Predictive and generative machine learning models for photonic crystals
Nanophotonics(2020)
摘要
The prediction and design of photonic features have traditionally been guided by theory-driven computational methods, spanning a wide range of direct solvers and optimization techniques. Motivated by enormous advances in the field of machine learning, there has recently been a growing interest in developing complementary data-driven methods for photonics. Here, we demonstrate several predictive and generative data-driven approaches for the characterization and inverse design of photonic crystals. Concretely, we built a data set of 20,000 two-dimensional photonic crystal unit cells and their associated band structures, enabling the training of supervised learning models. Using these data set, we demonstrate a high-accuracy convolutional neural network for band structure prediction, with orders-of-magnitude speedup compared to conventional theory-driven solvers. Separately, we demonstrate an approach …
更多查看译文
关键词
generative models,inverse design,machine learning,neural networks,photonic crystals
AI 理解论文
溯源树
样例
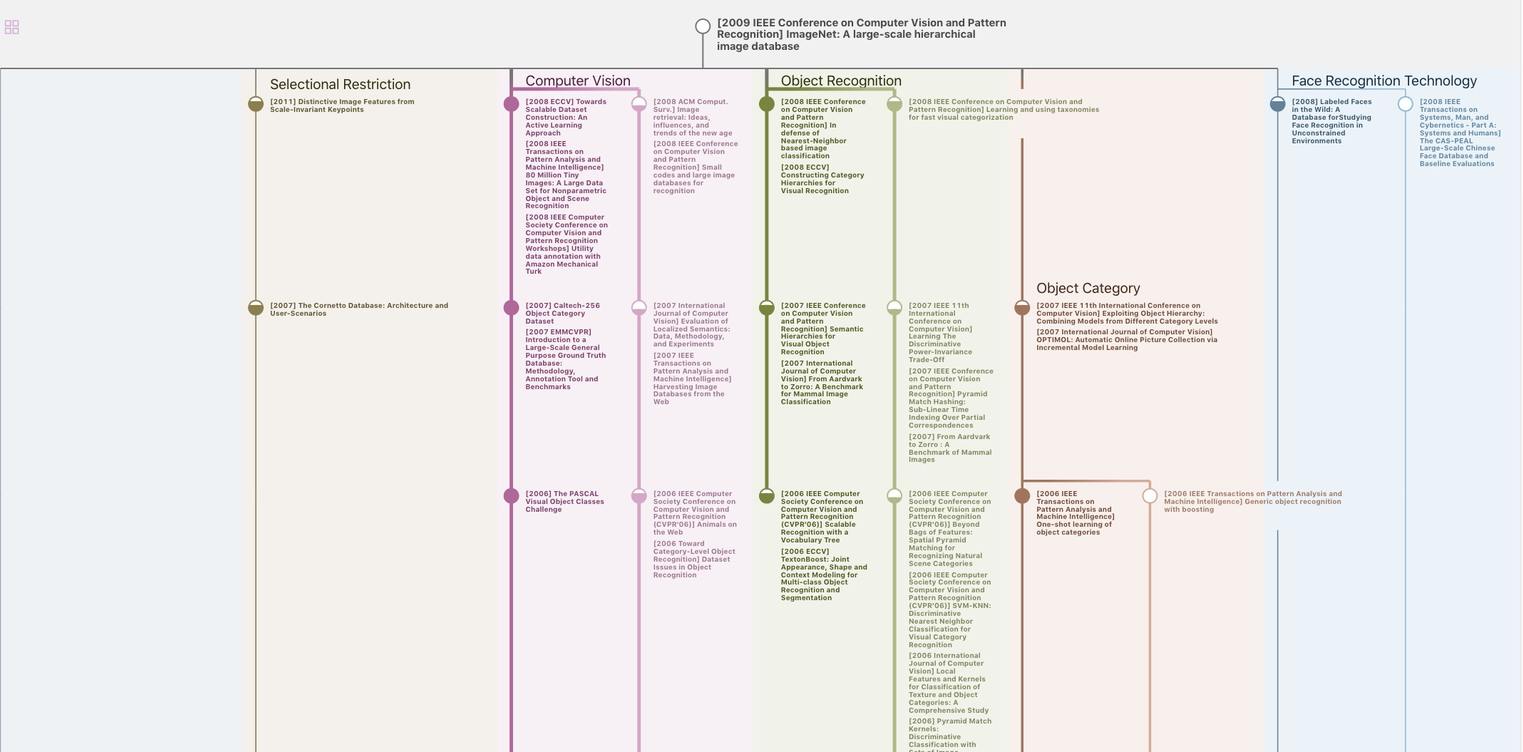
生成溯源树,研究论文发展脉络
Chat Paper
正在生成论文摘要