Grasp Pose Detection with Affordance-based Task Constraint Learning in Single-view Point Clouds
JOURNAL OF INTELLIGENT & ROBOTIC SYSTEMS(2020)
摘要
Learning to grasp novel objects is a challenging issue for service robots, especially when the robot is performing goal-oriented manipulation or interaction tasks whilst only single-view RGB-D sensor data is available. While some visual approaches focus on grasping that satisfy force-closure standards only, we further link affordances-based task constraints to the grasp pose on object parts, so that both force-closure standard and task constraints can be ensured. In this paper, a new single-view approach is proposed for task-constrained grasp pose detection. We propose to learn a pixel-level affordance detector based on a convolutional neural network. The affordance detector provides a fine grained understanding of the task constraints on objects, which are formulated as a pre-segmentation stage in the grasp pose detection framework. The accuracy and robustness of grasp pose detection are improved by a novel method for calculating local reference frame as well as a position-sensitive fully convolutional neural network for grasp stability classification. Experiments on benchmark datasets have shown that our method outperforms the state-of-the-art methods. We have also validated our method in real-world and task-specific grasping scenes, in which higher success rate for task-oriented grasping is achieved.
更多查看译文
关键词
Robot grasp,Grasp pose detection,Object affordance,Convolutional neural networks,Constraints learning
AI 理解论文
溯源树
样例
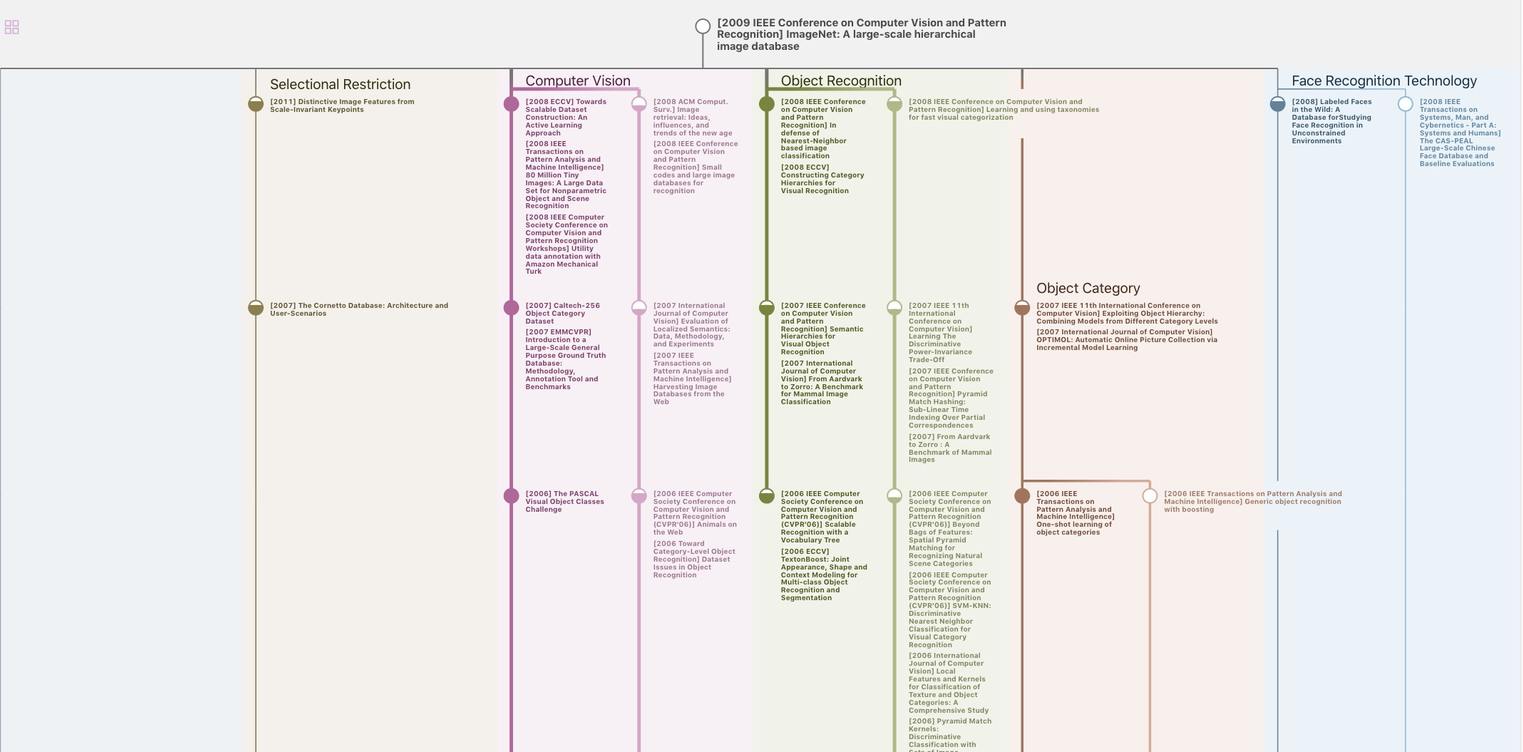
生成溯源树,研究论文发展脉络
Chat Paper
正在生成论文摘要