Lifting the Curse of Multidimensional Data with Learned Existence Indexes
semanticscholar(2018)
摘要
Bloom filters are a form of existence index which offer compressed representations of sets at the cost of some false positives. Recent work has introduced the notion of learned Bloom filters, which can leverage salient differences between in-index elements and out-of-index queries to offer further compression. In this extended abstract, we show that learned filters can handle multidimensional data particularly well, giving space savings of up to 72% in our experiments. We show how to maximize performance by leveraging three key optimizations: separate modeling of high-cardinality versus low-cardinality dimensions, Bloom filter sandwiching, and robust learning.
更多查看译文
AI 理解论文
溯源树
样例
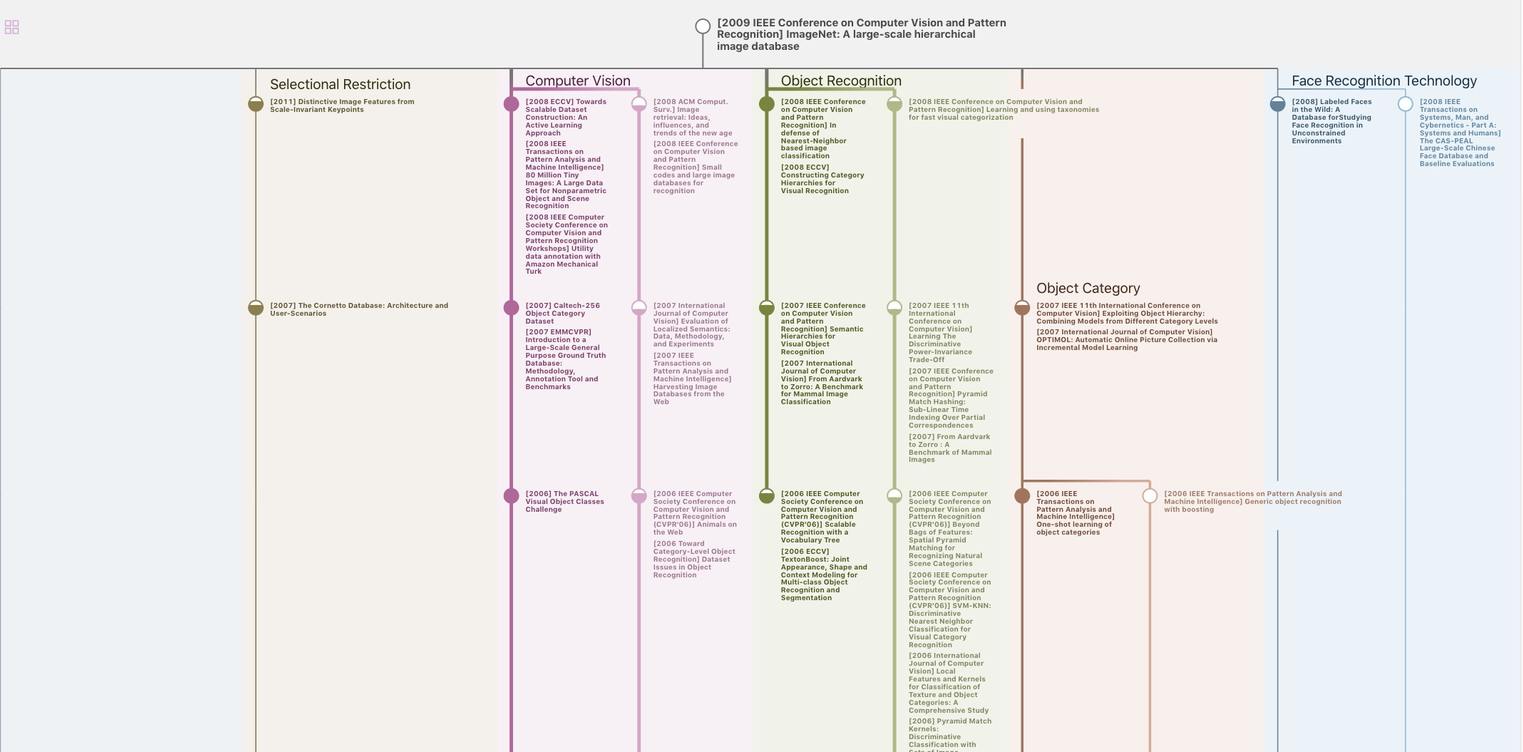
生成溯源树,研究论文发展脉络
Chat Paper
正在生成论文摘要