Deep adaptive registration of multi-modal prostate images.
Computerized Medical Imaging and Graphics(2020)
摘要
Artificial intelligence, especially the deep learning paradigm, has posed a considerable impact on cancer imaging and interpretation. For instance, fusing transrectal ultrasound (TRUS) and magnetic resonance (MR) images to guide prostate cancer biopsy can significantly improve the diagnosis. However, multi-modal image registration is still challenging, even with the latest deep learning technology, as it requires large amounts of labeled transformations for network training. This paper aims to address this problem from two angles: (i) a new method of generating large amount of transformations following a targeted distribution to improve the network training and (ii) a coarse-to-fine multi-stage method to gradually map the distribution from source to target. We evaluate both innovations based on a multi-modal prostate image registration task, where a T2-weighted MR volume and a reconstructed 3D ultrasound volume are to be aligned. Our results demonstrate that the use of data generation can significantly reduce the registration error by up to 62%. Moreover, the multi-stage coarse-to-fine registration technique results in a mean surface registration error (SRE) of 3.66 mm (with the initial mean SRE of 9.42 mm), which is found to be significantly better than the one-step registration with a mean SRE of 4.08 mm.
更多查看译文
关键词
Convolutional neural networks,Prostate cancer,Image registration,Multi-modal image fusion
AI 理解论文
溯源树
样例
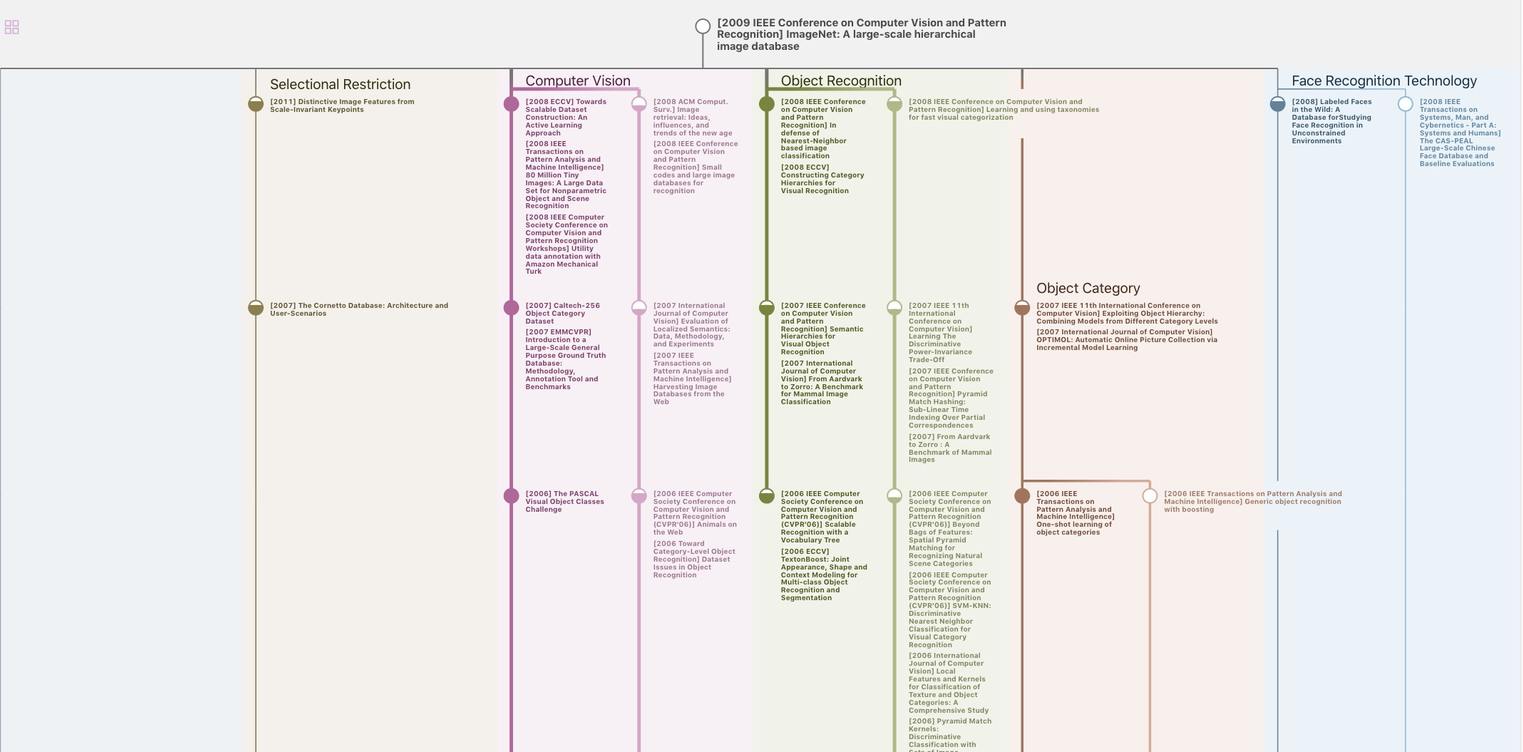
生成溯源树,研究论文发展脉络
Chat Paper
正在生成论文摘要