Behaviorally Diverse Traffic Simulation via Reinforcement Learning
2020 IEEE/RSJ International Conference on Intelligent Robots and Systems (IROS)(2020)
摘要
Traffic simulators are important tools in autonomous driving development. While continuous progress has been made to provide developers more options for modeling various traffic participants, tuning these models to increase their behavioral diversity while maintaining quality is often very challenging. This paper introduces an easily-tunable policy generation algorithm for autonomous driving agents. The proposed algorithm balances diversity and driving skills by leveraging the representation and exploration abilities of deep reinforcement learning via a distinct policy set selector. Moreover, we present an algorithm utilizing intrinsic rewards to widen behavioral differences in the training. To provide quantitative assessments, we develop two trajectory-based evaluation metrics which measure the differences among policies and behavioral coverage. We experimentally show the effectiveness of our methods on several challenging intersection scenes.
更多查看译文
关键词
traffic simulation,autonomous driving development,traffic participants,behavioral diversity,easily-tunable policy generation algorithm,autonomous driving agents,driving skills,exploration abilities,deep reinforcement learning,distinct policy set selector,intrinsic rewards,behavioral differences,behavioral coverage,trajectory-based evaluation metrics,intersection scenes
AI 理解论文
溯源树
样例
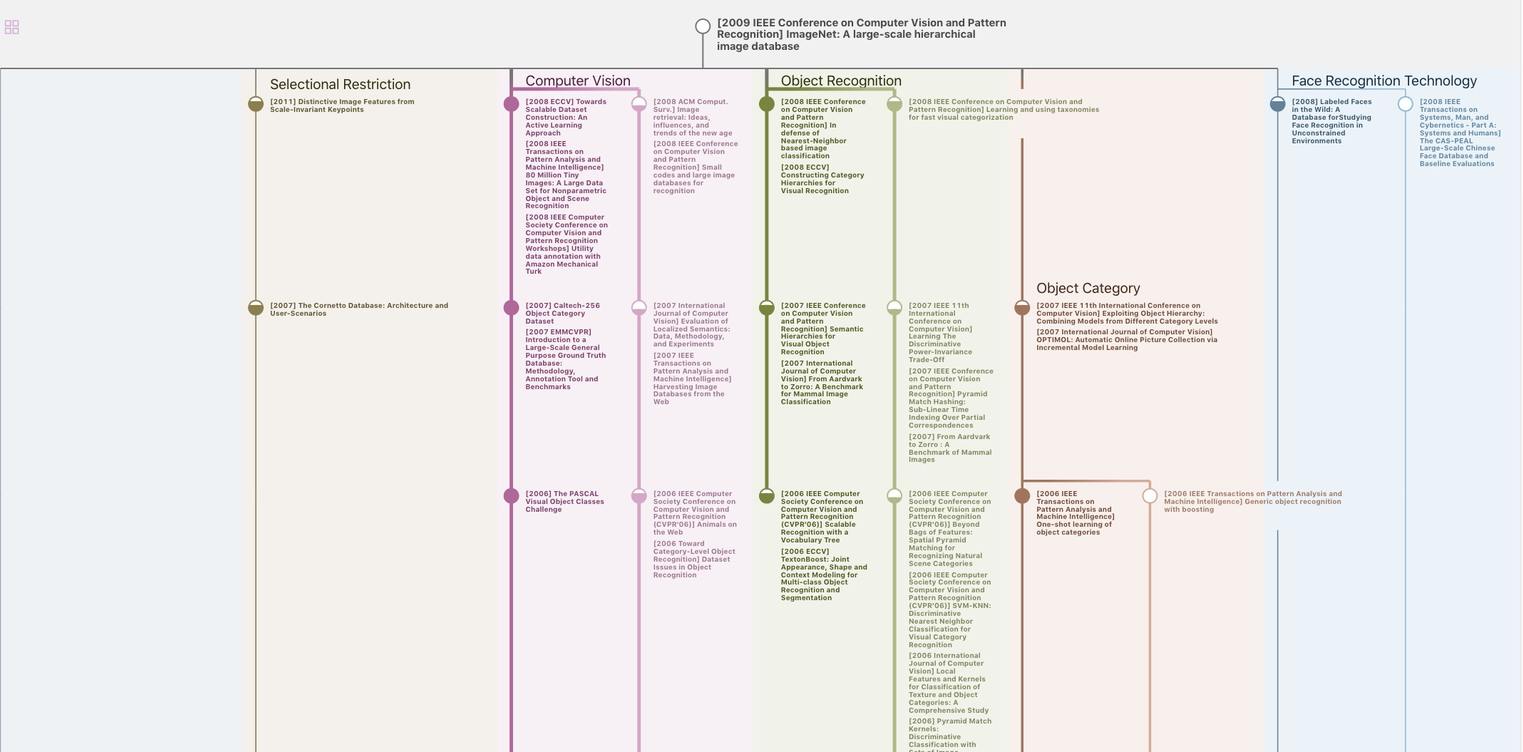
生成溯源树,研究论文发展脉络
Chat Paper
正在生成论文摘要