate objects. However, realizing these gains requires careful selection of which parts of the model to transfer. Our key insight is that outputs of standard vision models highly correlate with affordance maps commonly used in manipulation. Therefore, we explore directly transferring model parameters from vision networks to affordance prediction networks, and show that this can result in successful zero-shot adaptation, where a robot can pick up certain objects with zero robotic experience …
更多查看译文
关键词
visual priors,vision-based manipulation,transfer learning,passive vision task,data distribution,active manipulation task,affordance maps,vision networks,zero-shot adaptation,zero robotic experience,visual pre-training,object detection,object manipulation,affordance prediction networks
AI 理解论文
溯源树
样例
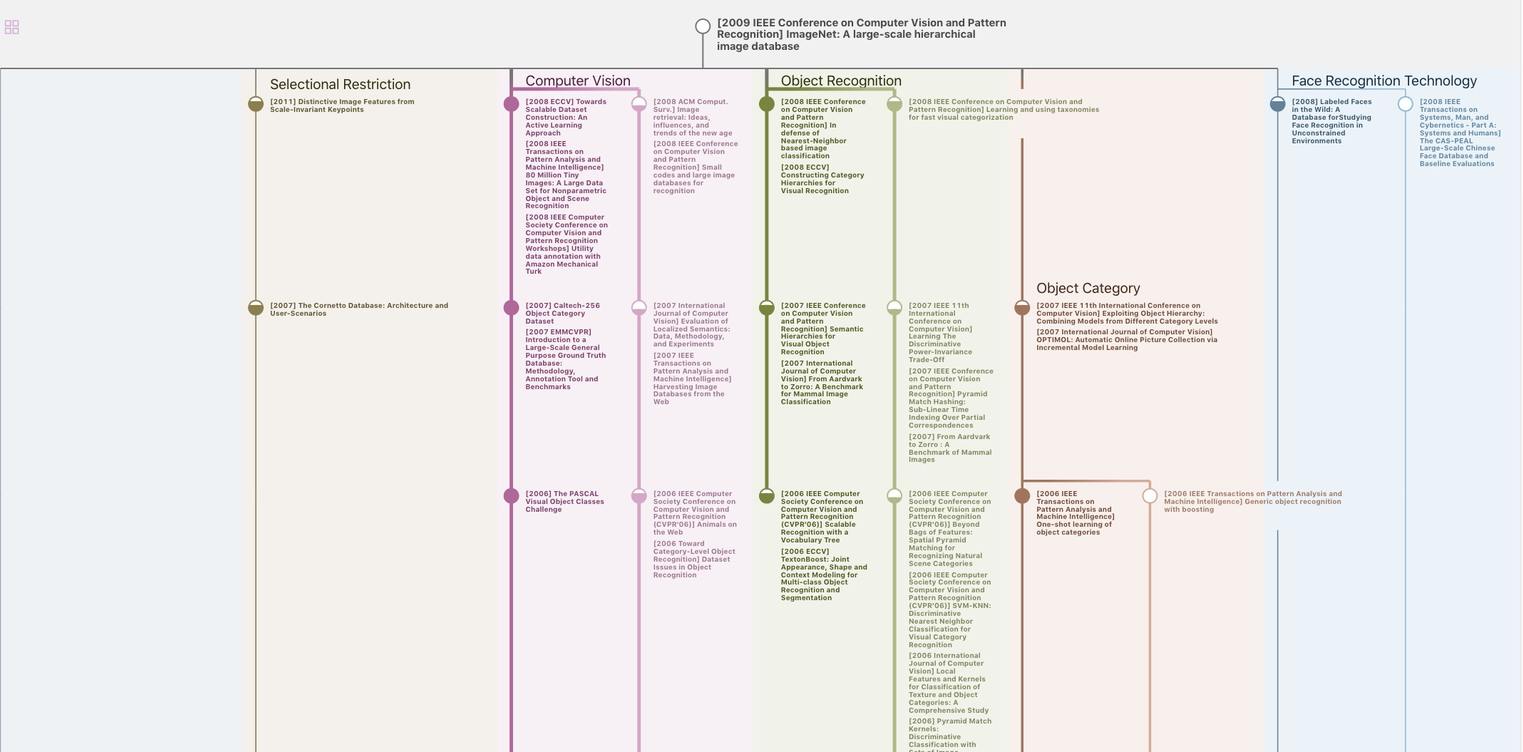
生成溯源树,研究论文发展脉络
Chat Paper
正在生成论文摘要