Hierarchical and Parameterized Learning of Pick-and-place Manipulation from Under-Specified Human Demonstrations
ADVANCED ROBOTICS(2020)
摘要
Imitating manipulation skills through observing human demonstrations in everyday life is promising in allowing service robots to be programed quickly, as well as to perform human-like behaviors. Such a Learning by demonstration (LbD) problem is challenging because robots are expected to adapt their learned behaviors to the changes of task parameters and the environment, rather than simply cloning the human teacher's motion. In this paper, we propose a hierarchical and parameterized LbD framework that combines symbolic and trajectory learning of pick-and-place manipulation tasks. We have extended the two-step parameterized learning method with error compensation for learning Environment-adaptive Action Primitives (EaAPs), which is capable of adapting robot's reproduced trajectories to new task instances as well as environmental changes. To arrive at refined plans in situations of under-specified human demonstrations, we propose to model the semantics of demonstrated activities with PDDL-based skill scripts. Therefore, latent motion primitives that are impossible to be learned directly from observing human demonstration in noisy video data can be inferred. The proposed method is implemented as a hierarchical LbD framework and has been evaluated on real robot hardware to illustrate the effectiveness of the proposed approach.
更多查看译文
关键词
Learning by demonstration,object manipulation,dynamic movement primitives,PDDL
AI 理解论文
溯源树
样例
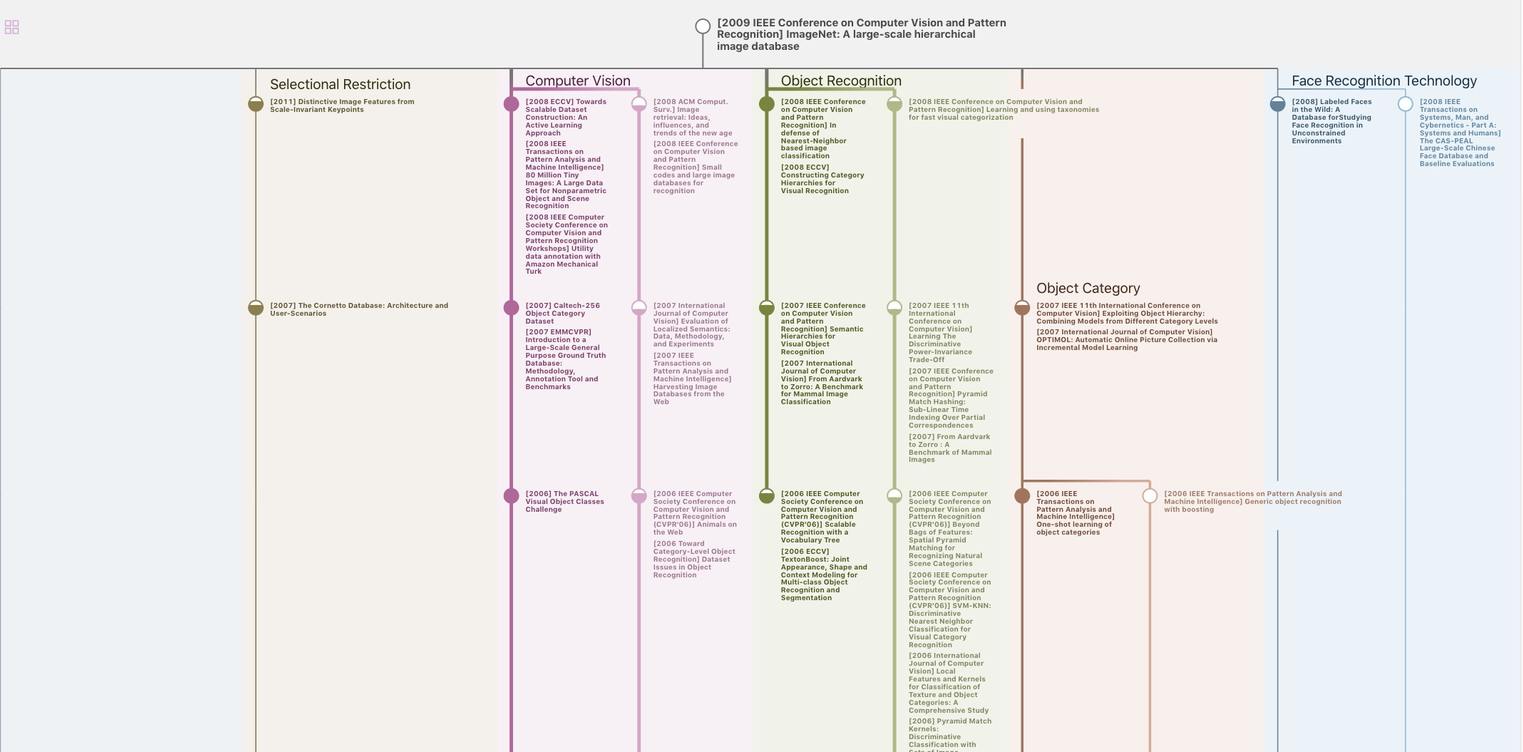
生成溯源树,研究论文发展脉络
Chat Paper
正在生成论文摘要