NDNet: Narrow While Deep Network for Real-Time Semantic Segmentation
IEEE Transactions on Intelligent Transportation Systems(2021)
摘要
The rapid development of autonomous driving in recent years presents many challenges for scene understanding. As an essential step towards scene understanding, semantic segmentation has received increased attention in the past few years. Although deep learning based approaches have achieved great success in improving the segmentation accuracy, most of them suffer from an inefficiency problem and can hardly be applied to real-time applications. In this paper, we analyze the computational cost of Convolutional Neural Network (CNN) and find that the inefficiency of CNNs is mainly caused by their wide structure rather than deep structure. In addition, the success of pruning based model compression methods proves that there are many redundant channels in CNNs. Thus, we design a narrow while deep backbone network to improve the efficiency of semantic segmentation. By casting our network to fully convolutional network (FCN32) segmentation architecture, the basic structure of most segmentation methods, we achieve 61.5% mIoU on Cityscapes validation dataset with only 4.2G floating-point operations (FLOPs) on 1024×2048 inputs, which already outperforms one of the earliest real-time deep learning based segmentation methods: ENet (58.3% mIoU, 3.8G FLOPs on 640×360 inputs). By further refining the output resolution of our network to the 1/8 of the input resolution with a simple encoder-decoder structure, we achieve 65.3% mIoU on Cityscapes test set with 14.0G FLOPs and 39.9 frames per second (FPS) on Titan X card. We have made our model publicly available at https://github.com/zgyang-hnu/NDNet.
更多查看译文
关键词
Semantic segmentation,autonomous driving,real-time,deep learning
AI 理解论文
溯源树
样例
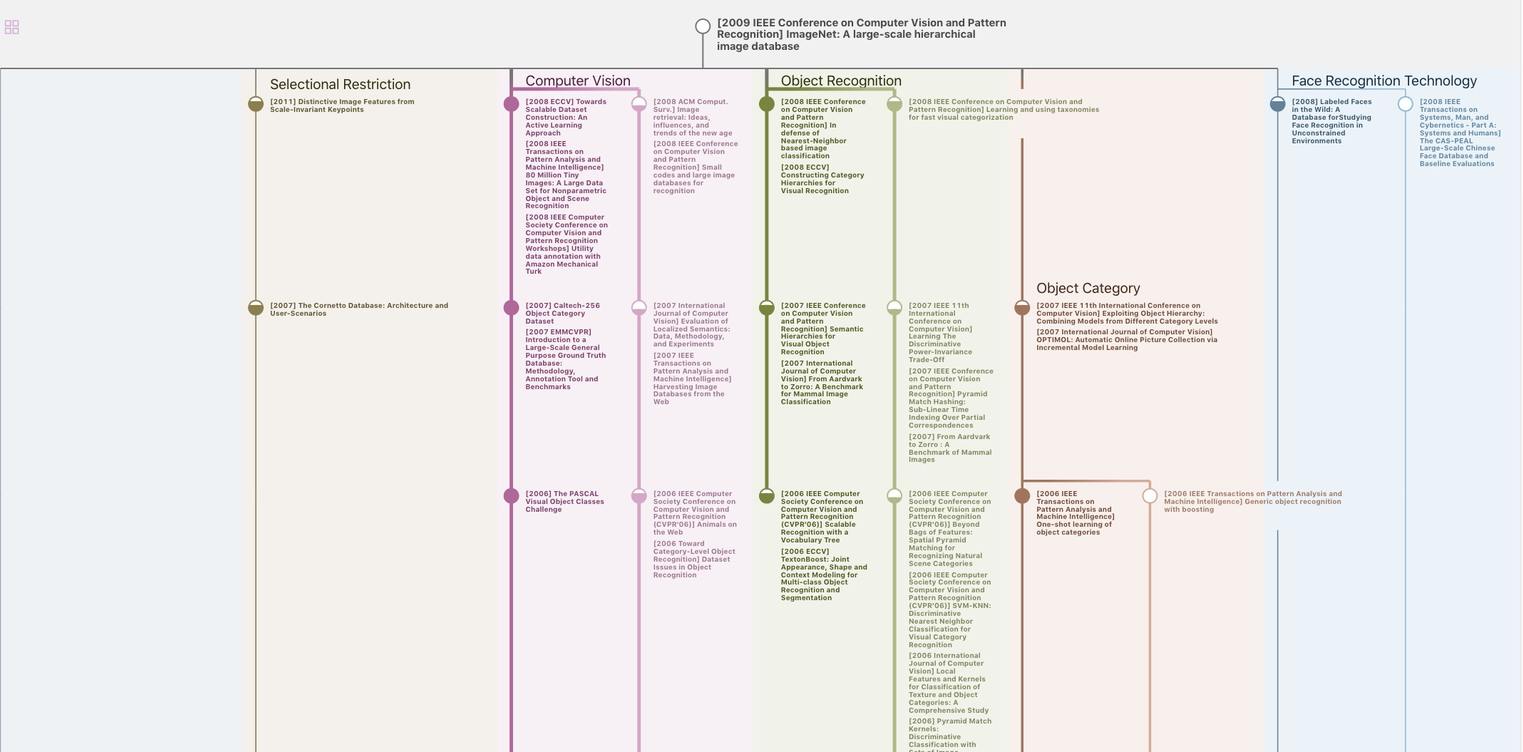
生成溯源树,研究论文发展脉络
Chat Paper
正在生成论文摘要