Learning Optimization-based Adversarial Perturbations for Attacking Sequential Recognition Models
MM '20: The 28th ACM International Conference on Multimedia Seattle WA USA October, 2020(2020)
摘要
A large number of recent studies on adversarial attack have verified that a Deep Neural Network (DNN) model designed for non-sequential recognition (NSR) tasks (e.g., classification, detection and segmentation) can be easily fooled by adversarial examples. However, only a few researches pay attention to the adversarial attack on sequential recognition (SR). They either apply the attack methods proposed for NSR to SR by neglecting the sequential dependencies, or focus on attacking specific SR models without considering the generality. In this paper, we study the adversarial attack on the general and popular DNN structure of CNN+RNN, i.e., the combination of convolutional neural network (CNN) and recurrent neural network (RNN), which has been widely used in various SR tasks. We take the scene text recognition (STR) and image captioning (IC) as case study, and derive the objective function for attacking the CNN+RNN based models with targeted and untargeted attack modes, and then developed an optimization-based algorithm to learn adversarial perturbations from the derived gradients of each character (or word) in sequence by incorporating the sequential dependencies. Extensive experiments show that our proposed method can effective fool several state-of-the-arts including four STR models and two IC models with higher successful rate and less time consumption, comparing to three latest attack methods.
更多查看译文
关键词
Sequential Recognition, Adversarial Attacks, Image Captioning, Scene Text Recognition
AI 理解论文
溯源树
样例
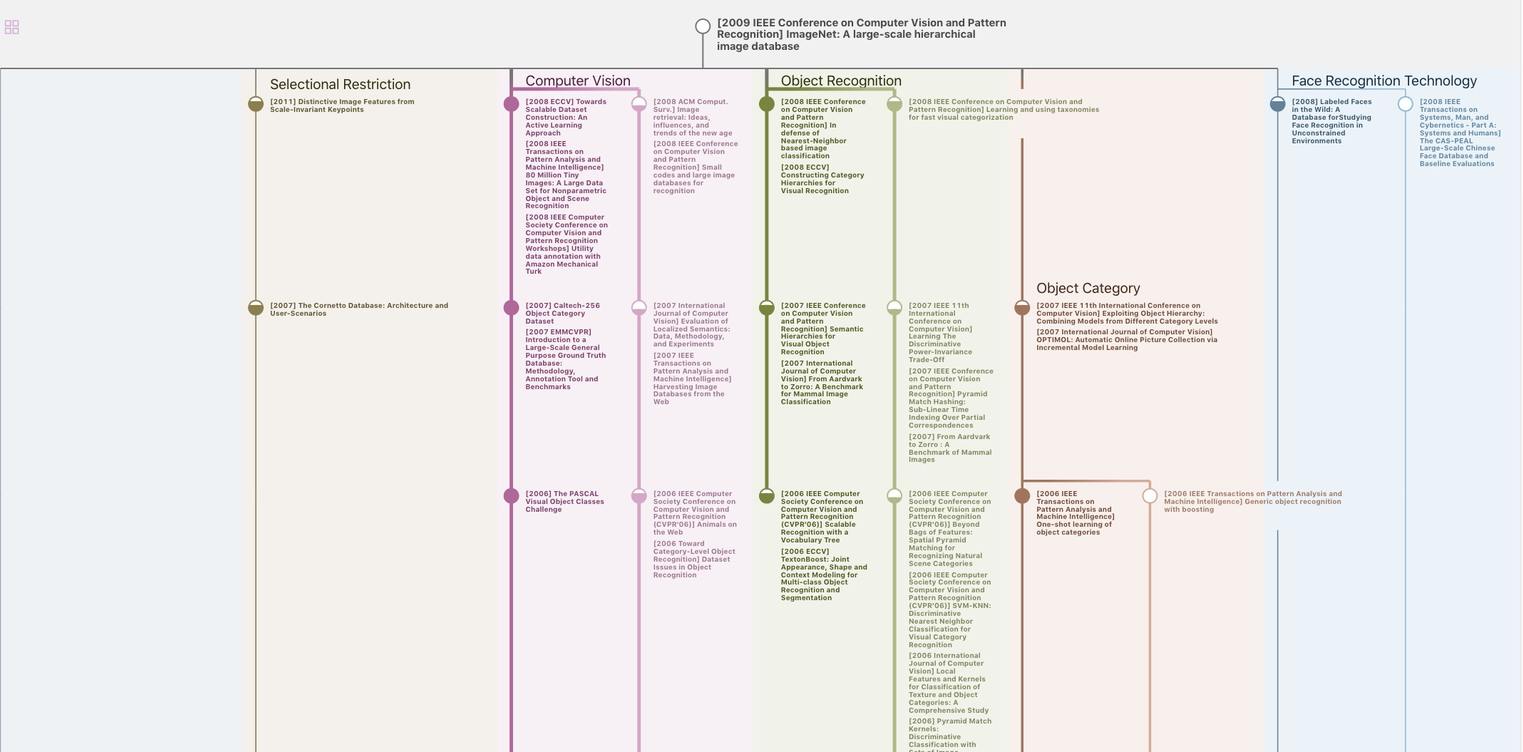
生成溯源树,研究论文发展脉络
Chat Paper
正在生成论文摘要