Learning Stable Graphs from Multiple Environments with Selection Bias
KDD 2020(2020)
摘要
Nowadays graph has become a general and powerful representation to describe the rich relationships among different kinds of entities via the underlying patterns encoded in its structure. The knowledge (more generally) accumulated in graph is expected to be able to cross populations from one to another and the past to future. However the data collection process of graph generation is full of known or unknown sample selection biases, leading to spurious correlations among entities, especially in the non-stationary and heterogeneous environments. In this paper, we target the problem of learning stable graphs from multiple environments with selection bias. We purpose a Stable Graph Learning (SGL) framework to learn a graph that can capture general relational patterns which are irrelevant with the selection bias in an unsupervised way. Extensive experimental results from both simulation and real data demonstrate that our method could significantly benefit the generalization capacity of graph structure.
更多查看译文
关键词
Graph Structure, Stability, Multiple Environments, Selection Bias
AI 理解论文
溯源树
样例
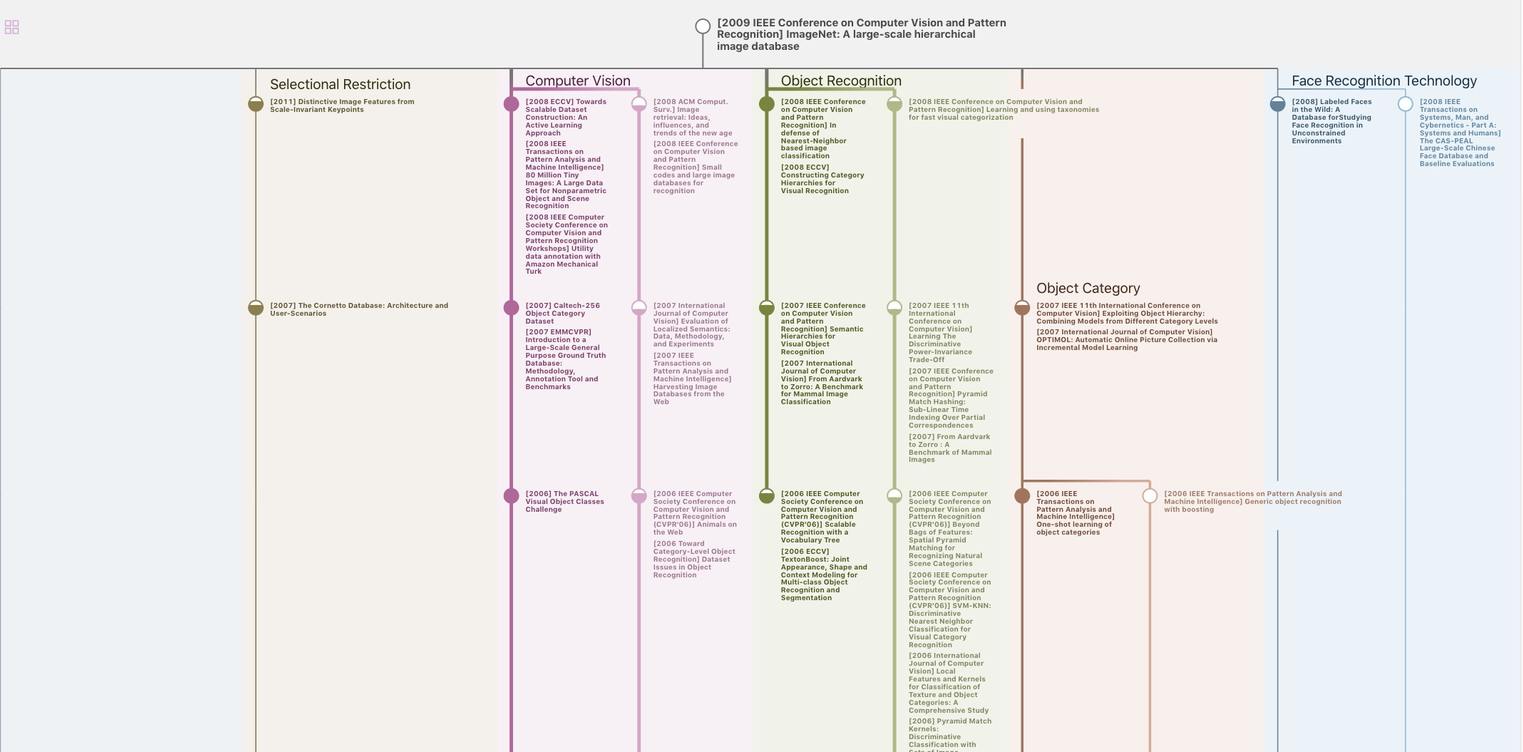
生成溯源树,研究论文发展脉络
Chat Paper
正在生成论文摘要