Towards Production-Grade, Platform-Independent Distributed ML
semanticscholar(2016)
摘要
Most existing frameworks for distributed machine learning are either tied to a specific data platform, or focus on novel computational and communication abstractions. The latter often neglect the constraints of shared-use clusters, such as fault tolerance, fair resource (network, CPU) usage, and isolation. This paper proposes a new distributed ML framework, SALMON, that abstracts the key components (control flow, partitioned data store, group communication) and relies only on above-resource-manager platform dependencies (via Apache REEF). The resulting framework is both expressive for common ML algorithm patterns (e.g., iterative MapReduce and parameter server), and flexible to operate on a variety of conventional, shared-use platforms (e.g., Apache Hadoop and HPC). Early experiments demonstrate the promise of this approach via comparisons with Apache Spark on a large-scale production dataset.
更多查看译文
AI 理解论文
溯源树
样例
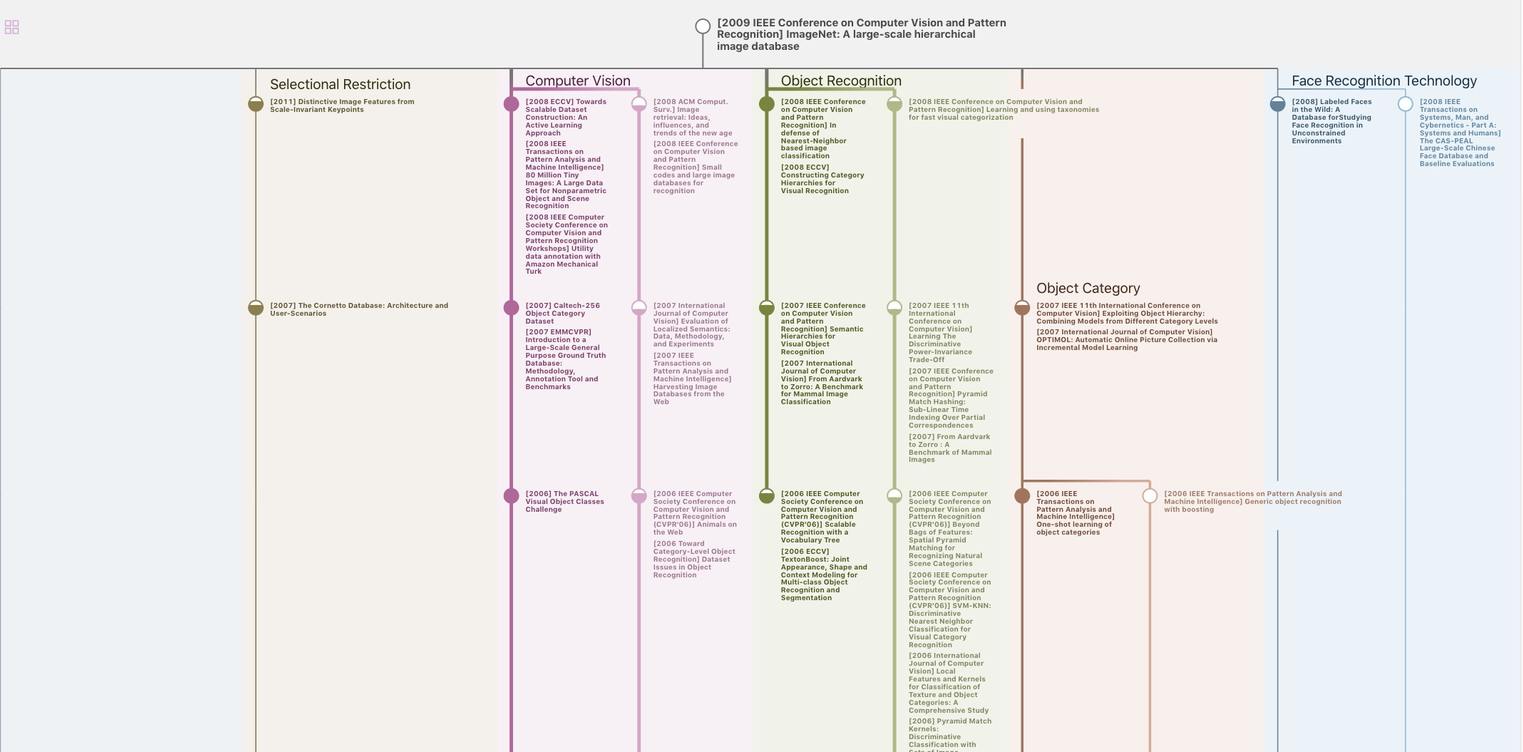
生成溯源树,研究论文发展脉络
Chat Paper
正在生成论文摘要