Adaptive Multi-Task Dual-Structured Learning with Its Application on Alzheimer’s Disease Study
ACM Transactions on Internet Technology(2021)
摘要
AbstractMulti-task learning has been widely applied to Alzheimer’s Disease (AD) studies due to its capability of simultaneously rating the disease severity (classification) and predicting corresponding clinical scores (regression). In this article, we propose a novel technique of Adaptive Multi-task Dual-Structured Learning, named AMDSL, by mutually exploring the dual manifold structure for the label and regression score of the disease data under joint classification and regression tasks, while learning an adaptive shared similarity measure and corresponding feature mapping among these two tasks. We encode both the reconstructed label representation and regression score adaptive to the ideal similarity measure on disease data to achieve the ideal performance on these two joint tasks. The alternating algorithm is proposed to optimize the above objective. We theoretically prove the convergence of the optimization algorithm. The superiority of AMDSL is experimentally validated under joint classification and regression as per various evaluation metrics against the most authoritative Alzheimer’s disease data.
更多查看译文
关键词
Multi-task learning, Alzheimer's predictive model
AI 理解论文
溯源树
样例
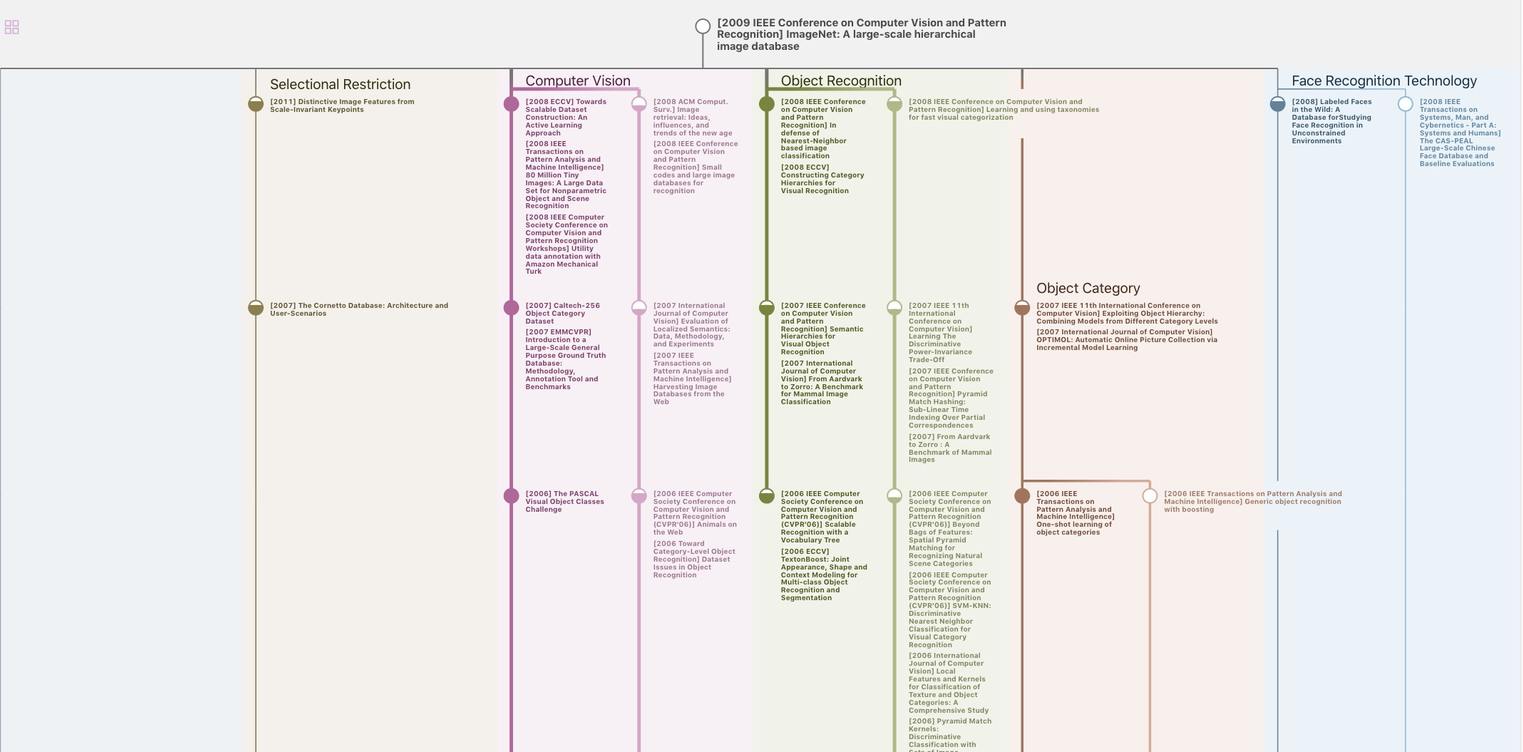
生成溯源树,研究论文发展脉络
Chat Paper
正在生成论文摘要