Adaptive CVaR Optimization for Dynamical Systems with Path Space Stochastic Search
arxiv(2020)
摘要
We present a general framework for optimizing the Conditional Value-at-Risk for dynamical systems using stochastic search. The framework is capable of handling the uncertainty from the initial condition, stochastic dynamics, and uncertain parameters in the model. The algorithm is compared against a risk-sensitive distributional reinforcement learning framework and demonstrates outperformance on a pendulum and cartpole with stochastic dynamics. We also showcase the applicability of the framework to robotics as an adaptive risk-sensitive controller by optimizing with respect to the fully nonlinear belief provided by a particle filter on a pendulum, cartpole, and quadcopter in simulation.
更多查看译文
关键词
path space stochastic search,dynamical systems,optimization
AI 理解论文
溯源树
样例
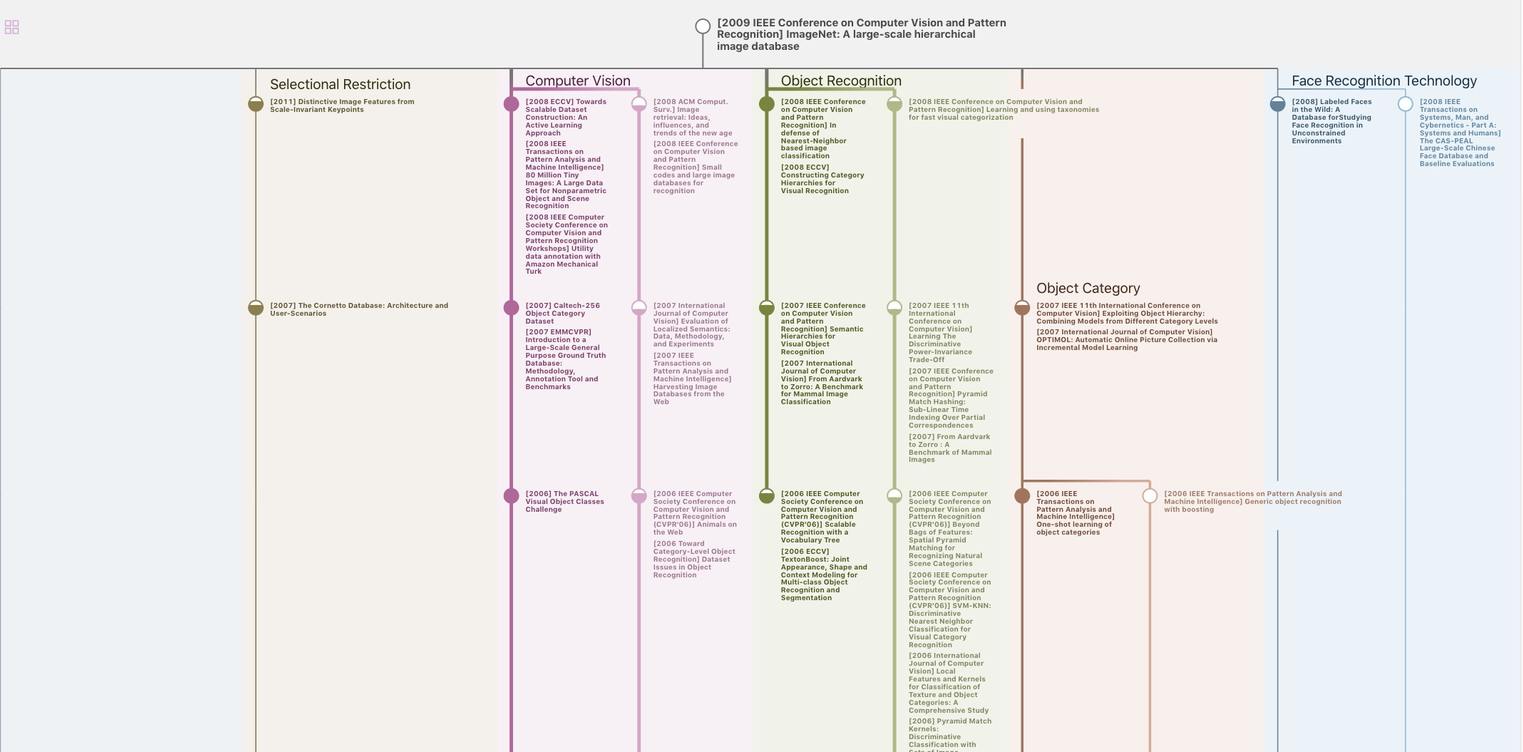
生成溯源树,研究论文发展脉络
Chat Paper
正在生成论文摘要