Learning From Multi-Dimensional Partial Labels
IJCAI 2020(2020)
摘要
Multi-dimensional classification (MDC) has attracted much attention from the community. Though most studies consider fully annotated data, in real practice obtaining fully labeled data in MDC tasks is usually intractable. In this paper, we propose a novel learning paradigm: Multi-Dimensional Partial Label Learning (MDPL) where the ground-truth labels of each instance are concealed in multiple candidate label sets. We first introduce the partial hamming loss for MDPL that incurs a large loss if the predicted labels are not in candidate label sets, and provide an empirical risk minimization (ERM) framework. Theoretically, we rigorously prove the conditions for ERM learnability of MDPL in both independent and dependent cases. Furthermore, we present two MDPL algorithms under our proposed ERM framework. Comprehensive experiments on both synthetic and real-world datasets validate the effectiveness of our proposals.
更多查看译文
AI 理解论文
溯源树
样例
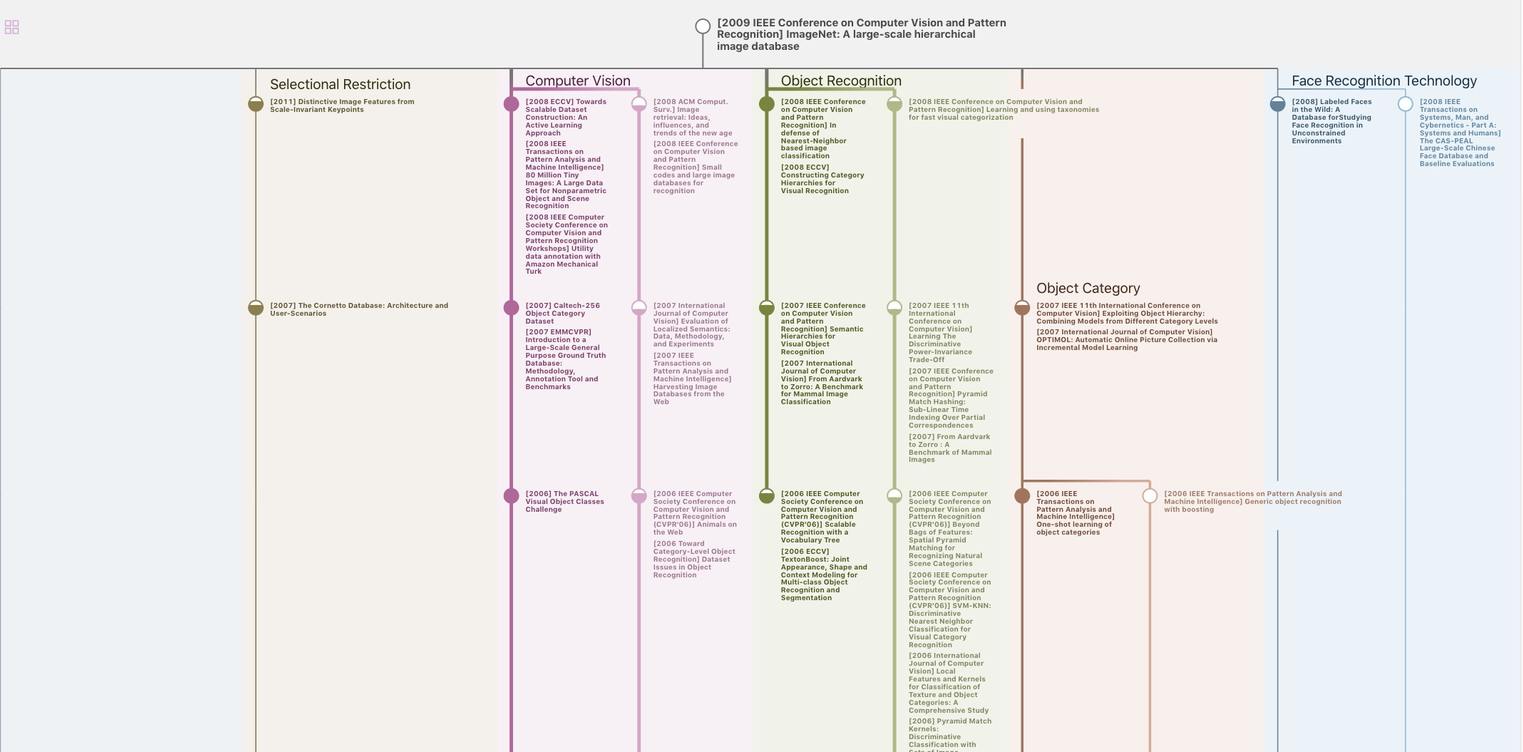
生成溯源树,研究论文发展脉络
Chat Paper
正在生成论文摘要