A Machine Learning Approach for the Classification of Disease Risks in Time Series
2020 9th Mediterranean Conference on Embedded Computing (MECO)(2020)
摘要
In this work, a new hybrid algorithm for disease risk classification is proposed. The proposed methodology is based on Dynamic Time Warping (DTW). This methodology can be applied to time series from various domains such as vital sign time series available in medical big data. To validate our methodology, we applied it to risk classification for sepsis, which is one of the most challenging problems within the area of medical data analysis. In the first step the algorithm uses different statistical properties of time series features. Furthermore, using differently labeled training data sets, we created a DTW Barycenter Averaging (DBA) on each feature. In the second step, validation data sets and DTW are used to validate the precision of classification and the final results are compared. The performance of our methodology is validated with real medical data and on six different criteria definitions for the sepsis diseases. Results show that our algorithm performed, in the best case, with precision and recall of 96,38% and 90,90%, respectively.
更多查看译文
关键词
Machine Learning,Time Series,Dynamic Time Warping,Data Mining,Sepsis
AI 理解论文
溯源树
样例
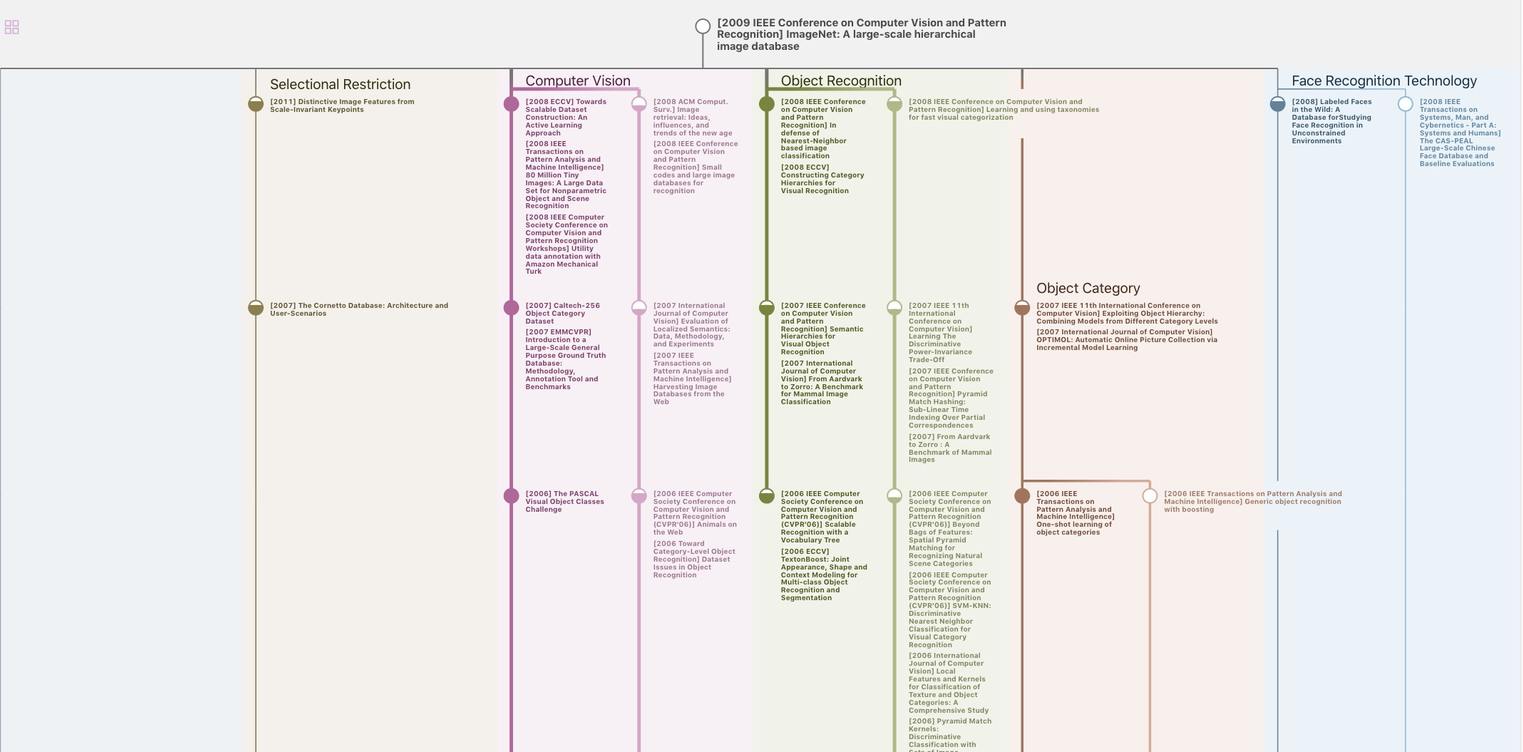
生成溯源树,研究论文发展脉络
Chat Paper
正在生成论文摘要