Counterfactual Prediction for Bundle Treatment
NIPS 2020(2020)
Abstract
Estimating counterfactual outcome of different treatments from observational data is an important problem to assist decision making in a variety of fields. Among the various forms of treatment specification, bundle treatment has been widely adopted in many scenarios, such as recommendation systems and online marketing. The bundle treatment usually can be abstracted as a high dimensional binary vector, which makes it more challenging for researchers to remove the confounding bias in observational data. In this work, we assume the existence of low dimensional latent structure underlying bundle treatment. Via the learned latent representations of treatments, we propose a novel variational sample re-weighting (VSR) method to eliminate confounding bias by decorrelating the treatments and confounders. Finally, we conduct extensive experiments to demonstrate that the predictive model trained on this re-weighted dataset can achieve more accurate counterfactual outcome prediction.
MoreTranslated text
Key words
Confounding Control,Bias Correction,Model Selection,Composite Endpoints,Covariance Estimation
AI Read Science
Must-Reading Tree
Example
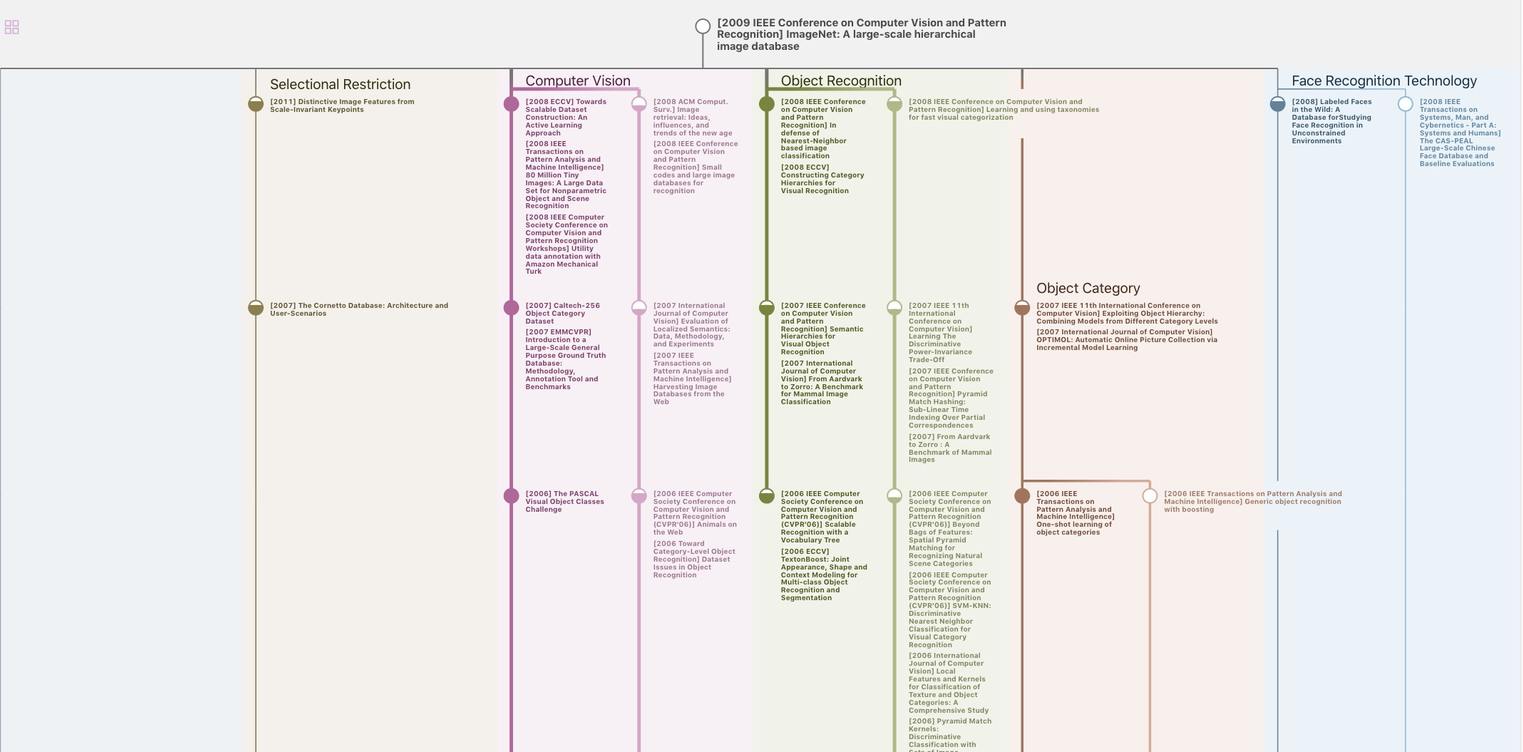
Generate MRT to find the research sequence of this paper
Chat Paper
Summary is being generated by the instructions you defined