Accurate UAV Tracking with Distance-Injected Overlap Maximization
MM '20: The 28th ACM International Conference on Multimedia Seattle WA USA October, 2020(2020)
摘要
UAV tracking is usually challenged by the dual-dynamic disturbances that arise from not only diverse moving target but also motion camera, leading to a more serious model drift issue than traditional visual tracking. In this work, we propose to alleviate this issue with distance-injected overlap maximization. Our idea is improving the accuracy of target localization by deriving a conceptually simple target localization loss and a global feature recalibration scheme in a mutual reinforced way. In particular, the target localization loss is designed by simply incorporating the normalized distance of target offset and generic semantic IoU loss, resulting in the distance-injected semantic IoU loss, and its minimal solution can alleviate the drift problem caused by camera motion. Moreover, the deep feature extractor is reconstructed and alternated with a feature recalibration network, which can leverage the global information to recalibrate significant features and suppress negligible features. Following by multi-scale feature concat, the proposed tracker can improve the discriminative capability of feature representation for UAV targets on the fly. Extensive experimental results on four benchmarks, i.e. UAV123, UAVDT, DTB70, and VisDrone, demonstrate the superiority of the proposed tracker against existing state-of-the-arts on UAV tracking.
更多查看译文
关键词
UAV Tracking, feature recalibration, target refinement
AI 理解论文
溯源树
样例
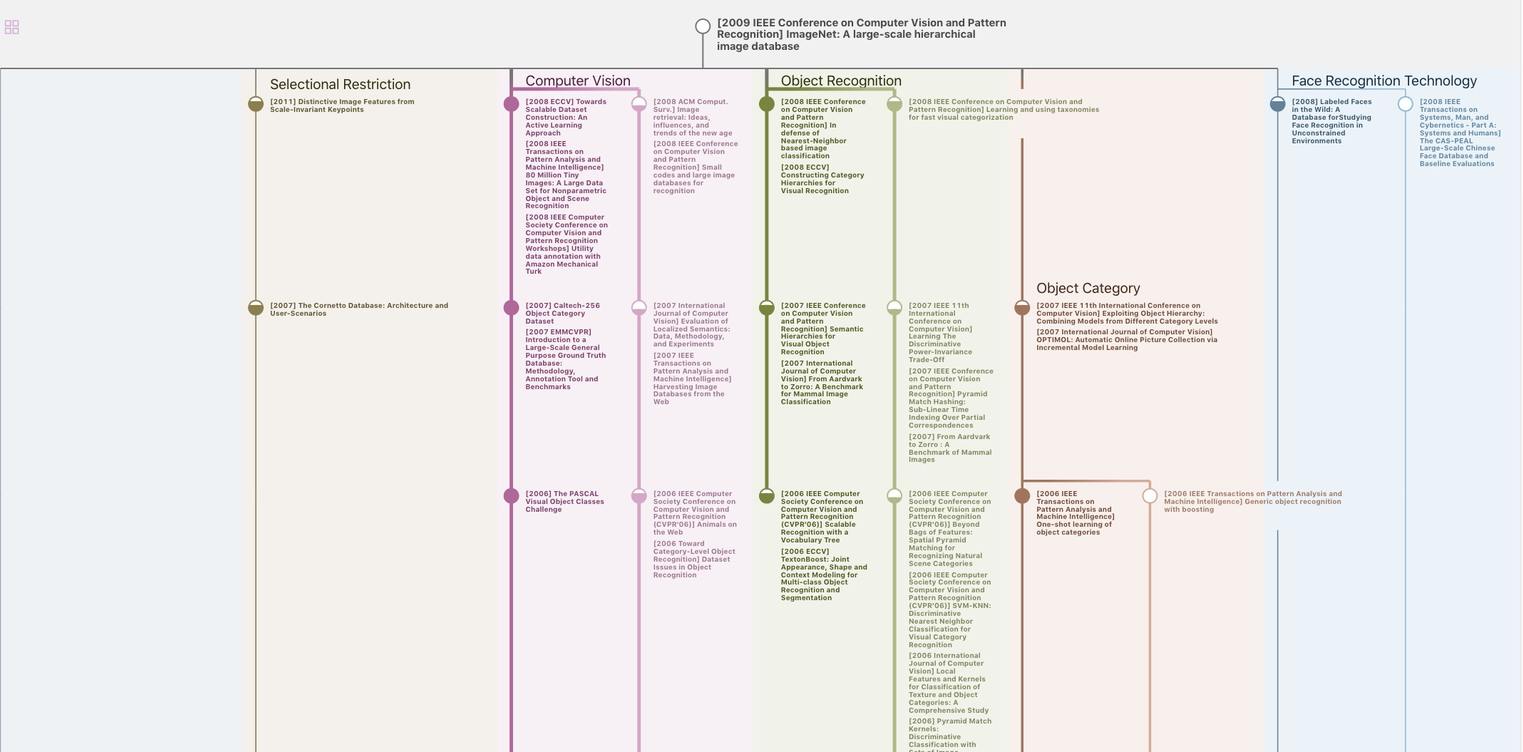
生成溯源树,研究论文发展脉络
Chat Paper
正在生成论文摘要