The Emergence Of Explainability Of Intelligent Systems: Delivering Explainable And Personalized Recommendations For Energy Efficiency
INTERNATIONAL JOURNAL OF INTELLIGENT SYSTEMS(2021)
摘要
The recent advances in artificial intelligence namely in machine learning and deep learning, have boosted the performance of intelligent systems in several ways. This gave rise to human expectations, but also created the need for a deeper understanding of how intelligent systems think and decide. The concept of explainability appeared, in the extent of explaining the internal system mechanics in human terms. Recommendation systems are intelligent systems that support human decision making, and as such, they have to be explainable to increase user trust and improve the acceptance of recommendations. In this study, we focus on a context-aware recommendation system for energy efficiency and develop a mechanism for explainable and persuasive recommendations, which are personalized to user preferences and habits. The persuasive facts either emphasize on the economical saving prospects (Econ) or on a positive ecological impact (Eco) and explanations provide the reason for recommending an energy saving action. Based on a study conducted using a Telegram bot, different scenarios have been validated with actual data and human feedback. Current results show a total increase of 19% on the recommendation acceptance ratio when both economical and ecological persuasive facts are employed. This revolutionary approach on recommendation systems, demonstrates how intelligent recommendations can effectively encourage energy saving behavior.
更多查看译文
关键词
energy efficiency, explainable recommendation system, Internet of things, recommendation systems, rule-based recommendation, user habits
AI 理解论文
溯源树
样例
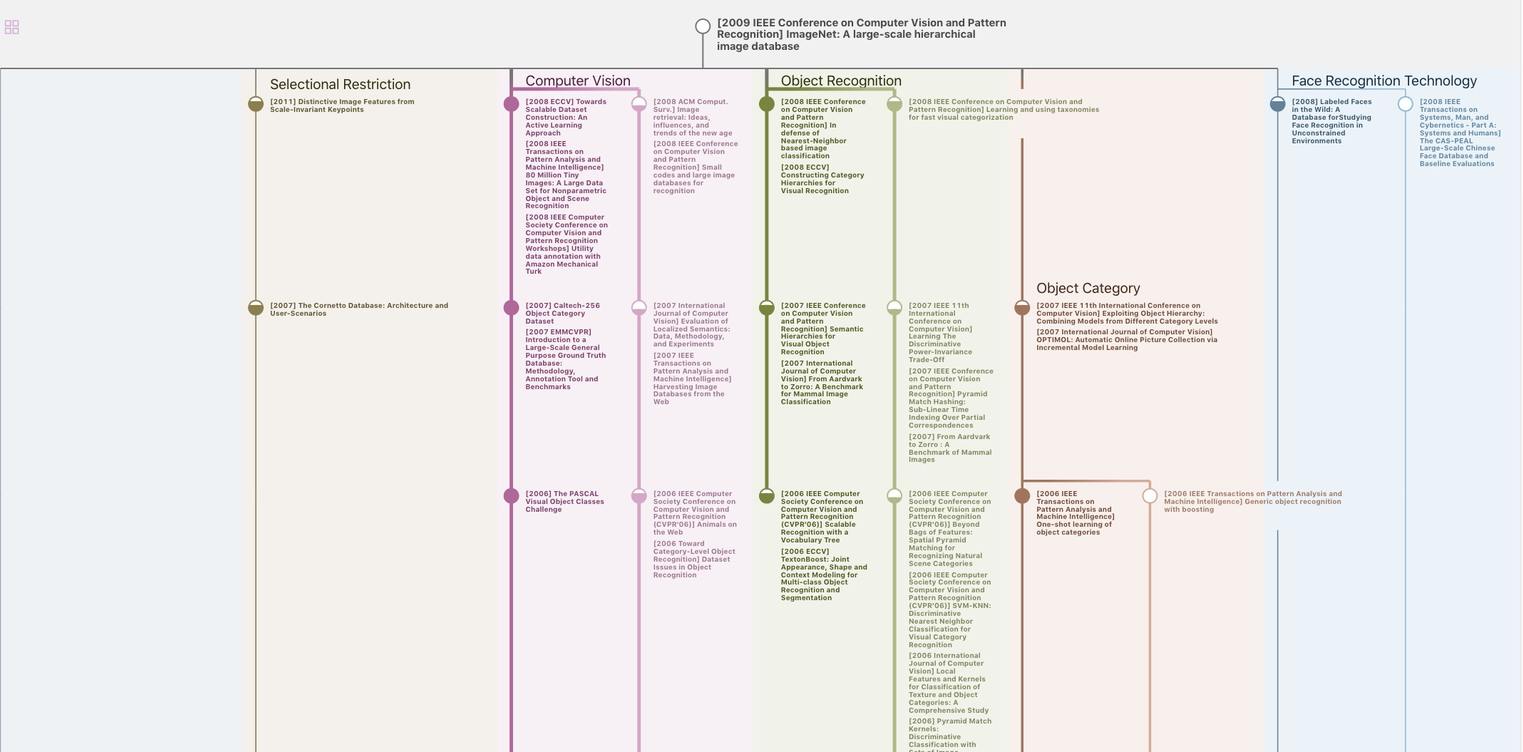
生成溯源树,研究论文发展脉络
Chat Paper
正在生成论文摘要