Discovering Avoidable Planner Failures of Autonomous Vehicles using Counterfactual Analysis in Behaviorally Diverse Simulation
ITSC(2020)
摘要
Automated Vehicles require exhaustive testing in simulation to detect as many safety-critical failures as possible before deployment on public roads. In this work, we focus on the core decision-making component of autonomous robots: their planning algorithm. We introduce a planner testing framework that leverages recent progress in simulating behaviorally diverse traffic participants. Using large scale search, we generate, detect, and characterize dynamic scenarios leading to collisions. In particular, we propose methods to distinguish between unavoidable and avoidable accidents, focusing especially on automatically finding planner-specific defects that must be corrected before deployment. Through experiments in complex multi-agent intersection scenarios, we show that our method can indeed find a wide range of critical planner failures.
更多查看译文
关键词
avoidable planner failures,counterfactual analysis,autonomous vehicles,simulation
AI 理解论文
溯源树
样例
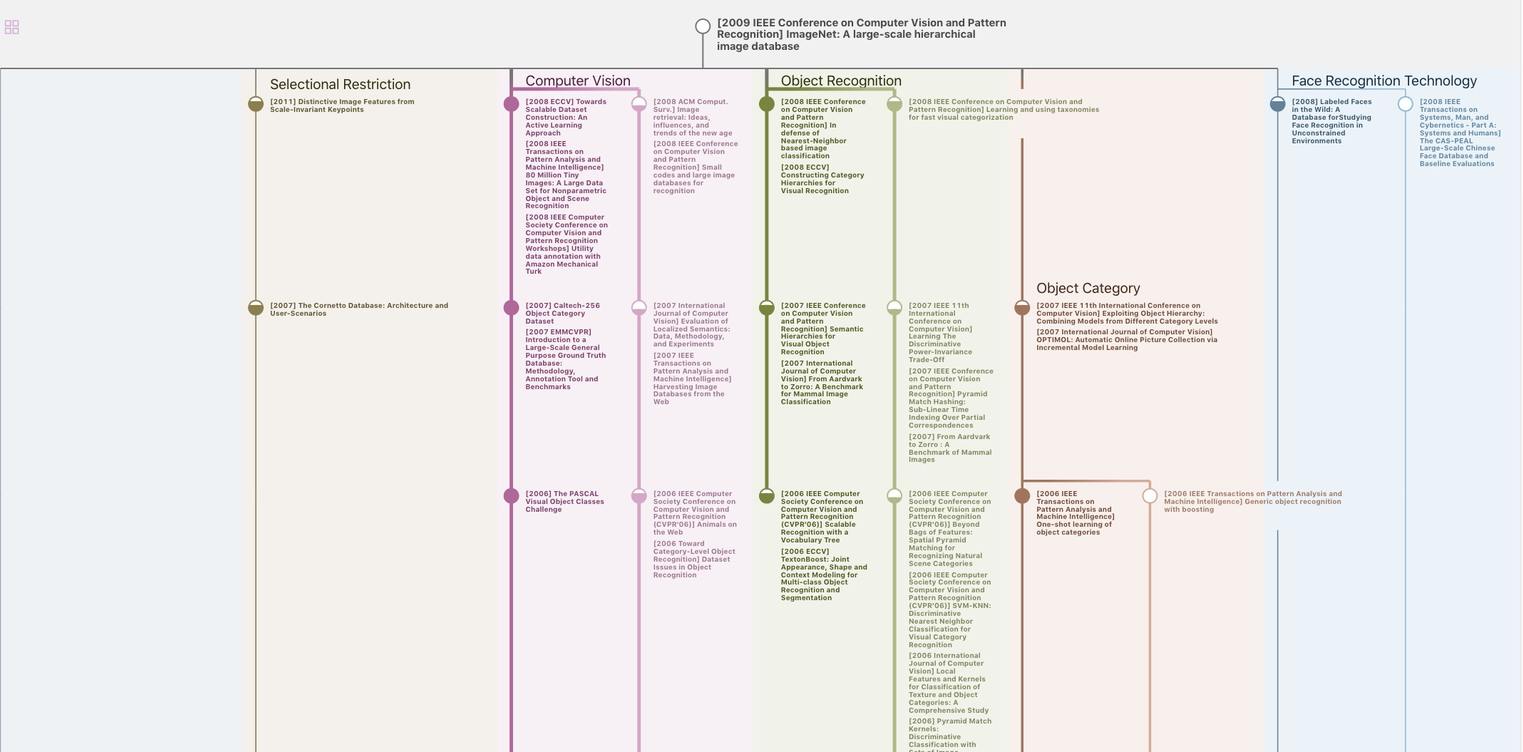
生成溯源树,研究论文发展脉络
Chat Paper
正在生成论文摘要