Detection of Hazardous Road Events From Audio Streams: An Ensemble Outlier Detection Approach
2020 IEEE Conference on Evolving and Adaptive Intelligent Systems (EAIS)(2020)
摘要
Change detection in audio data streams has become an efficient way for online notification of events. An interesting application is audio surveillance, including road traffic monitoring and online car crash alarms. However, in the particular case of crash alarms, most of the collected sounds are background noises, with various sub-classes, such as talking pedestrians, engine sounds, horn blowing, etc., whereas those corresponding to the event of interest are much less abundant. Therefore, it is difficult to apply classical tools of classification or clustering to detect crash sounds as a particular class or as outliers. To tackle this problem, we propose an ensemble classifier based on one-class SVM in order to separate outliers from normal data first, and deep neural networks to classify event-related data. Finally the results of both outlier detection and classification outputs are aggregated in such a way that outliers are considered as a novel class, a priori unknown by the DNN classifier. The application of this method on an audio traffic monitoring database confirms its ability to detect, (a) non-events (background noise), nonhazardous and hazardous event, and (b) non-accidents and accidents, from a stream of audio data.
更多查看译文
关键词
Change detection,audio data streams,one-class support vector machines (SVM),deep neural networks (DNN),ensemble classifier
AI 理解论文
溯源树
样例
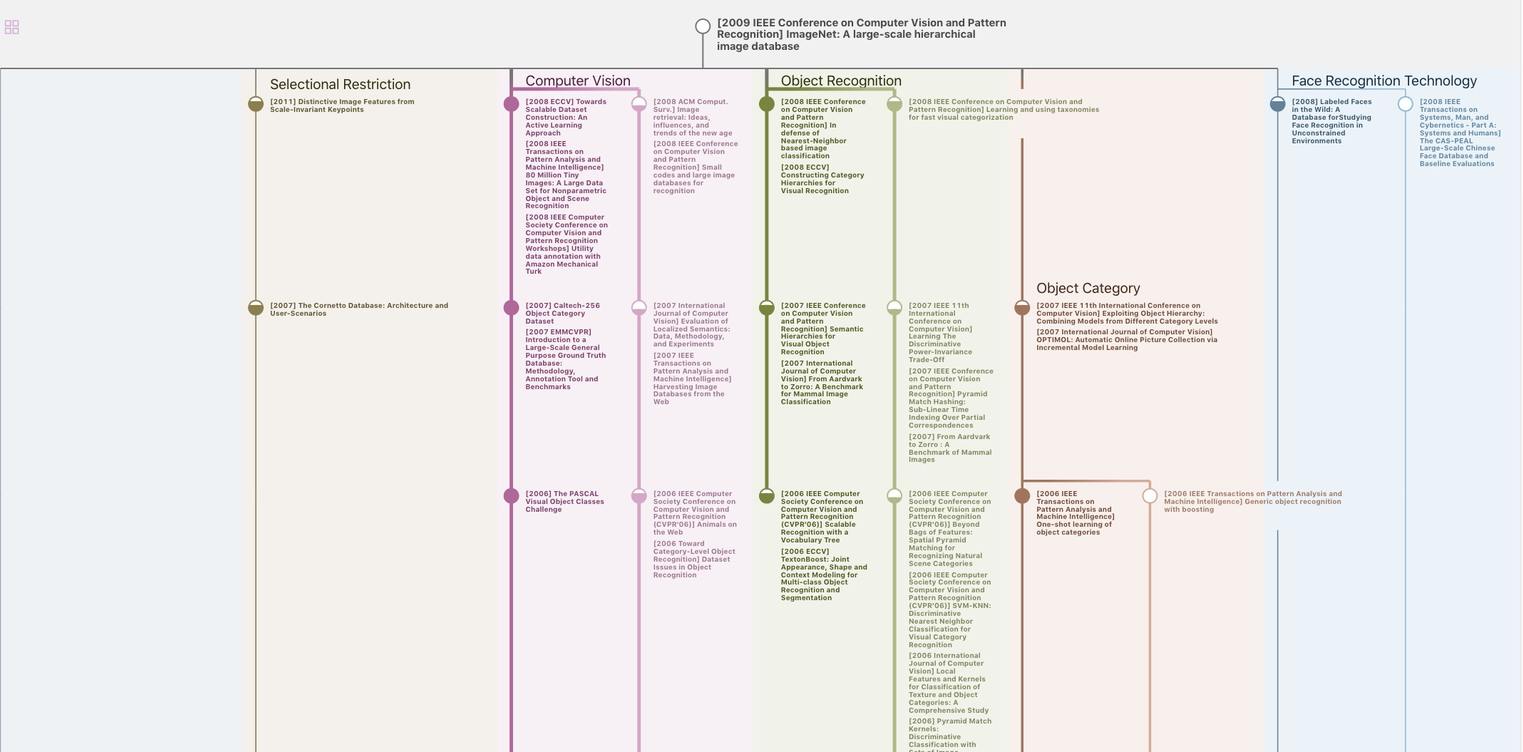
生成溯源树,研究论文发展脉络
Chat Paper
正在生成论文摘要